25+ Options Pricing Models – Ways to Value Options & Derivatives
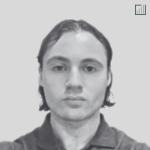
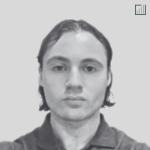
There are many options pricing models with complex mathematical foundations and variables that go into determining what an option is worth.
But in terms of the big-picture intuitive understanding of an option’s value is, it really boils down to two main factors:
- the probability that an option will be in the money (ITM) by expiration (i.e., have value), and
- by how much
Everything is essentially wrapped up under these two variables.
Summary – Options Pricing Models
- Binomial Option Pricing Model: This model uses a “tree” methodology to create a multi-period binomial lattice for valuing options. Assumes that the price of the underlying asset can move to two possible values over each time step. As the number of steps increases, the model’s accuracy improves.
- Black-Scholes Model: A staple. Assumes a continuous-time framework and uses variables like stock price, strike price, volatility, time to expiration, and risk-free interest rate. Suited for European options (can only be exercised at expiration).
- Black Model: Aka the Black-76 model, an adaptation of the Black-Scholes model for pricing futures options. Widely used for pricing bond options, interest rate caps and floors, and swaptions.
- Finite Difference Methods: Involves numerical solutions to the differential equations governing option pricing. Particularly useful for complex options for which analytical solutions might not exist.
- Garman-Kohlhagen Model: Designed for pricing FX options. Extends the Black-Scholes framework to incorporate two interest rates – one for each currency in the pair.
- Lattice Models: These models, including binomial and trinomial trees, are suited for American options (can be exercised at any time before expiration). Also useful for exotic options with path-dependent features.
- Put-Call Parity: Establishes a relationship between the prices of European put and call options with the same strike price and expiration. Fundamental concept in options pricing theory.
- Monte Carlo Methods: Used for options with complex features or multiple sources of uncertainty. Simulates a vast range of potential future outcomes to calculate an option’s value.
- Real Options Analysis (ROA): Extends options pricing theory to capital budgeting decisions, treating investment opportunities as options.
- Trinomial Tree Model: An extension of the binomial model. Includes an extra step, allowing for a more refined approach in certain scenarios – particularly for exotic options.
- Vanna-Volga Pricing: Applied in currency markets. Adjusts the Black-Scholes model to account for specific risks related to an option’s volatility.
- Carr-Madan Formula: Using Fourier transforms, this approach is efficient for pricing European options – particularly when dealing with complex features or a large number of options.
- Ito’s Stochastic Calculus: Essential for modeling the “random” motion of financial instruments. Forms the basis for many options pricing models.
- Partial Differential Equations (PDEs): Used in models like Black-Scholes, these equations describe how the value of an option evolves over time under different scenarios.
- Quantum Options Pricing Models: Emerging field. Uses quantum computing principles to potentially improve the efficiency and accuracy of traditional options pricing models.
- Dupire Model: Dynamically adjusts volatility based on the underlying asset’s price and time. Creates a detailed volatility surface from market data of various option prices.
- Heston Model: Incorporates a stochastic volatility factor that follows a mean-reverting square-root process. Allows for more realistic modeling of volatility’s random fluctuations over time.
- SABR Model: Models the stochastic behavior of both the underlying asset price and its volatility. Commonly used in interest rate derivative markets.
- Non-Parametric Models: Don’t assume a pre-existing functional form or distribution in price, volatility, or other factors. Trade-off is that they’re more computationally intensive and dependent on lots of data.
- Kernel Smoothing Techniques: Uses kernel functions to estimate probability densities for underlying asset or option prices. Adapts to the actual data shape without assuming specific distributions. Captures complex market behaviors and non-linearities.
- Nearest Neighbor Methods: Estimate soption prices using the prices of similar “neighboring” options or assets. Data-driven approach that’s effective in rich data environments.
- Splines and Interpolation Techniques: Employs spline functions for flexible interpolation or smoothing of price-yield curves or volatility surfaces. More adaptable fit than traditional models.
- Quantile Regression: Focuses on modeling conditional quantiles of option price distributions based on variables like strike price and maturity. Broader perspective on potential outcomes – esp. in distribution tails.
- Artificial Neural Networks (ANNs): Machine learning tools that discern complex, non-linear patterns in financial data for options pricing. Can yield more precise outcomes in markets with intricate/unique dynamics.
Options and expected value
Options are especially valuable for managing risk, making bets in a risk-limited way, and capturing the part of the distribution that might be after.
Especially valuable in options trading is the concept of expected value.
