Portfolio Optimization Techniques
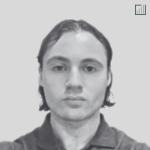
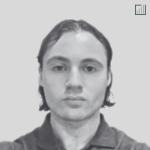
Portfolio optimization is a very important aspect of financial management and trading and investment strategies.
It involves the process of selecting the optimal mix of assets to maximize returns while minimizing risk, allowing traders/investors to achieve their financial goals.
Over the years, various techniques have been developed to improve the efficiency and effectiveness of portfolio management.
In this article, we look at the most prominent methods, providing a comprehensive understanding of these techniques and how they can be applied in different investment contexts.
Key Takeaways – Portfolio Optimization Techniques
- Portfolio optimization techniques, such as Utility Maximization Models, Markowitz Model, and Risk Parity, help investors select the optimal mix of assets to maximize returns and minimize risk.
- These approaches consider factors like investor preferences, risk tolerance, and asset correlations to construct well-balanced and diversified portfolios.
- Advanced approaches, like Hierarchical Risk Parity, Black-Litterman Model, and Conditional Value-at-Risk Optimization, enhance portfolio optimization by incorporating hierarchical asset correlations, investor-specific expectations, and tail risk measures.
- These methods aim to improve risk-adjusted performance and resilience in different market conditions.
- Mathematical tools, including linear programming, quadratic programming, and meta-heuristic methods, provide the foundation for portfolio optimization.
- These tools help investors model and solve complex optimization problems, considering factors like asset returns, risk factors, constraints, and uncertainties.
- Nonetheless, regulatory constraints, taxes, and transaction costs can pose challenges to achieving optimal portfolios in real-world scenarios.
Portfolio Optimization Methods and Techniques
Below we have various forms of portfolio optimization methods and techniques:
Utility Maximization Models
The Utility Maximization Model is an approach based on the concept of utility, which refers to the satisfaction or happiness gained from consuming goods or services.
In this context, the model seeks to maximize the expected utility of an investor’s portfolio.
This is achieved by considering the investor’s preferences and risk tolerance, along with the potential returns and risks of different assets.
By assigning a utility score (or by using various utility metrics) to each possible portfolio, investors can identify the one with the highest utility, therefore maximizing their overall satisfaction.
Markowitz Model
The Markowitz Model, also known as Modern Portfolio Theory, is one of the most influential and widely-used portfolio optimization techniques.
Developed by Harry Markowitz in 1952, this model focuses on the trade-off between risk and return.
By diversifying investments across different assets, the Markowitz Model seeks to minimize risk while maintaining a desired level of return.
This is achieved by constructing an efficient frontier, which represents the optimal set of portfolios that offer the best risk-return balance for a given level of risk tolerance.
Risk Parity
Risk Parity is a portfolio optimization technique that aims to equalize the contribution of each asset to the overall portfolio risk.
This method, unlike traditional approaches, does not rely on historical returns. Instead, it focuses on the volatility of assets and their correlations.
By allocating capital in such a way that each asset contributes equally to the portfolio’s risk, investors can achieve a more balanced and diversified portfolio, potentially improving risk-adjusted returns.
Hierarchical Risk Parity
Hierarchical Risk Parity (HRP) is an advanced risk parity approach that uses a hierarchical clustering algorithm to group assets based on their correlations.
This method aims to improve diversification and reduce the impact of estimation errors in correlation and covariance matrices.
The HRP technique begins by constructing a hierarchical tree of assets, which is then used to determine an optimal allocation that equalizes risk contributions.
By accounting for the hierarchical structure of asset correlations, HRP can enhance the risk-adjusted performance of a portfolio.
Black-Litterman Model
The Black-Litterman Model, developed by Fischer Black and Robert Litterman, is a portfolio optimization technique that combines an investor’s views on the market with the market equilibrium to derive optimal asset allocations.
