Statistical Mechanics & Applications to Finance
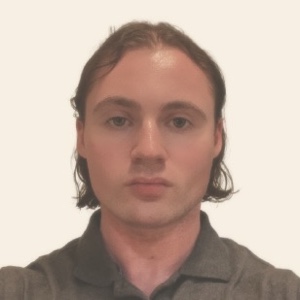
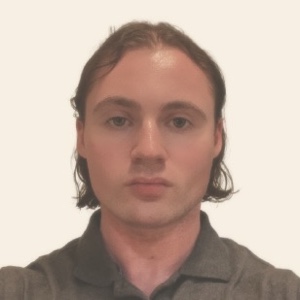
Statistical Mechanics, also known as Statistical Physics, looks into the microscopic details of systems to predict macroscopic behaviors.
Beyond traditional physics, Statistical Mechanics finds applications in finance, modeling complex systems from particles to assets and broader physical systems to portfolios.
Key Takeaways – Statistical Mechanics & Applications to Finance
- Statistical mechanics can provide new analytical perspectives and mathematical frameworks in finance.
- It can model market behaviors by treating entities in a financial system like particles in a thermodynamic system.
- Concepts like entropy and ensemble averaging provide different ways of looking at market unpredictability, risk, and long-term equilibrium.
- Models from physics like Ising, Potts, and percolation can be adapted to study market dynamics like sentiment spreading, systemic risk, and asset correlations.
- Mathematical approaches like stochastic processes and mean field theory also find direct analogues and applications in finance.
- The physics of phase transitions and critical phenomena can provide perspective on financial paradigm shifts, liquidity crises, and system interconnectivity.
- Entropy concepts from statistical mechanics offer a framework to evaluate market volatility and the distribution of returns, assisting in risk management and portfolio optimization.
Statistical Mechanics Theory
Principle of Maximum Entropy
The Principle of Maximum Entropy is a foundational concept in statistical mechanics.
It posits that, given certain constraints, a system will evolve to a state of maximum entropy, representing the most probable macrostate.
In finance, this principle can be likened to the Efficient Market Hypothesis (EMH).
Just as systems tend towards maximum entropy, financial markets, according to EMH, adjust rapidly to new information, ensuring that securities are appropriately priced.
The idea is that markets, like physical systems, gravitate towards a state of equilibrium or “maximum informational entropy,” where no arbitrage opportunities exist.
Applications to Finance
- Risk Management: By understanding the most probable states of a financial system, traders/investors can better assess and manage risk. The principle can be used to model the distribution of returns, helping in the optimization of portfolios.
- Asset Pricing: In an efficient market, asset prices reflect (discount) all available information. The principle of maximum entropy can be used to derive models that predict asset prices based on the assumption that the market is in its most probable state.
Ergodic Theory
Ergodic Theory, in the context of statistical mechanics, studies the long-term behavior of dynamical systems.
It asserts that, over a long time, the time average of a system property will be equal to its ensemble average. (An ensemble means a collective behavior of multiple interacting components.)
Physics Example
In a gas-filled container, even if individual gas molecules move chaotically, over time, the average energy per molecule (time average) will match the average energy across all molecules (ensemble average), leading to a consistent temperature throughout the container.
Translated to finance, over time, the average risk-adjusted return of a single asset (time average) will align with the average risk-adjusted return of a diversified portfolio (ensemble average), and reducing the influence of individual asset volatility.
Applications to Finance
- Trading Strategies: Traders can use the ergodic nature of financial markets to develop strategies that exploit long-term predictable behaviors. For instance, if a particular stock exhibits ergodic behavior, its future price movements can be predicted by analyzing its historical data. If a stock has shown low volatility and consistent returns in the past (e.g., a utility stock), that behavior is more likely to continue.
- Investment Analysis: Investors can assess the long-term performance of an investment by studying the short-term performance of a broad ensemble of similar investments. This can aid in making informed decisions about asset allocation and diversification.
