Theoretical Finance (Concepts)
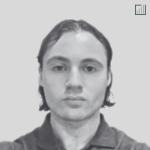
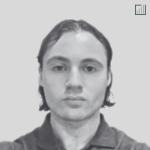
Theoretical finance forms the backbone of our understanding of how markets operate.
It integrates mathematical models and economic theory to predict, explain, and understand the behaviors of financial markets.
It includes a range of topics including asset pricing, risk management, market microstructure, and corporate finance.
Key Takeaways – Theoretical Finance
- Foundation of Market Understanding: Theoretical finance integrates economic and financial theory with mathematical models.
- Used for predicting and explaining market behavior, encompassing topics from asset pricing to corporate finance.
- Risk and Return Quantification: It introduces frameworks like CAPM and MPT for asset valuation and portfolio diversification.
- Employs risk management tools like VaR and stochastic processes to measure and mitigate potential losses.
- Theoretical Models vs. Market Realities: While theoretical finance provides a structured approach to understanding markets, real-world deviations such as market inefficiencies, behavioral biases, and external shocks highlight the gap between theory and practice.
Asset Pricing Models
Asset pricing is the backbone of theoretical finance, dealing with the valuation of financial securities.
The Capital Asset Pricing Model (CAPM) and the Arbitrage Pricing Theory (APT) serve as foundational theories.
CAPM describes the relationship between systematic risk and expected return, while APT assumes multiple risk factors influence an asset’s return.
Portfolio Theory
Modern Portfolio Theory (MPT), introduced by Harry Markowitz in 1952, changed the process of investment decision-making.
It quantifies the balance between risk and return in a portfolio of assets, promoting diversification to minimize unsystematic risk.
MPT’s mean-variance optimization is a staple in financial analysis (maximizing reward per unit of risk).
Risk Management
Risk management within theoretical finance involves the strategies and instruments designed to understand and mitigate risk.
Value at Risk (VaR) and Conditional Value at Risk (CVaR) are key risk measurement techniques.
These methodologies estimate the potential loss in value of a portfolio under normal and extreme market conditions.
Stochastic processes are also used for quantifying risks over time.
They allow for the modeling of various sources of uncertainty, such as market risk, credit risk, and operational risk, and help in the calculation of VaR and its derivatives.
Market Microstructure
Market microstructure examines the mechanisms through which securities are traded.
It focuses on how trades are executed and how they affect prices.
It looks at the roles of liquidity, transaction costs, and information asymmetry.
Theories within market microstructure are important in designing and regulating trading protocols, as well as algorithm development.
Corporate Finance Theory
The theoretical foundations of corporate finance involve concepts such as the Modigliani-Miller theorem, which talks about the effects of capital structure on a company’s value.
The theorem posits the irrelevance of debt versus equity financing *in perfect markets*.
However, incorporating real-world elements like taxes and bankruptcy costs adjusts this perspective.
Theory is often divorced from real-world limitations (e.g., liquidity, transaction costs, taxes, liberalized capital accounts, etc.).
Behavioral Finance
Challenging the assumption of rational market participants, behavioral finance introduces psychology-based theories to explain anomalies in financial markets.
Prospect theory and mental accounting are examples that demonstrate how cognitive biases can lead to irrational financial decisions, impacting market outcomes.
Related
Partial Differential Equations in Theoretical Finance
Partial differential equations (PDEs) are used in the modeling and analysis of financial markets.
These equations help in describing the evolution of prices and the valuation of financial derivatives.
Options Pricing and the Black-Scholes Model
The Black-Scholes model, a cornerstone of modern financial theory, uses a PDE to price European-style options.
The Black-Scholes PDE demonstrates how the price of an option evolves over time.
It considers factors like the underlying asset’s price, the option’s strike price, time to expiration, risk-free interest rate, and the asset’s volatility.
Interest Rate Models
Interest rate models, like the Heath-Jarrow-Morton (HJM) framework and the Black-Derman-Toy (BDT) model, often employ PDEs to predict future movements of interest rates and to value interest rate derivatives.
PDEs capture the dynamics of interest rates, including their term structure and risk factors.
Portfolio Optimization
In portfolio optimization, PDEs are used to solve dynamic programming problems.
They provide the necessary mathematical framework to determine optimal portfolio policies over continuous time.
Stochastic Processes in Theoretical Finance
Stochastic processes are used in theoretical finance to model the randomness inherent in financial markets.
These mathematical objects represent variables that evolve over time in a probabilistic manner.
Modeling Asset Prices
Stochastic processes, like the geometric Brownian motion, are employed to model stock prices and other financial assets.
The geometric Brownian motion captures the continuous-time random walk of asset prices.