Expected value, in a nutshell, is essentially:
What is your probability of being right multiplied by the reward for being right minus the probability of being wrong multiplied by the penalty for being wrong.
For example, if you’re playing a game and you have a 10 percent chance of being right and a reward of $1,000 for being right and a 90 percent chance of being wrong and a $75 penalty for being wrong, is that a risk that’s worth taking?
From an expected value standpoint, playing this would amount to:
Expected value = $1,000 * 0.10 – $75 * 0.90 = +$25
You have a positive expected value. So as long as you can cover the $75 loss in the chance you’re wrong, your chances of being positively rewarded are high if you play these odds an adequate number of times.
The game just described is akin to buying an out-of-the-money (OTM) option.
Your odds of being right are usually under 50 percent, sometimes significantly less, but your potential reward is so high it can sometimes justify making the trade.
To use a baseball analogy, it’s not your batting average that matters (how many times you put the ball in play to get a hit), but your slugging percentage (the value of the hit when you do put the barrel on the ball).
It’s okay to be wrong a lot so long as you’re adequately compensated for all your misses when you are right.
Increases in the option premium
If any given variable works to increase the option premium, it’s because it increases one or both of the abovementioned factors.
So, a longer time until expiration or higher implied volatility will increase premiums because it increases the chance that the option will be in-the-money (ITM) by expiration and increases the odds of it being ITM by a larger amount.
Similarly, premiums will be lower the more an option is OTM and the lower the implied volatility because the odds of the underlying security, asset, or product reaching the strike price and going above it by expiration is low.
Prices and time are straightforward to measure. However, what can’t be known with a high level of precision is the volatility of the underlying asset.
Therefore, volatility is a key consideration and a big driver of options prices given its outsized impact on the probability of whether an option will be in the money or by how much.
Historical volatility is not necessarily a very good indicator of where volatility will be in the future. It can provide a reasonable approximation of future volatility where the future is likely to be similar to the past but big deviations are possible.
Various options pricing models have been created to more accurately determine what options should be worth, or to price them more effectively when they’re first created.
Let’s take a look at some of the main ones.
Binomial option pricing model
The binomial option pricing model calculates what a call premium should be if the underlying asset can only be of one or two different prices by expiration.
A variable that can only take on two possible values is known as a binomial random variable.
By dividing time into smaller intervals with two possible prices that are closer together, a more accurate option premium can be derived.
As the number of time periods increases, the distribution of possible prices of the underlying asset, security, or product approaches something like a normal distribution – the familiar bell curve.
(Financial returns are more fat-tailed than what’s predicted by the normal distribution. Oftentimes, financial people will refer to big moves in markets as an ‘X-sigma’ move, where X is a number like 5-10, signifying a very rare move. But they’re only seemingly rare because of the flaws associated with using the normal distribution to describe financial returns.)
Black-Scholes model
In 1973, US economists Myron Scholes and Fisher Black developed a mathematical formula for calculating options prices.
Their model was based on variables such as the current price of the underlying security, time until expiration, and volatility (by how much the prices varied over time).
Robert Merton later expanded on this options pricing model, and the Black-Scholes is sometimes also referred to as the Black-Scholes-Merton (BSM) model.
To simplify matters, they based their pricing model on certain assumptions:
- A “no arbitrage rule”: This means that prices reflect all information that’s known about the underlying asset today and into the future. Accordingly, an arbitrageur would not earn guaranteed profits by hedging against future risk.
- A call or put option contract can be used to offset any risk of any portfolio of securities or assets.
- Asset price fluctuations are random, but follow a normal distribution, which means that prices are assumed to not change much over the short run.
Academically, the Black-Scholes formula is the most commonly used formula to calculate options prices.
Part of its popularity is the ease of using it. It still depends on assessing the volatility of the underlying security, which is denoted by the standard deviation, σ, of the underlying asset price.
Black-Scholes Formula
C0 = S0N(d1) – Xe-rtN(d2)
- C0 = current call premium
- S0 = current stock price
- N(d1) = the probability that a value in a normal distribution will be less than d
- N(d2) = the probability that the option will be in the money by expiration
- X = strike price of the option
- T = time until expiration (expressed in years)
- r = risk-free interest rate
- e = 2.71828, the base of the natural logarithm
- ln = natural logarithm function
- σ = standard deviation of the stock’s annualized rate of return (compounded continuously)
d1 = ln(S0/X) + (r + σ2/2)Tσ√T
d2 = d1 – σ√T
Note that:
- Xe-rt = X/ert = the present value of the strike price using a continuously compounded interest rate
Requirements for the Black-Scholes:
- The stock or instrument does not pay a dividend before expiration.
