Quantum Economics – Applications in Trading
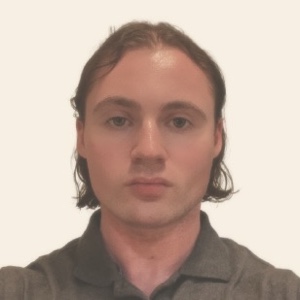
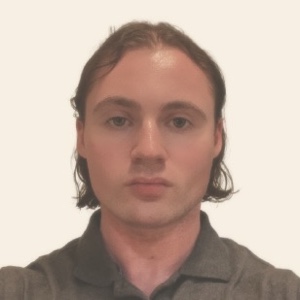
Quantum economics is an emerging research field that applies mathematical methods and ideas from quantum physics to the field of economics.
It is motivated by the belief that economic processes such as financial transactions have much in common with quantum processes, and can be appropriately modeled using quantum formalism.
It draws on techniques from the related areas of quantum finance and quantum cognition, and is a sub-field of quantum social science.
Key Takeaways: Quantum Economics – Applications in Trading
- Quantum economics, rooted in quantum physics’ mathematical techniques, represents a developing branch within the wider sphere of quantum social science, exploring economic phenomena through a quantum lens.
- Quantum economics deviates from the principles of neoclassical economics, questioning the foundational assumptions of expected utility theory, and exploring the nature of decision-making processes from a quantum perspective.
- It portrays price fluctuations as a result of fundamental uncertainty. It could potentially better provide a framework for modeling complex dependencies that are found in economies and markets.
- This contrasts with the efficient market theory, and highlights the interconnected nature of financial actors, which is represented in quantum game theory.
- Quantum economics can look at the active role of financial transactions and the interlinkages formed through credit systems (e.g., debtors being tied to creditors), a new approach with different foundational assumptions compared to neoclassical economics.
- Quantum trading seeks to integrate quantum mechanics’ principles and techniques into finance and trading. This provides a new approach to portfolio optimization and predicting market movements with high computational efficiency.
History of Quantum Economics
A number of economists including Paul Samuelson and Bernard Schmitt have found inspiration in quantum theory.
Asghar Qadir
Perhaps the first to directly exploit quantum techniques in economic analysis was the Pakistani mathematician Asghar Qadir.
In his 1978 paper Quantum Economics, he argued that the formalism of quantum mechanics is the best mathematical framework for modeling situations where “consumer behavior depends on infinitely many factors and that the consumer is not aware of any preference until the matter is brought up.”
He proposed that, like particles in quantum mechanics, “the individual as an entity … can be thought of as a point in a Hilbert space.”
Qadir’s paper received little attention.
However, during the 1990s, workers in the field of quantum cognition showed that many aspects of human decision-making, including those involved in economic decisions, seemed to follow a kind of quantum logic.
At the same time, researchers such as economist Martin Shubik, physicist Martin Schaden, and social scientist Emmanuel Haven were beginning to use quantum formalism to model stock markets.
Belal E. Baaquie
In his 2007 book Quantum Finance: Path Integrals and Hamiltonians for Options and Interest Rates, Belal E. Baaquie showed how methods from quantum physics could be applied to things like the pricing of financial options.
However, he wrote that the “larger question of applying the formalism and insights of (quantum) physics to economics, and which forms a part of the larger subject of econophysics, is left for future research.”
In a 2010 review, Schaden noted that quantum techniques could only be justified if the financial system could be shown to exhibit quantum properties such as entanglement, and if their use led to genuine simplifications over existing stochastic approaches.
In their 2013 book Quantum Social Science, Emmanuel Haven and Andrei Khrennikov extended Baaquie’s work in finance to questions such as arbitrage and the reflexivity theory of George Soros.
David Orrell
In a 2016 paper and book (the latter co-authored with journalist Roman Chlupatý), the mathematician David Orrell proposed a quantum theory of money and value.