- Financial Forecasting: Ergodic Theory can be instrumental in financial forecasting. By assuming that financial markets are ergodic, analysts can make predictions about long-term market behavior based on short-term data.
Statistical Thermodynamics in Finance: Bridging Physics and Financial Markets
Ensembles
In statistical mechanics, an ensemble is a large collection of microstates representing possible configurations of a system.
In finance, an ensemble can be thought of as a collection of various assets, which interact to give the broader performance of a portfolio.
By analyzing these ensembles, one can gauge the probabilities of various market outcomes.
Applications to Finance
- Portfolio Diversification: Just as an ensemble represents various system configurations, a diversified portfolio represents a collection of assets that can weather different market scenarios.
- Monte Carlo Simulations: This method, used extensively in finance, generates a vast number of possible price paths (ensembles) to calculate option prices, Value at Risk (VaR), and other financial metrics.
Partition Functions
The partition function in thermodynamics sums over all possible states of a system, weighted by their probability.
In finance, the partition function can represent the sum of all possible financial outcomes, weighted by their likelihood.
Applications to Finance
- Option Pricing: The partition function can be used to model the sum of all possible payoffs of an option, leading to more accurate pricing models.
- Risk Assessment: By considering all possible financial scenarios and their probabilities, one can better assess and hedge against risks.
Equations of State
Equations of state relate macroscopic properties of a system, like pressure and volume, to its temperature and number of particles.
In finance, equations of state can be analogous to models relating macroeconomic indicators to more specific market behaviors (e.g., how consumer staples might outperform consumer discretionary in a worsening economy).
Applications to Finance
- Macroeconomic Analysis: Equations can be developed to relate economic indicators like inflation, GDP, and current/capital account to stock market performance or interest rates.
Thermodynamic Potential
In thermodynamics, potentials like U, H, F, and G describe the energy of a system under different conditions.
For finance, these can be likened to various measures of portfolio performance under different market conditions.
Applications to Finance
- Portfolio Optimization: By understanding the performance of a portfolio under various scenarios like falling/rising inflation, falling/rising growth, changing discount rates, and changing risk premiums (akin to different thermodynamic conditions), traders and investors can optimize their portfolios to maximize returns and minimize risks.
Maxwell Relations
Maxwell relations are mathematical relationships between different partial derivatives of thermodynamic potentials.
In finance, similar relationships can be derived between various financial metrics.
For example, the Greeks (Delta, Gamma, Vega, Theta) describe how option prices change with underlying asset price, volatility, and time.
Like Maxwell relations, they provide relationships between different derivatives of an option’s value, aiding in risk management and hedging strategies.
Applications to Finance
- Arbitrage Opportunities: By understanding the relationships between different financial metrics, traders can identify and exploit price discrepancies in the market.
- Financial Modeling: Maxwell-like relations can be used to develop models that capture the cause-effect interplay between various financial indicators, leading to more accurate forecasts.
Models in Physics and Their Financial Analogues
Ferromagnetism Models
Ferromagnetism models describe the behavior of magnetic materials.
These models can be applied metaphorically to financial markets, where “spins” can represent the bullish or bearish sentiment of traders. (This analog is already applied in the study of econophysics.)
Ising Model
This model considers spins that can be either up or down.
In finance, this can represent binary outcomes, such as stock prices going up or down.
The Ising model can be used to understand sociophysics concepts, like herd behavior in markets, where the sentiment of one trader can influence another.
Applications to Finance:
- Market Sentiment Analysis: By modeling traders as spins, one can predict how bullish or bearish sentiment might spread through the market. If demand exceeds supply, prices will rise, and vice versa.
- Binary Options Pricing: The binary nature of the Ising model can be applied to price binary options.
Potts Model
The Potts Model is a generalization of the Ising model in statistical mechanics.
It describes interacting spins on a lattice that can take on q distinct values, rather than just two.
It’s used to study phase transitions and magnetism in complex systems.
In financial contexts, this can represent multiple market scenarios (“states of the world”) or strategies.