- No changes in interest rates and variance before expiration.
- No discontinuous jumps in stock price (which might otherwise be hedged through gamma hedging).
- The assumption of instant, cost-less trading.
The Black-Scholes formula calculates the premium for a call, but the put premium can be calculated by using the put-call parity formula.
From the Black-Scholes formula, the standard deviation, σ, which measures volatility, can be calculated if the other variables are known.
This is what’s known as implied volatility because it is implied (or essentially backed out) by the other variables.
Some traders will compare the implied volatility with the observed volatility to help determine whether an option is fairly priced.
On many trading platforms, when trading options (especially equity options), the broker will provide implied volatility (IV) readings to the trader.
Why Volatility Increases Time Value and Option Premiums
Let’s consider a couple hypotheticals.
Hypothetical #1
Volatility is the change in the price of the underlying security over time. It’s a big part of what gives options value.
For example, consider a hypothetical security that never changes in price.
An option based on a security of this nature would never have any time value because the underlying is always the same price.
According, if the option was out of the money (put or call) no one would want the option because it would always expire worthless.
At the same time, no one would write (sell) an option based on this security if it was in the money because it would be exercised.
Hypothetical #2
Now let’s say there was a scenario where the price of the security changed according to some formula that everyone knew about.
In other words, anyone could calculate the stock price with certainty at any time.
In this case, again, no option on this stock would have any time value because the price would be known by anyone ahead of time.
For example, if the stock were $100 and it was known that before a certain expiration date that it would be $120, nobody would want to write a 100 call unless they were being compensated $20 per share. (The exception would be if they could charge a little less in order to get the money sooner, in line with current risk-free interest rates.)
The only way the option would have value is if it was discounted adequately to at least equal current interest rates.
Accordingly, the unknown movement in the underlying price of a security is what gives options their value.
Hypothetical #3
Consider two hypothetical securities.
Both trade at $100.
Stock A has ranged between $80 and $120 over the past year. In other words, it’s reasonably stable and this price behavior is expected going forward.
Stock B, on the other hand, is more volatile. It has ranged from $60 to $140 over the past year. Like Stock A, this price behavior is also expected going forward.
If one wanted to buy a call option at a 100 strike, which would have a higher value?
Stock B would have a higher call premium because it has a greater potential payoff.
Even though both have a roughly equal chance of expiring worthless because they’re both at the same current price, from an expected value standpoint, Stock B can move further, giving the option on it a higher intrinsic value.
Criticisms of Black-Scholes
The normality assumption of the Black–Scholes model does not capture extreme movements such as crashes in markets.
Warren Buffett, in a 2008 Berkshire Hathaway shareholder letter wrote:
“I believe the Black–Scholes formula, even though it is the standard for establishing the dollar liability for options, produces strange results when the long-term variety are being valued… The Black–Scholes formula has approached the status of holy writ in finance… If the formula is applied to extended time periods, however, it can produce absurd results. In fairness, Black and Scholes almost certainly understood this point well. But their devoted followers may be ignoring whatever caveats the two men attached when they first unveiled the formula.”
Black Model
The Black model – sometimes known as the Black-76 model (in reference to the year it was introduced to separate it from other Fischer Black models) – is a variation of the Black–Scholes option pricing model.
The Black model is used most heavily for pricing:
The more popular Black-Scholes model is more commonly used for equity options.
The Black model is known as a log-normal forward model.
Finite Difference Methods
Finite difference methods for option pricing involve using numerical methods in the valuation of options. Eduardo Schwartz first applied the method in 1977.
Finite difference methods involve pricing options by approximating the differential equation that best describes how the value of the option changes over time through a series of difference equations.
Discrete-time difference equations can then be solved to find a price for the option.
Finite differences came about because an option’s value can be modeled through a partial differential equation as a function of price (of the underlying security) and time (and potentially other variables).
Once in the form of a partial differential equation, a finite difference model can be derived, which can then yield the valuation of the option.
The finite differences approach can be used to solve option pricing exercises that have the same level of complexity as those solved, perhaps more commonly, by tree approaches.
Garman-Kohlhagen
The value of a European option on a foreign exchange rate is commonly found by assuming that the rate follows a log-normal process. This is similar to the Black–Scholes model for equity options and the Black model for some types of interest rate options.
An FX options pricing model was first published by Biger and Hull in 1983. The model came before Garmam and Kolhagen’s model, which is more popularly referenced today. That same year, Garman and Kohlhagen extended the use of the Black–Scholes model to help solve FX options pricing when there are two interest rates involved in a currency pair – i.e., one associated with each currency.