This method overcomes some of the limitations of the Markowitz Model, such as sensitivity to input parameters and the tendency to generate extreme weights for assets.
For example, the Markowitz Model (i.e., Modern Portfolio Theory) uses past correlations and past returns, which may not align with what a trader/investor expects going forward.
Moreover, if the Markowitz Model is balancing risk allocations, if it wanted to strike a balance between stocks and short-term bonds (or cash), it might place an extreme weight on the short-term bonds or cash to try to equalize the risk.
The Black-Litterman Model incorporates investor-/trader-specific expectations into the portfolio construction process, resulting in more intuitive and diversified portfolio allocations.
Minimum Variance Portfolio
The Minimum Variance Portfolio (MVP) is an optimization technique that focuses on minimizing the portfolio’s overall volatility.
This approach constructs a portfolio that achieves the lowest possible risk, regardless of expected returns.
There are also variations of the MVP approach, such as meeting certain return thresholds, like the rate of inflation.
MVP is particularly useful for risk-averse investors who prioritize capital preservation over maximizing returns.
By combining assets with low correlations and minimizing the overall volatility, the MVP approach can deliver more stable and consistent performance over time.
Maximum Drawdown Portfolio
The Maximum Drawdown Portfolio (MDP) optimization technique aims to minimize the portfolio’s maximum drawdown, which is the largest peak-to-trough decline in the value of a portfolio over a specified period.
Drawdowns are a critical measure of risk, as they capture the potential loss an investor might experience during adverse market conditions.
By minimizing the maximum drawdown, this approach seeks to reduce the risk of significant losses and improve the portfolio’s risk-adjusted performance.
The MDP technique incorporates the historical drawdowns of individual assets, along with their correlations, to construct a portfolio that exhibits the lowest possible maximum drawdown.
Constant Proportion Portfolio Insurance (CPPI)
Constant Proportion Portfolio Insurance (CPPI) is a dynamic portfolio optimization technique that aims to protect a predetermined minimum level of portfolio value while participating in the upside potential of risky assets.
The CPPI approach involves dividing the portfolio into a risk-free asset, typically bonds or cash, and a risky asset, such as stocks.
The allocation to the risky asset is determined by a constant multiple of the difference between the current portfolio value and the predetermined minimum value.
By dynamically adjusting the allocation, CPPI can help investors maintain a desired level of risk while still benefiting from potential market gains.
Conditional Value-at-Risk (CVaR) Optimization
Conditional Value-at-Risk (CVaR), also known as Expected Shortfall, is a risk measure that captures the average loss experienced during extreme negative events, beyond a specified confidence level.
CVaR optimization focuses on minimizing the expected losses during such events, making it particularly suitable for investors who are concerned about the impact of tail risks on their portfolios.
By optimizing the allocation of assets based on their CVaR, this technique can help construct more resilient portfolios that are better prepared to withstand market downturns and extreme events.
Equal Risk Contribution (ERC) Portfolio
The Equal Risk Contribution (ERC) Portfolio is a risk-based optimization technique that aims to allocate capital such that each asset contributes equally to the overall portfolio risk.
This approach is similar to Risk Parity but extends the concept to cover not only the individual asset risks but also their interactions, as measured by the correlations and covariances between assets.
By considering both individual asset risks and their interdependencies, the ERC Portfolio can achieve a more balanced and diversified allocation, potentially improving risk-adjusted returns.
Bayesian Networks
Bayesian Networks are a probabilistic modeling technique that can be used to analyze and optimize portfolios.
They represent the relationships between variables, such as asset returns, economic indicators, and market factors, in a graphical structure.
By quantifying these relationships through conditional probabilities, Bayesian Networks can provide insights into the potential impact of various factors on portfolio performance.
This approach allows investors to update their portfolio allocations based on new information, dynamically adjusting to changing market conditions and improving decision-making.
Black-Scholes Model
The Black-Scholes Model is a mathematical model for pricing options, a type of financial derivative.