Applications to Finance:
- Multi-Scenario Analysis: For assessing the potential outcomes of complex financial instruments or strategies.
- Diversification Strategies: Understanding how different assets might behave under various market conditions.
Heisenberg Model
The Heisenberg Model describes interacting spins on a lattice, where each spin can point in any direction in three-dimensional space.
It’s fundamental in quantum mechanics for studying magnetic properties and phase transitions in materials.
This can represent the continuous range of returns in financial markets.
Applications to Finance:
- Portfolio Optimization: For understanding the range of possible returns and risks of a portfolio.
- Derivative Pricing: Modeling the continuous range of potential payoffs.
Percolation Theory
Percolation Theory studies the behavior of connected clusters in a random graph.
It explores the transition point where a system changes from being mostly disconnected to having a large connected component.
It’s often used to understand porous materials, disease spread, and network robustness.
In finance, it can represent the interconnectedness of financial institutions or assets.
Applications to Finance:
- Systemic Risk Analysis: Understanding how the failure of one institution might affect others.
- Correlation Studies: Assessing how different assets might be interconnected based on fundamental characteristics, players in the market, and so on.
Particles with Force Field
Particles with force fields describe interactions between particles based on distance.
These can be likened to the interactions between financial entities or assets.
Depletion Force
This force arises due to the exclusion of particles from a certain volume.
In finance, this can represent the exclusion of certain assets or strategies from a market or portfolio.
Applications to Finance:
- Market Entry/Exit Strategies: Understanding barriers to entry or exit in certain markets.
- Portfolio Construction: Excluding certain assets based on risk or other criteria.
Lennard-Jones Potential
This potential describes the attraction and repulsion between particles.
In finance, it can represent the push and pull forces in markets, such as supply and demand.
Applications to Finance:
- Market Dynamics: Modeling the forces of supply and demand and their impact on prices.
- Asset Correlation: Understanding how different assets might move together (positively correlate) or repel (negatively correlate) each other.
Mathematical Approaches in Physics and Their Financial Analogues
Boltzmann Equation
The Boltzmann equation describes the statistical behavior of a thermodynamic system not in equilibrium.
In finance, it can be used to model the evolution of a market or asset distribution over time, especially when external forces or shocks are applied.
Applications to Finance
- Market Dynamics: Understanding how markets evolve in response to external events, such as geopolitical incidents or regulatory changes.
- Asset Allocation: Modeling how the distribution of assets in a portfolio changes over time. (For example, how would an investor change their asset allocation or the volatility they run their portfolio at as they get nearer to retirement?)
H-Theorem
The H-theorem, derived from the Boltzmann equation, states that the entropy of an isolated system will increase over time, reaching a maximum at equilibrium.
In finance, this can represent the idea that markets move towards an equilibrium state.
Applications to Finance
- Risk Management: Predicting how disturbances in the market will eventually settle, aiding in long-term risk assessments.
- Market Efficiency: Understanding how markets self-correct and move towards equilibrium.
Vlasov Equation
The Vlasov equation models the evolution of plasma distribution in phase space, considering collective effects without collisions.
It describes how charged particles in a plasma interact with electromagnetic fields.
This is important for understanding many plasma phenomena and astrophysical contexts.
In finance, it can model the behavior of individual assets in a market without considering pairwise interactions.
Applications to Finance
- Market Segmentation: Understanding how individual market segments behave independently of others, at least under certain conditions.
- Trends/Shocks: It could help in understanding how large-scale market trends or shocks affect the distribution and behavior of individual assets or market participants over time.
BBGKY Hierarchy
The BBGKY hierarchy describes the evolution of multi-particle distribution functions in statistical mechanics.
It links the dynamics of a single particle to interactions with others.
In finance, it can represent the interdependencies and correlations between multiple assets or financial instruments.
How do individual asset behaviors relate to multi-asset interactions?
Applications to Finance
- Correlation Analysis: Studying how groups of assets move in relation to each other.