Suppose that is the risk-free interest rate (to expiry) associated with the domestic currency and
is the foreign currency’s risk-free interest rate.
The domestic currency is the currency where the value of the option is referenced.
The formula also requires that the currency – both strike and current spot price – be quoted in terms of units of domestic currency per unit of foreign currency.
The results are, in turn, expressed in the same units. To have meaning, they need to be converted into one of the currencies.
Lattice model
A lattice model involves the valuation of options and other derivatives in cases where discrete time models are needed.
American options differ from European options in that the former can be exercised at any time while the latter can only be exercised at expiration.
In these cases, a discrete time lattice model would be appropriate for American options because the decision to exercise is always open (at least during market hours) before and including maturity.
A continuous model would be more appropriate for European options where the option’s maturity date is the only exercise period. Black-Scholes is a continuous model.
Exotic options are often valued using lattice models because of unique features of their payoff, such as the path dependency in lookback and Asian options and that impact on their valuations.
Interest rate derivatives and their pricing works better with lattice models as well due to the issues continuous models have with capturing some of their features (e.g., pull to par).
In general, lattice models may have to inform traders that terminating the option early is optimal rather than waiting until expiration.
Continuous models don’t account for this.
Put-call parity
Put–call parity relates to the definition in the prices between a call option and put option (as it pertains to European options), holding expiry and strike price constant.
Under put-call parity, a long call option and a short put option should have the same price as a contract in the forward market at the same strike and expiry.
This should be true because if the price at expiry is above the strike price, the call option will be exercised. If it’s below, the put option will be exercised.
For put-call parity to hold, certain assumptions have to be met. But in reality, financing costs (interest on margin debt/leverage), transactions costs (e.g., spreads between bid and ask), the relationship will not be exact.
In highly liquid markets, such as the SPY options market, put-call parity will be close to holding true.
Monte Carlo
Monte Carlo is typically used as a method of last resort as an options pricing model.
It is commonly used when conducting a valuation with:
- multiple variables that are unknown and can’t be known
- complicated features associated with the derivatives, where continuous models like Black-Scholes or other models that could normally account for exotic options (e.g., lattice models) won’t be effective
Monte Carlo enables customization to fit the appropriate features of the option.
The assumption of normality often underlies options models as a matter of convenience and rough approximation. Monte Carlo enables this to be modified to any distribution.
So when it comes to real options analysis (as it pertains to capital spending decisions), Asian options, and lookback options, Monte Carlo is more common.
Nonetheless, if there is a numeric technique (e.g., pricing tree) or analytical/computational technique for valuing the option that already exists, Monte Carlo is generally the last method to be used.
Real options value or Real options analysis (ROV or ROA)
Real options value, also called real options analysis (ROV or ROA) has to do with options and derivatives valuation as it pertains to corporate budgeting and capital spending decisions.
These are called real options. These provide the right, but not the obligation, to undertake certain business decisions.
This pertains to anything involving a capital spending project, such as contracting, deferring, stopping, expanding, purchasing, and so on.
For example, it could mean the decision to expand a firm’s productive output while at the same time the option to sell productive output (such as a piece of machinery, factory, and so on).
Real options are unique because they are not like traditional financial options that are underwritten on securities that are traded in the markets.
Also, management teams – the holders of these options – can also influence the value of the option on an underlying project. This is less true as it pertains to options on a financial security.
On top of that, management teams also have no distinct measure of volatility. They must rely on their own knowledge of what uncertainty comes along with the project.
And real options don’t naturally exist. It’s not as simple as buying or selling an option that already exists on an underlying security.
So they have to be created and knowing how to do this is a task in itself – finding a counterparty (e.g., bank, investor) and how to price it.
The value of a real option is highest when uncertainty governing a project is highest.
Management has the power to influence the course of a project through the way they make decisions and allocate resources. So they can move the project along in a favorable way and also be willing to exercise the options on it.
The analysis of real options is a subset of corporate finance and making decisions under uncertainty.
The theory behind financial options can also extend to real options.
Purchasing managers in a company can use real options analysis to help them decide how to make certain decisions given all the known and unknown/can’t be known factors governing how they make purchasing decisions and allocate resources.
Other applications of real options analysis
Another application of real options analysis outside corporate finance and spending decisions might include whether a student joins the workforce immediately after high school or college, or gets a degree/graduate degree first (or even alongside working).
There are the costs of time, all-in educational expenses, and foregone income to consider in getting this degree and whether the extra income that comes of the extra skills procured is worth in excess of these costs. Then there are also discounting considerations – the value of earning money now versus earning money several years in the future.