This theory states that money has dualistic, quantum properties because of the way that it merges the exact concept of number with the fuzzy concept of value, and the use of money leads to mental and financial entanglement which can be modeled using quantum methods.
In his 2018 book Quantum Economics: The New Science of Money and other works, he described quantum economics, which combined this view of money with the insights of quantum finance and quantum social science.
Andrew Sheng
In a 2019 article for the Bretton Woods Committee, Andrew Sheng wrote that “A quantum paradigm of finance and the economy is slowly emerging, and its nonlinear, complex nature may help the design of a future global economy and financial architecture … Financial assets and virtual liabilities have quantum characteristics of entanglement with each other that are not yet fully understood.”
Descriptive Differences from Neoclassical Economics
Just as quantum physics differs in fundamental ways from classical physics, quantum economics differs from neoclassical economics in a number of key respects.
Expected Utility Theory vs. Quantum Interpretations
Neoclassical economics is based on expected utility theory, which combines utility theory to model people’s preferences, and probability theory to model expectations under non-deterministic outcomes.
However, the field of quantum cognition calls these assumptions into question, since people don’t necessarily have fixed preferences, or base their decisions on probability theory.
Many of the findings of behavioral economics are inconsistent with classical logic, but agree with quantum decision theory of the sort assumed in quantum social science.
How Prices Are Formed – Classic Economics vs. Quantum Framework
In financial applications, neoclassical economics is associated with efficient market theory, where price is assumed to reflect intrinsic value with a degree of change due to the pricing in of new information.
In quantum economics, price fluctuations are seen as being due to fundamental uncertainty.
How Financial Decisions Are Made – Classic Economics vs. Quantum Framework
Neoclassical economics assumes that people act independently while making economic decisions.
Quantum economics notes that financial actors are part of an entangled system, as in quantum game theory.
Quantum economics also stresses the importance of financial transactions and in particular the role of credit as an active force in the economy, for example in the way that it entangles debtors and creditors through loans.
Quantum economics can therefore be viewed as an alternative to neoclassical economics which begins from a different set of assumptions.
Real-World Quantum Trading Examples
Quantitative trading is a widespread component of financial markets, especially given the high-speed calculation requirements.
Despite its significance, quantum algorithms hadn’t been integrated into this domain until recently.
A study titled “Quantum Quantitative Trading: High-Frequency Statistical Arbitrage Algorithm” by Xi-Ning Zhuang, Zhao-Yun Chen, Yu-Chun Wu, and Guo-Ping Guo looks into this topic.
Key Points
- The study introduces quantum algorithms for high-frequency statistical arbitrage trading.
- These algorithms leverage variable time condition number estimation and quantum linear regression.
- The complexity of the algorithm has been reduced significantly from the classical benchmark O(N^2d) to O(sqrt(d)(kappa)^2(log(1/epsilon))^2).
- Here, N represents the length of trading data, d is the number of stocks, kappa is the condition number, and epsilon denotes the desired precision.
- The research showcases the potential quantum advantage in trading.
- Additionally, the study also develops two tool algorithms for condition number estimation and a cointegration test.
Implications
This research indicates that quantum algorithms can offer a competitive edge in high-frequency trading by reducing computational complexity and enhancing speed.
The introduction of such algorithms could change the way trading strategies are formulated and executed in the financial markets.
How Is Quantum Trading Implemented?
Quantum trading is a relatively new concept that seeks to apply principles and techniques from quantum mechanics to the world of finance and trading.
While the full integration of quantum mechanics into trading is still new, there are several ways in which quantum principles are being implemented in the trading world:
Quantum Algorithms for Portfolio Optimization
One of the primary applications of quantum computing in trading is portfolio optimization.
Traditional methods of portfolio optimization can be computationally intensive, especially when dealing with a large number of assets.
Quantum algorithms, such as the Quantum Approximate Optimization Algorithm (QAOA), can potentially solve these problems more efficiently than classical computers.
Predicting Financial Market Movements
Quantum computers have the potential to process vast amounts of data at unprecedented speeds.