- Multi-Asset Derivative Pricing: Pricing complex financial instruments that depend on multiple underlying assets.
Stochastic Process
A stochastic process is a collection of random variables representing the evolution of a system over time.
It is very popular in probability theory and stochastic processes is its own field of mathematics.
It’s directly applicable to finance, especially in modeling asset prices, interest rates, and other financial metrics.
Applications to Finance
- Option Pricing: Using models like the Black-Scholes, which is based on stochastic processes.
- Risk Assessment: Evaluating the potential future paths of assets or portfolios.
Mean-Field Theory
This theory approximates the effects of all particles in a system on a single particle.
In finance, it can model the effect of the entire market or a large group of assets on a single asset or a small group of assets.
Applications to Finance
- Market Impact Analysis: Assessing how major market moves might affect individual stocks or sectors. For example, how do the big four forces determining price changes at the broad asset class level (i.e., changes in discounted growth, changes in discounted inflation, changes in discount rates, changes in risk premiums) influence individual asset prices?
- Portfolio Diversification: Understanding how individual assets might behave in the context of a larger portfolio.
Conformal Field Theory
Conformal Field Theory (CFT) is a quantum field theory that remains invariant under conformal transformations, often used in high-energy physics and string theory. It’s a quantum field theory that respects conformal symmetry.
While its direct application to finance is less intuitive, the underlying idea of systems behaving uniformly under transformations can be applied metaphorically, if not in formal mathematical terms in various contexts.
CFT could represent a framework where market behaviors remain consistent under certain scaling or transformation conditions, highlighting universal patterns or symmetries in market dynamics.
In plain English, CFT is like a rulebook for particles that doesn’t change even if you stretch or squeeze the space they’re in.
Imagine a financial system where certain patterns of behavior stay the same, no matter how the market scales or shifts.
That’s the essence of CFT applied to finance.
For example, no matter the size of the market (assuming no liquidity issues), changes in discounted growth, discounted inflation, discount rates, and risk premiums should impact broad asset classes by the same percentage.
Applications to Finance
- Scale Invariance: Studying how financial patterns or behaviors might repeat at different scales (e.g., no matter how large or small a market is), from individual trades to large market movements.
- Fractal Analysis: Analyzing financial data at various scales to identify recurring patterns.
Critical Phenomena in Physics and Their Financial Analogues
Phase Transition
In physics, a phase transition refers to the transformation of a system from one state or phase to another, like water turning into ice or steam.
In financial contexts, phase transitions can be thought of as sudden, systemic shifts in market dynamics or regimes.
Applications to Finance
- Market Regime Shifts: Recognizing when markets transition from, say, a world where short-term interest rate changes are the biggest lever on liquidity (pre-2008) -> a world where quantitative easing is the big driver (2009-2020) -> a world where monetary and fiscal policy are intertwined (post-2020). Understanding these paradigm shifts can be important for asset allocation and risk management.
- Economic Cycles: Identifying transitions between economic expansions and recessions, which can have implications for investment strategies, particularly tactical asset allocation decisions.
- Liquidity Crises: Understanding how seemingly liquid markets can suddenly freeze when there’s not enough money and credit to buy assets (e.g., corporate credit in October 2008, many markets in March 2020), leading to rapid price declines.
Critical Exponents
Critical exponents, such as correlation length and size scaling, describe how physical quantities change as a system approaches a critical point or phase transition.
Correlation Length
In physics, this refers to the scale over which fluctuations in a system are correlated.
The distance over which properties of a system remain interrelated, indicating the extent of cooperative behavior before randomness/non-correlation takes over.
In finance, it can represent the extent to which price movements in one asset or market segment are related to those in another.
Applications to Finance:
- Portfolio Diversification: If assets are highly correlated over long “lengths” or durations, diversifying across them might not reduce risk as effectively.
- Hedge Effectiveness: Assessing how well a hedge will work, given the correlation length between the hedged asset and the hedging instrument.