Whatever the exact business circumstance, it forces those making the decisions to be clear about the assumptions they’re making that lead to their projections. Once all factors are considered, then a business strategy can be formulated on how to deal with this particular problem.
It might also involve building out support systems to deal with these decision-making needs.
Trinomial tree
Developed by Phelim Boyle in 1986, the trinomial tree is a lattice-based computational model used to value options.
The trinomial tree works off of the binomial options valuation model and is essentially the same as the explicit finite differences model discussed earlier in this article.
The trinomial method is used to produce more accurate results than the binomial model when there are fewer discrete time steps to model. So if time or other resources may be an issue, the trinomial model may be preferred.
For standard vanilla options, the binomial model is commonly implementeded because results converge when the number of time steps increases, so the method is easier to use.
The trinomial model is often used when modeling exotic options or complex options that have path dependencies that make their valuation more difficult to accurately model with the binomial or time continuous models.
Vanna-Volga pricing
Vanna-Volga pricing is used for pricing exotic options in currency derivative markets.
Vanna-Volga entails adjusting the Black-Scholes theoretical value by the cost associated with the main risks related to an option’s volatility:
- vega (sensitivity of the price of an option with respect to implied volatility)
- vanna (sensitivity of vega to a change in the spot price of the underlying)
- volga (sensitivity of vegas to a change in implied volatility)
Carr-Madan Formula
The Carr-Madan Formula is a mathematical approach used in financial mathematics to price European options.
It is named after Peter Carr and Dilip Madan, who introduced the formula as a part of their research on option pricing.
The formula is used to calculate the price of a European option by using Fourier transforms.
This can make the calculation process more efficient and faster. It’s especially true for options with complex features or for pricing a large number of options simultaneously.
Overview
The Carr-Madan method is based on the observation that the option pricing formula can be expressed in terms of the characteristic function of the logarithm of the asset price.
The characteristic function can be efficiently computed using the Fast Fourier Transform (FFT), a widely used algorithm to compute the discrete Fourier transform and its inverse.
Purpose
- Efficiency: The Carr-Madan formula allows for the efficient computation of option prices, which is particularly useful in markets where options are frequently traded and prices need to be calculated quickly.
- Flexibility: The method is flexible and can be used to price various types of European options, including those with exotic features.
- Numerical Stability: The use of Fourier transforms can enhance the numerical stability of the option pricing process, leading to more accurate and reliable prices.
Formula
The Carr-Madan formula expresses the price of a European call option as an integral involving the characteristic function of the logarithm of the asset price.
The formula is given as:
C(K) = e^-rT * (e^-dk/pi ∫ Re(e^-iu log (K) ϕ(u-i)) du)
Where:
- C(K) is the call option price with strike
- r is the risk-free interest rate
- T is the time to maturity
- d is the dividend yield
- u is a dummy variable of integration
- ϕ(u) is the characteristic function of the logarithm of the asset price
Ito’s Stochastic Calculus
Ito’s Stochastic Calculus, named after Kiyoshi Ito, is a branch of mathematics that deals with the calculus of functions of stochastic processes.
It’s particularly useful in finance for modeling the random motion of financial instruments, such as stock prices.
Key Concepts
- Brownian Motion (or Wiener Process): It’s a continuous-time stochastic process that represents the random movement of particles suspended in a fluid (or stock prices in finance). It’s characterized by having independent increments and being normally distributed with a mean of 0 and variance of t.
- Ito’s Lemma: This is a fundamental result in stochastic calculus. It provides a way to find the differential of a function of a stochastic process. In the context of option pricing, it’s used to derive the Black-Scholes equation. The lemma accounts for the quadratic variation of the Brownian motion, which is essential for capturing the randomness in stock price movements.
- Stochastic Differential Equations (SDEs): These are differential equations in which one or more of the terms is a stochastic process, resulting in a solution that is also a stochastic process. In finance, SDEs are used to model the dynamics of stock prices, interest rates, and other financial variables.
Partial Differential Equations (PDEs)
PDEs involve functions of several variables and their partial derivatives.
In the context of option pricing, PDEs are used to describe the evolution of an option’s price over time and under various stock price movements.
Key Concepts
- Black-Scholes PDE: This is the most famous PDE in finance. It describes how the price of a European option evolves over time as the underlying stock price changes. The Black-Scholes PDE can be derived using Ito’s lemma and the no-arbitrage principle. Once you have the PDE, you can solve it under appropriate boundary conditions to get the Black-Scholes formula for option pricing.
- Boundary Conditions: These are conditions that the solution to a PDE must satisfy at the boundaries of the domain of interest. For example, for a European call option, the boundary conditions might specify the option’s value at expiration and when the stock price is very high or very low.