While not directly related to portfolio optimization, the Black-Scholes Model plays an important role in the management of portfolios containing options and other derivatives.
By providing a theoretically sound method for valuing options, the model enables investors to make informed decisions about the optimal mix of assets and derivatives, therefore enhancing their ability to manage risk and achieve their investment objectives.
Even though Black-Scholes was developed in the early-1970s it is still widely used today.
Kelly Criterion
The Kelly Criterion is a money management and betting strategy that seeks to optimize the long-term growth rate of a portfolio.
It involves determining the optimal fraction of a portfolio to allocate to a specific investment, based on the expected return and risk.
The Kelly Criterion can be applied to various investment contexts, including stock trading, professional gambling, sports betting, and other games like trading.
By following the Kelly Criterion, investors can potentially maximize their long-term wealth while minimizing the risk of ruin.
Dynamic Asset Allocation
Dynamic Asset Allocation is a portfolio management strategy that involves adjusting the allocation of assets in response to changing market conditions, economic factors, and investment opportunities.
This approach contrasts with static asset allocation, which maintains a fixed allocation regardless of market fluctuations.
Dynamic Asset Allocation aims to capitalize on market trends and exploit mispricings while managing risk more effectively.
By continuously monitoring market conditions and adjusting allocations accordingly, investors can potentially improve their risk-adjusted returns and achieve better long-term performance.
Multi-Objective Optimization
Multi-Objective Optimization is an advanced portfolio optimization technique that seeks to balance multiple objectives, such as risk, return, liquidity, and environmental, social, and governance (ESG) factors.
This approach recognizes that investors often have multiple, sometimes conflicting, goals and aims to find an optimal compromise among them.
By using mathematical methods and algorithms, Multi-Objective Optimization can identify the trade-offs between different objectives and help investors construct portfolios that best meet their unique preferences and requirements.
Statistical Arbitrage
Statistical Arbitrage is a quantitative trading strategy that seeks to exploit temporary mispricings between related financial instruments.
While not a portfolio optimization technique per se, it can be used as a component of an investment strategy to enhance risk-adjusted returns.
Statistical Arbitrage relies on sophisticated statistical models and high-speed trading algorithms to identify and exploit short-term price inefficiencies. (Therefore, it’s mostly used at the institutional level.)
By incorporating Statistical Arbitrage strategies into their portfolios, traders/investors can potentially generate additional returns and improve the overall performance of their investments.
Mathematical Tools for Portfolio Optimization
Mathematical tools play a vital role in portfolio optimization, as they provide the foundation for constructing efficient and well-diversified portfolios.
They can help a trader/investor be more objective when employed correctly.
By utilizing various mathematical programming techniques and algorithms, investors can better understand the trade-offs between risk and return, and make better decisions about asset allocation.
Below we look at the most prominent mathematical tools used in portfolio optimization, exploring their applications and benefits.
Linear programming
Linear programming (LP) is an optimization technique that deals with linear objective functions and linear constraints.
It is widely used in finance to solve various problems, including portfolio optimization, where the goal is to maximize or minimize a linear objective function – such as expected return or risk – subject to linear constraints (e.g., budget and diversification requirements).
LP can be used to find the optimal allocation of assets in a portfolio, ensuring that the desired return is achieved while adhering to the specified constraints.
Quadratic programming
Quadratic programming (QP) is an extension of linear programming, wherein the objective function is quadratic rather than linear.
QP is particularly useful for portfolio optimization problems that involve the trade-off between risk and return, as risk is often modeled as a quadratic function, like portfolio variance.
The Markowitz Model, one of the most well-known portfolio optimization techniques, is based on quadratic programming, as it seeks to minimize portfolio risk subject to a target level of return.
Nonlinear programming
Nonlinear programming (NLP) is a general optimization technique that deals with objective functions and constraints that can be nonlinear.