Size Scaling
This describes how certain properties of a system scale with its size.
As an example in physics, as a ferromagnetic material’s size increases, the number of aligned spins (magnetization) scales proportionally.
In a phase transition, the behavior of small samples can differ significantly from bulk material due to boundary effects, illustrating size-dependent scaling of magnetic properties.
In financial terms, it can relate to how market behaviors or risks scale as the size of an investment or portfolio grows.
Applications to Finance:
- Portfolio Management: Understanding how risks and returns might change as a portfolio grows or shrinks in size.
- Market Impact: Assessing how large trades or investments might move the market, especially in less liquid markets.
- LTCM: LTCM collapsed when its modelers failed to consider the risks in a market due to the size of its own influence. Positions in markets can become increasingly linked for no other reason than the fact that they owned them.
Entropy in Physics and Information Theory: Implications for Finance
Information Theory
Information theory studies the quantification, storage, and communication of information.
Initiated by Claude Shannon in the 1940s, it introduced concepts like entropy to measure the amount of uncertainty or randomness in a source of information.
The theory provides the foundation for data compression, error correction, and much of modern communication technology.
In essence, it’s about efficiently transmitting and storing data while minimizing errors.
Applications to Finance
- Efficient Market Hypothesis (EMH): This theory posits that asset prices reflect all available information. Information theory can help quantify how quickly new information is incorporated into prices.
- Portfolio Optimization: By quantifying the amount of information or uncertainty in asset returns, traders/investors can design portfolios that maximize returns for a given level of risk.
- Signal Processing: Traders might use techniques from information theory to filter out “noise” from market data, focusing on meaningful trends or signals.
- Risk Management: By understanding the entropy (or uncertainty) of financial instruments, firms can better gauge and manage the inherent risks.
In essence, information theory in finance revolves around extracting meaningful insights from vast amounts of market data and making informed decisions based on that.
Boltzmann Entropy
Boltzmann entropy quantifies the number of microscopic configurations that correspond to a macroscopic state.
In finance, it can represent the number of ways a particular financial outcome can be achieved.
Applications to Finance
- Portfolio Diversification: Just as a system with higher entropy has more configurations, a diversified portfolio has multiple paths to achieve a given return, reducing reliance on any single asset.
- Risk Assessment: Understanding the multiplicity of scenarios that can lead to a particular market outcome, aiding in more comprehensive risk modeling.
Shannon Entropy
Shannon Entropy measures the uncertainty or randomness of information in a system.
It quantifies the average amount of information needed to predict the state of a random variable, foundational in information theory and data compression.
In finance, Shannon Entropy can quantify the unpredictability of stock returns or market movements.
A higher entropy suggests wider returns distributions, requiring diversified portfolios.
Conversely, lower entropy indicates more predictable patterns. It aids in assessing market complexity and risk.
Applications to Finance
- Market Efficiency: A market with high Shannon entropy is hard to predict, suggesting efficiency.
- Algorithmic Trading: Algorithms can be designed to identify patterns in data with lower entropy, potentially revealing exploitable structures.
Tsallis Entropy
While not as commonly referenced as others, Tsallis Entropy is a generalization of the standard Shannon Entropy.
It introduces a parameter q that allows for non-extensive statistics, making it suitable for systems that don’t adhere to the usual Boltzmann-Gibbs statistics.
An example would be system with long-range interactions or memory effects.
Given it accounts for non-standard statistical behaviors in complex systems, it’s often used in thermodynamics and information theory.
In finance, it can help in modeling asset returns, understanding market anomalies, and optimizing portfolios in markets that deviate from typical Gaussian distributions.
Applications to Finance
- Complex Financial Instruments: Evaluating the unpredictability or risk of more complex financial products that don’t fit traditional models.
Rényi Entropy
A generalization of Shannon entropy, Rényi entropy encompasses a family of measures that can be tuned to give different weights to rare or frequent events.
It introduces a parameter, which, when varied, can produce a spectrum of entropy measures. For , it reduces to the standard Shannon Entropy.