- Numerical Methods: Often, PDEs in finance can’t be solved analytically, so numerical methods like finite difference methods or binomial/trinomial trees (discussed above) are used to approximate the solution.
Connection between SDEs and PDEs
Ito’s Stochastic Calculus provides the tools to derive the stochastic differential equations that describe the dynamics of financial instruments.
When combined with the no-arbitrage principle, these SDEs can be transformed into PDEs, which describe the evolution of option prices.
The Black-Scholes PDE is a prime example of this connection.
Quantum Options Pricing Models
Quantum models of option pricing are a relatively new and evolving area of research that seeks to leverage the principles of quantum mechanics and quantum computing to improve the efficiency and potentially the accuracy of traditional option pricing models.
Here’s a brief overview of the mentioned quantum models:
1. Quantum Continuous Model
This model draws parallels between certain equations in quantum mechanics and the Black-Scholes equation used in option pricing.
The idea is to use techniques from quantum mechanics to solve the Black-Scholes equation more efficiently.
The Schrödinger equation, which describes how the quantum state of a physical system changes over time, has mathematical similarities with the Black-Scholes equation.
By treating the option price as a quantum-like wave function, one can potentially find solutions using quantum mechanical techniques.
2. Quantum Binomial Model
This is a quantum version of the classical binomial option pricing model.
The classical binomial model breaks down the option’s life into discrete time intervals and computes the option’s price based on possible stock price movements in each interval.
In the quantum version, quantum gates and qubits (the basic units of quantum information) are used to represent and compute the binomial price tree, potentially speeding up the computation.
3. Multi-step Quantum Binomial Model
This is an extension of the quantum binomial model where multiple steps or time intervals are considered simultaneously using quantum parallelism.
Quantum computers can process a vast amount of information simultaneously due to the principle of superposition.
By leveraging this, the multi-step quantum binomial model can evaluate multiple nodes in the binomial tree at once, potentially leading to faster option pricing.
4. Quantum Algorithm for the Pricing of Derivatives
This approach involves designing specific quantum algorithms that can be run on quantum computers to price derivatives, including options.
Quantum algorithms, like Grover’s and Shor’s algorithms, have shown the potential to solve certain problems much faster than classical algorithms.
By developing quantum algorithms tailored for derivative pricing, one can potentially achieve speedups in computation time.
Note: Grover’s algorithm efficiently searches an unsorted database, while Shor’s algorithm factors large numbers, potentially breaking RSA encryption. (RSA encryption is a public-key cryptographic system that uses the mathematical properties of large prime numbers to secure data transmission and communication.)
Benefits of Quantum Models
Quantum computers, in theory, can process information at exponentially faster rates than classical computers for certain problems.
This speed can be critical for real-time pricing and risk management.
Some complex financial products or scenarios that are computationally intensive on classical computers might be more tractable on quantum computers.
It could potentially change high-frequency trading considerations.
Challenges
- Hardware Limitations: Large-scale, error-free quantum computers are still in development. The quantum models’ real-world applicability depends on advancements in quantum hardware.
- Noise and Errors: Quantum computations are susceptible to noise, which can introduce errors. Error correction and noise reduction are active areas of research.
Summary
Quantum models of option pricing are promising and theoretically intriguing.
However, their practical application hinges on advancements in quantum computing technology and the development of robust quantum algorithms.
Why Are Options Pricing Models Commonly Used in the Valuation of Early-Stage Companies?
Options pricing models, particularly the Black-Scholes model and its variants, are originally designed for pricing options in financial markets.
However, they have found application in the valuation of early-stage companies for several reasons:
Uncertainty and Volatility
Early-stage companies are characterized by a high degree of unknowns in terms of future cash flows, growth prospects, and even survival.
This uncertainty is analogous to the volatility parameter in options pricing models, making such models suitable for capturing the risk and potential upside of young firms.
Option-like Characteristics of Equity
Equity in an early-stage company can be viewed as a “call option” on the firm’s assets.
If the company does well, equity holders benefit from the upside.
If the company fails, equity holders’ losses are limited to their initial investment, similar to how a call option works.
This analogy makes options pricing models appropriate for such valuations.
Flexibility in Decision Making
Early-stage companies often have the flexibility to make strategic decisions in the future, such as expanding into new markets, pivoting their business model, or even shutting down certain operations.
These strategic decisions can be viewed as “real options.”
Options pricing models can be used to value these real options, providing a more nuanced valuation that accounts for managerial flexibility.
Employee Stock Options
Many early-stage companies compensate their employees with stock options.
To value these options and determine the associated expense for financial reporting, companies use options pricing models.