NLP can be used to solve complex portfolio optimization problems that involve nonlinear relationships between assets, risk factors, or trader/investor preferences.
For example, NLP can be applied to problems involving:
- non-normal return distributions (most financial returns are fat-tailed)
- downside risk measures, or
- transaction costs (tend to increase in a nonlinear way as portfolio size increases)
All of these often exhibit nonlinear behavior.
Mixed integer programming
Mixed integer programming (MIP) is an optimization technique that allows for both continuous and integer variables.
MIP is useful for portfolio optimization problems that involve discrete choices, such as selecting a specific number of assets, or implementing threshold-based rules for asset allocation.
By combining continuous and integer variables, MIP can model a wide range of practical constraints and investment rules, leading to more realistic and implementable portfolio solutions.
Meta-heuristic methods
Meta-heuristic methods are a class of optimization algorithms that explore the search space of potential solutions in a guided, yet stochastic manner.
These methods, such as simulated annealing, particle swarm optimization, and ant colony optimization, are particularly useful for solving complex, non-convex optimization problems that may have multiple local optima.
Meta-heuristic methods can be applied to portfolio optimization to find near-optimal solutions, even in the presence of complex, nonlinear relationships and constraints.
We have a simplified explanation of meta-heuristic methods in the FAQ section below.
Stochastic programming for multistage portfolio optimization
Stochastic programming is a mathematical optimization technique that incorporates uncertainty into the decision-making process.
In the context of multistage portfolio optimization, stochastic programming can model the evolution of asset prices, interest rates, and other financial variables over time, allowing investors to make dynamic allocation decisions based on multiple possible scenarios.
This approach can help investors better manage risk and achieve more robust, adaptive portfolios that perform well under various market conditions.
Copula based methods
Copula-based methods are a statistical approach for modeling the dependence structure between multiple variables, such as asset returns.
By capturing the joint behavior of assets more accurately than traditional correlation measures, copulas can provide insights for portfolio optimization, especially in the context of tail risk management and extreme events.
Copula-based methods can be combined with various optimization techniques, such as linear programming or stochastic programming, to construct more resilient and diversified portfolios.
Principal component-based methods
Principal component analysis (PCA) is a dimensionality reduction technique that can be used to identify the main sources of variation in a dataset, such as asset returns.
By transforming the original variables into a smaller set of uncorrelated principal components, PCA can help simplify portfolio optimization problems and improve estimation accuracy.
Principal component-based methods can be particularly useful for large, high-dimensional datasets, where traditional optimization techniques may struggle with estimation errors and overfitting.
By incorporating PCA into the portfolio optimization process, traders/investors can better understand the underlying drivers of asset returns and construct more efficient portfolios.
Deterministic global optimization
Deterministic global optimization (DGO) is a class of optimization techniques that seek to find the global optimum of a given problem, rather than just a local optimum.
DGO methods, such as branch and bound, interval arithmetic, and cutting planes, systematically explore the search space of potential solutions, guaranteeing that the global optimum is found within a specified tolerance.
DGO can be applied to portfolio optimization problems with non-convex, nonlinear objective functions or constraints, ensuring that the best possible portfolio is identified.
We have a simplified explanation of DGO in the FAQ section below.
Genetic algorithm
As with some on this list, many approaches are brought into finance from other disciplines.
Genetic algorithms (GAs) are a type of meta-heuristic optimization method inspired by the process of natural selection and evolution in biology.
GAs use a population-based approach, where multiple candidate solutions are evolved over time using operators such as selection, crossover, and mutation.
Genetic algorithms can be applied to a wide range of portfolio optimization problems, including those with complex, nonlinear relationships, multiple objectives, and various constraints.
By mimicking the process of natural evolution, GAs can efficiently search the solution space and find near-optimal portfolios, even in the presence of challenging optimization landscapes.
Impediments to Portfolio Optimization
Portfolio optimization aims to maximize returns while minimizing risks by carefully selecting and allocating assets within a portfolio.