Rényi Entropy is useful for characterizing the diversity and randomness of a system (i.e., spectrum of entropy values).
In finance, this flexibility can be invaluable.
Applications to Finance
- Tail Risk Assessment: By adjusting the Rényi parameter, one can give more weight to extreme market events, aiding in the assessment of tail risks.
- Market Complexity: Gauging the complexity of market movements, especially in complicated financial environments.
von Neumann Entropy
Von Neumann Entropy quantifies the disorder or uncertainty of a quantum system, described by its density matrix.
Analogous to Shannon Entropy in classical systems, it’s fundamental in quantum information theory, measuring the information content and entanglement of quantum states.
While its direct application to classical finance is less intuitive, it can be applied to situations where mixed strategies* are considered.
It can be applied to quantify the uncertainty or complexity of financial portfolios, especially when considering quantum finance or advanced modeling techniques.
It could help in assessing the “quantum” uncertainty of financial instruments or strategies, guiding risk management.
*(In finance, a mixed strategy can refer to an investment or trading approach that combines multiple methods or asset types. For example, a trader might adopt a mixed strategy by allocating a portion of their portfolio to passive index funds (tracking market performance) while actively trading another portion based on market analysis, aiming to outperform the market.)
Applications to Finance
- Quantum Finance: In emerging fields that explore the intersection of quantum mechanics and finance, von Neumann entropy can play a role in modeling quantum financial systems.
- Mixed Strategy Games: In game-theoretic financial models, von Neumann entropy can quantify the unpredictability of mixed strategies.
Additional Applications of Statistical Mechanics in Finance
Statistical Field Theory
Statistical Field Theory (SFT) is a framework that combines quantum field theory with statistical mechanics to study systems with many degrees of freedom, like elementary particles and superfluids.
While the direct application of SFT to finance is abstract, the underlying principles can be applied.
- Elementary Particle: In finance, elementary “particles” can be thought of as the basic units or entities, such as individual assets or individual agents that make up a market.
- Superfluidity: This phenomenon, where matter behaves without viscosity, can be likened to markets with high liquidity, where transactions occur seamlessly without significant friction, slippage, or spread-related transaction costs.
Applications to Finance
- Market Liquidity: Using principles similar to superfluidity to understand and model markets where assets can be quickly bought or sold without causing significant price changes.
- Financial Interactions: Just as SFT studies interactions among particles, one can study interactions among financial entities, understanding how they collectively influence market dynamics, such as debtors and lenders and governments and private sector entities.
Condensed Matter Physics
This branch of physics studies the properties of solid and liquid matter.
Principles from condensed matter physics can be applied to understand the collective behavior of assets in markets.
Applications to Finance
- Market Structures: Understanding how individual assets (analogous to atoms in materials) come together to form market structures, such as sectors or indices.
- Collective Behavior: Analyzing how individual financial entities influence and are influenced by the collective behavior of the market.
Complex System
Complex systems are characterized by intricate interactions and feedback loops, leading to emergent behaviors that are not easily predictable from the individual components.
- Chaos: Chaotic systems are highly sensitive to initial conditions. Economies and financial markets, with their vast web of interactions, can exhibit chaotic behaviors, especially during crises.
- Information Theory: This theory quantifies information and can be used to understand the flow and impact of information in financial markets.
- Boltzmann Machine: A type of artificial neural network inspired by statistical mechanics. It can learn and recognize complex patterns, making it useful for financial modeling and prediction.
Applications to Finance
- Market Predictability: Using chaos theory to understand and model the unpredictability of financial markets, especially during turbulent times.
- Information Flow Analysis: Using information theory to model how news and data influence market movements and trader/investor behaviors.
- Financial Forecasting: Leveraging neural networks to recognize patterns in historical data and predict future market movements.
Criticisms of Statistical Mechanics in Finance
Criticisms of the use of these concepts generally lie in the fact that work has already been done in finance to solve various problems, and applications of many mathematical frameworks outside of the standard fare (i.e., pure math or math applied toward physical systems) are more theoretical or just metaphorical.