Complex Capital Structures
Early-stage companies, especially those that have gone through multiple rounds of financing, might have a complex capital structure with different classes of equity and convertible securities.
Options pricing models can help in valuing these complex securities by treating them as compound options.
Regulatory and Accounting Standards
For financial reporting purposes, especially post the issuance of the Statement of Financial Accounting Standards (SFAS) No. 123R, companies are required to expense employee stock options.
Options pricing models provide a standardized method to compute this expense.
Market Comparables Limitation
For early-stage companies, especially in novel industries, there might be a lack of comparable publicly traded companies to use for relative valuation.
In such cases, options pricing models can offer an alternative valuation approach.
Investor Expectations
Venture capitalists and other early-stage investors are often familiar with options pricing models.
Using these models in valuation can align the company’s valuation methodology with investor expectations.
Limitations
While options pricing models offer several advantages in the valuation of early-stage companies, they also come with limitations.
Assumptions about volatility, risk-free rates, and other parameters can significantly impact the valuation.
As with any valuation method, it’s important to use them judiciously and in conjunction with other valuation approaches when appropriate.
Analytical Branches in Options Pricing
Classically, there are two main analytical branches in options pricing:
- risk-neutral probability
- arbitrage-pricing probability
We’ll look at them more below.
Risk-Neutral Probability
The risk-neutral probability approach is based on the idea of adjusting the probabilities of future stock price movements so that anyone analyzing the stock would be indifferent to risk.
In a risk-neutral world, all assets are expected to grow at the risk-free rate.
Key Concepts
- Risk-Neutral Measure: This is a hypothetical probability measure under which the expected return on the underlying asset (like a stock) is the risk-free rate. It’s not the “real-world” probability but a mathematical construct used for pricing derivatives.
- Discounting at the Risk-Free Rate: In the risk-neutral framework, future payoffs from the option are discounted back to the present using the risk-free rate. (This is because, in a risk-neutral world, all investments are expected to grow at this rate.)
- Martingale Property: Under the risk-neutral measure, the price of the underlying asset, when discounted at the risk-free rate, is a martingale. This means its expected future value, when discounted back to the present, is its current value.
Application
The Black-Scholes model and the binomial option pricing model both use the risk-neutral probability approach.
In these models, options are priced by taking the expected value of their future payoffs under the risk-neutral measure and then discounting back to the present at the risk-free rate.
Arbitrage-Pricing Probability
The arbitrage-pricing approach is grounded in the principle that there should be no arbitrage opportunities in the market.
An arbitrage opportunity arises when one can make a risk-free profit without any investment.
Key Concepts
- Law of One Price: Two assets that have the same cash flows in the future should have the same price today. If they don’t, an arbitrage opportunity exists.
- Replicating Portfolio: In the context of option pricing, one can create a portfolio using the underlying asset and risk-free bonds that replicates the cash flows of the option. The price of the option should be the same as the cost of this replicating portfolio to prevent arbitrage.
- Dynamic Hedging: This involves adjusting the proportions of the underlying asset and bonds in the replicating portfolio over time to continuously match the option’s payoffs.
Application
The Black-Scholes model and the binomial option pricing model also use the arbitrage-pricing approach.
In these models, the option’s price is determined by finding the cost of a replicating portfolio that mimics the option’s payoffs.
If the option were priced differently from this replicating portfolio, arbitrageurs would step in to exploit the price difference, bringing the prices back in line.
Summary
Both the risk-neutral probability and the arbitrage-pricing probability approaches are foundational in option pricing.
They offer different perspectives but often converge to the same results in many commonly used option pricing models.
Local Volatility Models
The Black-Scholes-Merton (BSM) model has limitations, particularly in its assumption of constant volatility.
To address these limitations and better capture market realities, alternative volatility models have been developed in quantitative finance.
These models introduce more complexity into the pricing process by allowing for dynamic changes in volatility and other factors.
Two significant categories of these models are.
Concept
These models allow volatility to vary with both the price of the underlying asset and time.
This approach reflects the real-world observation that volatility is not constant but depends on factors like the asset’s price level and the time to expiration.
Dupire Model
One of the most well-known local volatility models is the Dupire model, named after Bruno Dupire.
This model uses market data, specifically the prices of different options across various strikes and maturities, to construct a volatility surface.
The volatility surface represents how implied volatility changes with both strike price and time to maturity.
Application
Local volatility models are particularly useful for pricing exotic options, where the path of the underlying asset’s price and the time-dependency of volatility are crucial.
Stochastic Volatility Models
These models, unlike the BSM model, assume that volatility itself follows a random process.
In other words, volatility is stochastic and can change unpredictably over time.