However, several impediments can make achieving optimal portfolios challenging in real-world situations.
We look at three major obstacles to portfolio optimization – regulation, taxes, and transaction costs – and discuss their implications for traders/investors.
Regulation
Regulatory frameworks play a significant role in the financial markets and can directly impact portfolio optimization.
Regulations can impose constraints on the types and amounts of assets that investors can hold, affecting the available investment opportunities and the ability to construct an optimal portfolio.
Some examples of regulatory constraints include:
Restrictions on certain types of investments
Regulations may prohibit investors from investing in specific assets or asset classes, such as high-risk investments, which can limit diversification opportunities and hinder portfolio optimization.
Capital requirements
Regulatory capital requirements can impact portfolio optimization by limiting the amount of leverage an investor can use.
This constraint can reduce the potential returns that can be generated from the portfolio.
Capital requirements are popular with banks, as we cover more in this article.
Risk management requirements
Regulations may require investors to adhere to specific risk management practices, such as limiting exposure to certain assets or maintaining a minimum level of diversification.
These requirements can impose additional constraints on the portfolio optimization process, potentially leading to suboptimal portfolios.
Taxes
Taxes can significantly influence the performance of investment portfolios, as they directly impact the after-tax returns of assets.
Tax considerations can complicate the portfolio optimization process in several ways:
Different tax treatment of asset types
Various assets may be subject to different tax rates or tax treatments, such as long-term capital gains, short-term capital gains, and dividend taxes.
This disparity can make it challenging to compare assets and construct optimal portfolios.
Tax implications of rebalancing
Regularly rebalancing a portfolio to maintain the optimal asset allocation can trigger taxable events, such as capital gains taxes.
These taxes can reduce the overall portfolio returns and complicate the optimization process.
In another article, we talk about the different rebalancing approaches.
Tax-loss harvesting
Investors may engage in tax-loss harvesting to offset realized capital gains with realized losses.
This strategy can help reduce tax liabilities but may also impose additional constraints on the portfolio optimization process.
Transaction Costs
Transaction costs are another critical impediment to portfolio optimization.
These costs, which include brokerage fees, bid-ask spreads, and market impact costs, can significantly impact portfolio performance by eroding returns.
The larger a portfolio, the more transaction costs tend to go up (i.e., they’re nonlinear).
Transaction costs can affect portfolio optimization in several ways:
Reduced returns
High transaction costs can reduce the net returns of assets, making it more challenging to achieve the desired risk-return trade-off within the portfolio.
Frequent trading
Portfolio optimization techniques that involve frequent trading or rebalancing can incur high transaction costs, potentially negating the benefits of optimization.
Market impact
Large trades can impact market prices, leading to price slippage and reduced returns.
This market impact can make it difficult to execute optimal portfolio allocations, especially for illiquid assets or large institutional investors.
Conclusion
By and large, all traders and investors want to maximize their return per unit of risk while keeping their portfolios within acceptable risk parameters.
Portfolio optimization techniques are important tools for traders and investors seeking to maximize their portfolios in these ways.
By understanding the various methods available, traders/investors can choose the most appropriate approach for their specific needs and preferences.
From traditional models like the Markowitz Model to more advanced techniques such as Multi-Objective Optimization, these methods offer insights and guidance for constructing efficient and well-balanced portfolios.
By employing these techniques, investors can make more informed decisions, enhance their risk management, and ultimately achieve their financial goals.
Mathematical tools play a vital role in portfolio optimization, providing investors with the means to model complex relationships between assets, risk factors, and investor preferences.
By leveraging these techniques, ranging from linear and quadratic programming to meta-heuristic methods and copula-based approaches, traders/investors can better understand the trade-offs between risk and return, and construct more efficient, well-diversified asset mixes.
Naturally, portfolio optimization will continue to evolve. Especially in institutional management, it’s most important than ever, especially in more systematic approaches.