However, the future of quantitative finance is inherently about building new paths. In the search for alpha in quant finance, one ultimately needs to be doing new things.
Traders who are using the same models and same data should expect similar results.
A huge dose of independent thinking is necessary for generating alpha in markets.
Just as the mean-variance framework that came into use in the 1950s is widely known as too simplistic, eventually so too will today’s quant models (though many of the foundations and cause-effect mechanics involved in markets and economies are universal and last over time).
FAQs – Statistical Mechanics & Applications to Finance
Theory:
What is the Principle of Maximum Entropy and why is it significant?
The Principle of Maximum Entropy states that, given a set of constraints, the probability distribution that best represents the current state of knowledge is the one with the largest entropy.
In simpler terms, it suggests that we should choose the most unbiased, or least informative, distribution consistent with our knowledge.
This principle is significant because it provides a systematic method to assign probabilities in situations with non-deterministic outcomes (such as financial markets).
In financial markets, the Principle of Maximum Entropy can be used to derive the most unbiased probability distribution for asset returns given limited/known information.
What are the basics of Ergodic Theory?
Ergodic Theory studies the long-term average behavior of systems that evolve over time.
In the context of statistical mechanics, a system is said to be ergodic if its time average is equal to its ensemble average.
This means that, given enough time, the system will explore all accessible microstates equally.
Traders might use Ergodic Theory to predict that a stock’s long-term average performance will match its historical average because of what it’s fundamentally like.
Statistical Thermodynamics:
What are Ensembles in Statistical Mechanics?
Ensembles are collections of a large number of virtual copies of a system, each representing a possible microstate.
The three main types of ensembles are the Microcanonical (NVE), Canonical (NVT), and Grand Canonical (µVT) ensembles.
They are used to describe the statistical behavior of systems in different conditions.
Financial analysts use ensemble methods to simulate various market scenarios and predict the outcome (e.g., from an expected value standpoint).
How does the Partition Function relate to the behavior of a system?
The Partition Function, often denoted as Z, is a mathematical function that encapsulates all the statistical information about a system.
It plays a central role in linking the microscopic properties of molecules to the macroscopic thermodynamic properties.
By knowing the partition function, one can derive various thermodynamic quantities like energy, entropy, and pressure.
In finance, analogous concepts to the Partition Function might help in understanding the overall behavior of a portfolio from its individual assets.
What are the Thermodynamic Potentials and their significance?
Thermodynamic potentials are functions used to describe the energy changes in a system.
The four main potentials are:
- U (Internal Energy): Total energy of a system.
- H (Enthalpy): Total energy plus the product of pressure and volume.
- F (Helmholtz Free Energy): Energy available to do work at constant temperature and volume.
- G (Gibbs Free Energy): Energy available to do work at constant temperature and pressure. Their significance lies in predicting the direction of spontaneous processes and determining equilibrium states.
The concept of Gibbs Free Energy can be likened to assessing the potential profitability of an investment, considering both risks and rewards.
What are Maxwell Relations and why are they important?
Maxwell Relations are a set of equations derived from the exact differentials of the thermodynamic potentials.
They provide relationships between various partial derivatives of state functions.
These relations are important because they allow us to relate measurable quantities, like temperature and pressure, to changes in entropy, volume, and other state functions.
These can be used in finance to relate various market indicators, helping in predicting one variable based on others.
Models:
How do Ferromagnetism Models like Ising and Potts help in understanding magnetic systems?
Ferromagnetism models, such as the Ising and Potts models, are mathematical representations used to describe the behavior of magnetic spins in a lattice.
These models help in understanding phase transitions, critical phenomena, and the behavior of magnetic systems at different temperatures.
Analogously, models like Ising could be used to understand market sentiment shifts in financial systems.
What is the significance of Particles with Force Field models like the Lennard-Jones Potential?