Here are a couple examples:
Heston Model
Model assumes that volatility follows a mean-reverting square-root process.
It’s characterized by parameters that control the:
- long-run average level of volatility
- speed of mean reversion, and
- volatility of volatility itself
SABR Model
The SABR (Stochastic Alpha, Beta, Rho) model is a dynamic model in which both the future volatility and the underlying asset price follow stochastic processes.
It’s widely used in interest rate derivative markets.
Application
Stochastic volatility models are better at capturing the complex behaviors of market-implied volatilities and are commonly used in interest rate, foreign exchange, and equity markets.
Both local volatility and stochastic volatility models offer a more nuanced approach to options pricing compared to the BSM model.
They are particularly effective in markets where volatility shows significant variation and patterns that are not adequately captured by the assumption of constant volatility.
These models nonetheless come with increased computational complexity and the need for sophisticated calibration techniques.
They rely heavily on market data for calibration and require more advanced mathematical and numerical methods for implementation.
But they provide a more accurate and realistic framework for pricing options.
Non-Parametric Models
Non-parametric models in options pricing offer a flexible alternative to traditional parametric models like the Black-Scholes-Merton model.
Parametric models are based on specific assumptions about the distribution and behavior of underlying asset prices and volatility.
These non-parametric approaches do not presuppose a particular functional form for the asset’s price distribution or volatility and instead derive insights directly from market data.
Some notable non-parametric methods include:
Kernel Smoothing Techniques
These techniques involve using kernel functions to estimate the probability density of underlying asset prices or the option prices themselves.
Unlike parametric models, kernel smoothing doesn’t assume a specific distribution for the data.
It’s highly adaptable, able to conform to the actual shape and structure of the market data.
This flexibility makes it valuable for capturing complex market behaviors and non-linear relationships that traditional models might miss.
Nearest Neighbor Methods
These methods estimate option prices based on the prices of similar options or underlying assets.
The idea is to find “neighbors” – data points that are close in characteristics to the option being priced – and use their prices to infer the price of the option in question.
This approach is data-driven and can be effective in markets with a rich dataset of similar instruments.
Splines and Interpolation Techniques
These techniques use spline functions – piecewise polynomial functions – to interpolate or smooth the price-yield curves or volatility surfaces.
This method can create smooth curves or surfaces that pass through or near the market data points.
This provides a more flexible fit than traditional parametric models.
Quantile Regression
This approach models the conditional quantiles of the option price distribution as a function of independent variables, such as the strike price and time to maturity.
Unlike ordinary least squares regression that focuses on the mean, quantile regression can provide a more comprehensive view of the potential outcomes – particularly in the tails of the distribution.
Artificial Neural Networks (ANNs)
ANNs are a form of machine learning that can be used to model complex, non-linear relationships in financial data.
In options pricing, ANNs learn from market data to find patterns and relationships that might be missed by traditional models.
It offers potentially more accurate pricing, particularly in markets with complex dynamics.
Summary
These non-parametric models are particularly useful in situations where the underlying asset’s market behavior is complex and does not conform to the standard assumptions of parametric models.
They offer the flexibility to adapt to different market conditions and can capture more nuanced aspects of option pricing.
Nevertheless, they often require a substantial amount of data and can be computationally intensive.
Conclusion
Options pricing models are used as methods to value options contracts.
There are several different types of options pricing models, including the Black-Scholes, the binomial options pricing model, the trinomial options pricing model, more niche applications like the Vanna-Volga pricing model, and broad applications to real-world business decisions (real options analysis).
Each of these models has its own strengths and weaknesses and is used for different sorts of markets.
The binomial options pricing model is simple and fast, but may not produce accurate results for exotic options.
The trinomial options pricing model is more accurate than the binomial options pricing model, but is also more complex and takes longer to compute.
The Vanna-Volga pricing model adjusts the Black-Scholes theoretical value by accounting for the cost of a portfolio that hedges three main risks associated with the volatility of the option.
Monte Carlo methods are used when a trader or analyst doesn’t want to be constrained by the distribution assumptions used in a standard mathematical model. However, it is typically used as a method of last resort, especially if existing models provide relatively robust estimations for options pricing.
SDEs (Stochastic Differential Equations) model random processes and are fundamental in options pricing, while PDEs (Partial Differential Equations) like the Black-Scholes equation, derived from SDEs, determine the fair market value of options.
Quantum options pricing models leverage the computational power of quantum mechanics to solve complex financial equations faster and more accurately than classical methods.
This enhanced speed and precision can better capture intricate market dynamics, potentially offering traders a more accurate valuation of options and a competitive edge in the market.