More advanced mathematical tools and techniques will be developed for a variety of different needs and reasons, further enhancing our ability to manage investments and achieve certain goals.
While portfolio optimization techniques aim to provide investors with the most efficient allocation of assets, real-world constraints such as regulation, taxes, and transaction costs can hinder this process.
By understanding these impediments and incorporating them into the portfolio optimization process, traders/investors can better navigate these challenges and construct more realistic, effective investment portfolios.
FAQs – Portfolio Optimization Techniques
What is the main goal of portfolio optimization?
The primary goal of portfolio optimization is to maximize return and minimize risk for a given investment portfolio.
This is achieved by selecting the best combination of assets and determining the optimal allocation of capital among them.
Portfolio optimization techniques help investors create well-diversified portfolios that align with their risk tolerance and investment objectives.
What are some portfolio optimization techniques besides basic utility maximization models, Markowitz model, and risk parity?
There are several other portfolio optimization techniques that can be considered besides Utility Maximization Models, Markowitz Model, and Risk Parity.
Here are some of them:
- Black-Litterman Model: This model is an extension of the Markowitz Model that incorporates investor views on the market, helping to reduce the impact of estimation errors on portfolio allocation.
- Minimum Variance Portfolio: This technique seeks to minimize the portfolio’s variance, which results in a portfolio that is less volatile than the market. This approach is commonly used by risk-averse investors.
- Maximum Drawdown Portfolio: This technique aims to minimize the maximum loss that a portfolio can experience over a given time period. This approach is often used by investors who are focused on downside risk.
- Constant Proportion Portfolio Insurance (CPPI): This approach involves dynamically allocating funds between a risky asset and a risk-free asset to ensure that the portfolio’s value never falls below a pre-defined level.
- Conditional Value-at-Risk (CVaR) Optimization: This technique focuses on minimizing the expected tail risk of the portfolio. It is commonly used by investors who are concerned about extreme downside events.
- Equal Risk Contribution (ERC) Portfolio: This approach aims to allocate capital in such a way that each asset in the portfolio contributes equally to the overall portfolio risk.
- Bayesian Networks: Bayesian networks are a probabilistic graphical model that can be used to model dependencies between assets in a portfolio. By considering these dependencies, the model can be used to optimize the portfolio allocation.
- Black-Scholes Model: This model is widely used in options pricing and can be adapted to portfolio optimization by taking into account the expected return and volatility of each asset in the portfolio.
- Kelly Criterion: This technique involves allocating capital to each asset in the portfolio in proportion to the expected return and risk of each asset. The goal is to maximize the portfolio’s long-term growth rate.
- Hierarchical Risk Parity: This approach seeks to allocate risk across multiple levels of a portfolio, taking into account the correlations between assets at different levels.
- Dynamic Asset Allocation: This technique involves adjusting the portfolio allocation over time based on how markets change and economic indicators.
- Multi-Objective Optimization: This approach considers multiple objectives, such as maximizing returns while minimizing risk and transaction costs, when optimizing the portfolio allocation.
No single approach is optimal for all investors or all market conditions, and the best portfolio optimization technique may depend on a variety of factors.
For institutional investors that invest based on a more systematic approach, they usually develop their own models that are proprietary in nature.
What is a simplified way of explaining meta-heuristic methods?
Since meta-heuristics methods can be a little abstruse, here’s a simpler explanation:
Imagine you have a puzzle (i.e., deciding how to allocate the portfolio) with lots of different ways to solve it, but you don’t know the best way.
Meta-heuristic methods are like having a special tool that helps you find a good solution without trying every single possibility (like using guess-and-check as a human might).
Instead of trying every single option one by one, the tool helps you make smart guesses. It looks at the puzzle and uses some clever rules to decide which guesses are likely to be better than others.
The tool keeps making guesses and checking if they are good or not. It learns from each guess and tries to make better guesses each time. It keeps doing this until it finds a good solution or it can’t find any better ones.