Models like the Lennard-Jones Potential describe the interaction between pairs of neutral atoms or molecules.
The potential represents the balance between the attractive and repulsive forces.
Such models are fundamental in understanding molecular dynamics, phase transitions, and the properties of real gases.
Lennard-Jones Potential-like models might be used to describe the balance of buying and selling pressures in asset markets, which can lead to trending moves.
Mathematical Approaches:
How does the Boltzmann Equation describe the evolution of a system?
The Boltzmann Equation describes the statistical behavior of a thermodynamic system not in equilibrium.
It provides a link between the macroscopic properties of a system and the microscopic dynamics of its particles.
The equation is fundamental in understanding gas dynamics, transport phenomena, and relaxation processes.
In finance, it can be analogous to describing the evolution of market dynamics over time.
What is the H-Theorem and its implications in Statistical Mechanics?
The H-Theorem, derived from the Boltzmann Equation, states that the entropy of an isolated system always increases, reaching a maximum at equilibrium.
This theorem provides a statistical foundation for the Second Law of Thermodynamics.
This concept can be applied to understand the tendency of financial markets to move toward their various forms of equilibrium.
Critical Phenomena:
What is Phase Transition and why is it crucial in Statistical Mechanics?
A phase transition refers to the transformation of a system from one state of matter to another, like from a liquid to a gas.
In statistical mechanics, phase transitions help in understanding the macroscopic changes in a system resulting from microscopic interactions.
In finance, a phase transition might describe a sudden market shift, like a change in monetary policy.
How do Critical Exponents like Correlation Length and Size Scaling help in understanding phase transitions?
Critical exponents describe how physical quantities diverge as a system approaches a critical point during a phase transition.
For instance, the Correlation Length exponent describes how fluctuations in a system grow as it nears a critical point.
These exponents provide insights into the universal behavior of systems near phase transitions.
In financial markets, these can help understand how market volatility scales as it approaches critical events like economic downturns (i.e., too much debt coming due and not enough income, savings, and new credit availability to service it).
Entropy:
How do different entropy definitions like Boltzmann, Shannon, and von Neumann differ from each other?
While all these definitions measure the amount of disorder or uncertainty in a system, they are applied in different contexts:
- Boltzmann entropy is used in classical thermodynamics.
- Shannon entropy is used in information theory to measure the information content.
- von Neumann entropy is used in quantum mechanics to describe the uncertainty of a quantum state.
In finance, Shannon entropy might be used to measure the distribution of stock returns.
Applications of Statistical Mechanics:
How does Statistical Field Theory apply to areas like Elementary Particle physics and Superfluidity?
Statistical Field Theory combines quantum field theory with statistical mechanics.
In Elementary Particle physics, it helps in understanding the behavior of particles at high temperatures.
In the context of Superfluidity, it looks into the collective behavior of particles and the emergence of macroscopic quantum phenomena.
This can be applied to finance to understand how assets interact with each other in a market under various conditions.
How does Statistical Mechanics relate to Complex Systems like Chaos and Information Theory?
Statistical mechanics provides tools to understand the behavior of large systems with many interacting components.
In Chaos Theory, it helps in understanding the unpredictability and complex behavior of deterministic systems.
In Information Theory, concepts like entropy measure the amount of information or uncertainty in a system.
In finance, chaos theory can describe how seemingly small things (e.g., a virus originating in China) can end up causing huge economic and market effects.
An economic bubble in another classic example of a chaotic system.
Information theory can aid in quantifying and analyzing the transmission of market information, potentially enhancing trading strategies and risk management.
What are degrees of freedom?
Degrees of freedom refer to the number of independent ways something can move or vary without breaking any rules or constraints.
In finance, degrees of freedom can refer to the number of independent choices or variables in a model or analysis.
For example, when forecasting stock prices, if you consider both historical prices and interest rates, you have two degrees of freedom.
Too many degrees can lead to overfitting, where a model might perform well on past data but poorly on new data, which is one of the primary risks with using AI in financial forecasting.