As such, it accommodates the unpredictability and the so-called randomness observed in market prices.
Interest Rate and Credit Models
Models such as the Cox-Ingersoll-Ross (CIR) model for interest rates and the Jarrow-Turnbull model for credit risk use stochastic calculus to reflect the random nature of interest rate changes and credit defaults.
These models are useful for valuing bonds, interest rate swaps, and credit derivatives.
Stochastic Differential Equations (SDEs)
Many models in finance, including the Black-Scholes and the HJM framework, are based on SDEs, which are a type of stochastic process.
These equations take into account both the deterministic, cause-effect trends and the “random shocks” (known unknowns and unknown unknowns) affecting financial variables.
Example of Theory Before Practical Application – Tensor Analysis
In other articles, we explored topics like statistical physics, econophysics, quantum economics, and quantum finance – all things that originated in mathematics and physics that could potentially be applied to economics and markets in the appropriate circumstances.
Consider the example of tensors.
A tensor in mathematics is a generalization of scalars, vectors, and matrices.
It’s a mathematical object that can be used to represent data with many variables.
Just like:
- a scalar is a single number
- a vector is a list of numbers, and
- a matrix is a grid of numbers…
- …a tensor can be thought of as a multi-dimensional array of numbers
Each number in this array is identified by several indices instead of just one or two.
This allows tensors to describe more complex relationships between sets of numbers in higher dimensions.
Analogously, a tensor is like a higher-dimensional version of a grid that can store and organize data across multiple dimensions, beyond just rows and columns.
So it helps in solving complex problems that involve many different factors and relationships.
Tensor analysis can be used in finance. But it’s much less common than in the field of general relativity in physics.
In finance, tensor analysis might theoretically be applied in the following ways:
Risk and Return Models
Tensors can represent multi-dimensional data sets, which are prevalent in finance.
For instance, a tensor could model the returns of a set of assets over time under various economic conditions.
High-Dimensional Financial Derivatives Pricing
More complex financial instruments, like collateralized debt obligations (CDOs), might benefit from tensor-based models.
Tensors could theoretically handle the multidimensional aspects of these products, such as layered repayments and tranches.
Machine Learning and Data Mining
Tensor decomposition methods like CANDECOMP/PARAFAC (CP) and Tucker decomposition can analyze multi-way financial data.
It’s a form of principal component analysis (PCA), commonly used in risk analysis and portfolio construction.
This can be particularly useful in identifying patterns and anomalies in high-dimensional datasets.
For instance, we’ve used the example that PCA can help a trader understand that X% of the variance in his/her portfolio can be attributed to changes in:
- discounted growth
- discounted inflation
- risk premiums, and
- discount rates
This can lead to insights for algorithmic trading strategies.
Related
Portfolio Optimization
Tensor calculus can be used to generalize mean-variance portfolio optimization to higher moments.
“Higher moments” just means other factors outside mean and variance.
Examples commonly include capturing the skewness and kurtosis of asset returns (i.e., the shape of the distribution of returns).
Higher skewness means a distributed skewed toward higher positive returns and less toward negative returns (a good thing).
Higher kurtosis means a fatter-tailed distribution than what a normal distribution might predict. This might mean a trader might choose to hedge with OTM options.
Skewness and kurtosis are popularly reported data to help understand how portfolios performed in the past, as we did with these popular strategies:
- Harry Browne Permanent Portfolio
- Golden Butterfly Portfolio
- Yale Portfolio (David Swensen Lazy Portfolio)
- Paul Merriman Ultimate Buy & Hold Portfolio
- Gone Fishing Portfolio
- 3-Fund Bogleheads Portfolio
- Scott Burns Couch Potato Portfolio
Stochastic Processes Modeling
In modeling complex financial systems, tensors can represent the state of a stochastic process at different points in time and under different scenarios.
This can provide a comprehensive view of potential outcomes.
Multi-factor Financial Models
In these models, tensors can be used to represent the interactions between multiple factors that affect asset prices.
This can provide a more nuanced view of market dynamics.
Summary
While the use of tensor analysis in finance is less intuitive and more abstract than its application in physics, it offers a potential tool for dealing with the complexity of financial systems.
Especially when traditional matrix algebra becomes insufficient for capturing the full scope of multi-dimensional relationships and dynamics.
Nonetheless, the complexity of tensors means that they’ll be reserved for more advanced financial modeling tasks.
Applications and Limitations of Theoretical Finance
The application of theoretical finance extends to various domains from guiding investment strategies to shaping regulatory policies.
However, limitations arise due to the simplifying assumptions necessary for mathematical tractability and the dynamic nature of financial markets.
So, real-world results may deviate from theoretical predictions.