So, in simple words, meta-heuristic methods are like a smart tool that helps you find good solutions to a difficult puzzle without trying every single possibility.
What is a simplified way of explaining deterministic global optimization?
A portfolio is like fitting a puzzle together. So, imagine you have a big puzzle with lots of different pieces, and your goal is to find the very best way to put them all together.
Deterministic global optimization is like using special techniques to solve the puzzle and find the absolute best solution possible, instead of just settling for a good solution.
To do this, you use methods like “branch and bound,” which means dividing the puzzle into smaller parts and exploring each part very carefully.
“Interval arithmetic” and “cutting planes” help make educated guesses and eliminate less desirable options.
These techniques make sure you don’t miss any possible solutions, so you can be confident that you’ve found the very best way to solve the puzzle.
You can use these techniques to solve problems like deciding the best way to trade or invest money, where you want to find the most optimal solution considering different factors and constraints.
How does the Markowitz Model contribute to portfolio optimization?
The Markowitz Model, also known as Modern Portfolio Theory (MPT), is a portfolio optimization technique that introduced the concept of risk-return trade-off.
The model seeks to minimize portfolio risk, measured as the portfolio’s variance or standard deviation, for a given level of expected return or vice versa.
By considering both risk and return, the Markowitz Model allows investors to construct efficient portfolios that optimize their performance based on their risk tolerance.
How are linear programming and quadratic programming used in portfolio optimization?
Linear programming (LP) and quadratic programming (QP) are mathematical optimization techniques that can be used to solve portfolio optimization problems.
LP deals with linear objective functions and constraints, while QP handles quadratic objective functions.
In portfolio optimization, LP can be used to find the optimal allocation of assets subject to linear constraints, while QP is particularly useful for problems involving the trade-off between risk and return, such as the Markowitz Model.
What’s the difference between single-objective and multi-objective optimization in portfolio management?
Single-objective optimization focuses on maximizing or minimizing a single objective, such as risk or return.
In contrast, multi-objective optimization seeks to balance multiple objectives, such as risk, return, liquidity/illiquidity, and ESG factors.
Multi-objective optimization recognizes that investors often have multiple, sometimes conflicting, goals and aims to find an optimal compromise among them.
Just about everyone knows about the trade-off between risk and return that’s a challenge for any trader/investor.
If you don’t take enough risk, you won’t make any money. If you take too much risk, you won’t keep money. So multi-objective optimization works to strike the right balance.
How do genetic algorithms and other meta-heuristic methods contribute to portfolio optimization?
Genetic algorithms and other meta-heuristic methods are optimization techniques that explore the search space of potential solutions in a guided, yet stochastic manner.
These methods can efficiently search for near-optimal solutions in complex, non-convex optimization problems, such as those found in portfolio optimization.
By incorporating meta-heuristic methods (e.g., genetic algorithms, simulated annealing, particle swarm optimization), traders/investors can tackle challenging optimization problems and improve their portfolio’s performance.
How can stochastic programming be used for multistage portfolio optimization?
Stochastic programming is a mathematical optimization technique that incorporates uncertainty into the decision-making process.
In multistage portfolio optimization, stochastic programming can model the change in asset prices, interest rates, and other financial variables, enabling traders/investors to make dynamic allocation decisions based on various potential scenarios.
This approach helps investors better manage risk and achieve more robust, adaptive portfolios that perform well across market conditions (e.g., rising growth, falling growth, rising inflation, falling inflation, etc.).
What role do copula-based methods play in portfolio optimization?
Copula-based methods are a statistical approach for modeling the dependence structure between multiple variables.
By capturing how different assets act in relation to each other more accurately than traditional correlation measures, copulas can provide valuable insights for portfolio optimization, especially when it comes to tail risk management and extreme events.
Combining copula-based methods with various other optimization techniques can help construct more resilient and diversified portfolios.