This capability can be used to analyze historical market data and identify patterns that might be too complex for classical computers to detect.
By doing so, traders can gain insights into potential future market movements and make better trading decisions.
Quantum Encryption in Trading
Security is a paramount concern in trading – markets can be theoretically hacked – especially with the rise of high-frequency trading and the need for ultra-fast, secure communication channels.
Quantum encryption, based on the principles of quantum mechanics, could offer potential advances in security.
Modeling Financial Instruments Using Quantum Mechanics
Some financial instruments, especially derivatives, can be complex and difficult to price using classical methods.
Quantum mechanics, with its ability to model complex systems, can be used to develop more accurate pricing models for these instruments.
Quantum Machine Learning for Trading
Machine learning, especially deep learning, has already made inroads in trading.
Quantum machine learning, which combines quantum computing with machine learning techniques, can potentially process and analyze data at a much faster rate than classical machine learning algorithms.
This can be especially useful in trading, where milliseconds can make a difference in terms of profit and loss.
Challenges and Limitations
While the potential of quantum trading is high, the field is still in its early stages.
Quantum computers capable of outperforming classical computers in real-world applications, known as “quantum supremacy,” are still in development.
Moreover, the integration of quantum principles into trading also requires a deep understanding of both quantum mechanics and financial markets, making it a highly specialized field.
Quantum Cognition
Quantum cognition is a field that applies principles of quantum theory to understand cognitive processes and human decision-making.
It explores how people perceive and respond to ambiguous information or conflicting beliefs, often demonstrating behavior that defies classical probability theory but aligns with quantum probability.
This approach enables a more nuanced and potentially accurate depiction of complex cognitive phenomena compared to classical models.
Quantum Cognition’s Application to Trading
Quantum cognition can assist in modeling the financial markets more accurately by considering the “superposition” of multiple market states, enhancing prediction accuracy.
Traders might use these principles to develop more sophisticated strategies that factor in the inherent unknowns and complex dependencies (“probabilities of probabilities”) found in financial markets.
This can potentially lead to more profitable decision-making and risk management in trading.
It encourages thinking beyond classical probability theories (e.g., basic expected value), exploring new avenues for predicting market behavior and trends.
FAQs: Quantum Economics – Applications in Trading
What is Quantum Economics?
Quantum economics is a budding research domain that employs mathematical techniques and concepts from quantum physics to economics.
It’s driven by the idea that economic activities, like financial transactions, share similarities with quantum processes and can be accurately represented using quantum formalism.
This field is an offshoot of quantum social science and incorporates methods from quantum finance and quantum cognition.
How did Quantum Economics originate?
Historically, several economists, including Paul Samuelson and Bernard Schmitt, drew inspiration from quantum theory.
However, Asghar Qadir, a Pakistani mathematician, was perhaps the first to directly apply quantum techniques to economic analysis in his 1978 paper titled “Quantum Economics.”
Although Qadir’s work initially garnered minimal attention, the 1990s saw a surge in interest as researchers began to observe quantum logic in human decision-making, especially in economic choices.
How does Quantum Economics differ from Neoclassical Economics?
While neoclassical economics is grounded in expected utility theory, which merges utility theory and probability theory, quantum economics challenges these assumptions.
Quantum cognition suggests that individuals don’t always have set preferences or base their decisions on probability theory.
Unlike neoclassical economics, which assumes individuals act independently, quantum economics posits that financial actors are part of an interconnected system, akin to quantum game theory.
Additionally, quantum economics emphasizes the active role of money and credit in the economy, particularly in how it links debtors and creditors.
What are the applications of Quantum Economics in Trading?
In trading, quantum economics offers a fresh perspective on price fluctuations, viewing them as results of fundamental uncertainty rather than just noise from new information, as suggested by efficient market theory in neoclassical economics.
Quantum economics also provides insights into financial entanglement, which can be useful for understanding market dynamics and developing trading strategies.
How does Quantum Economics explain financial entanglement?
Quantum economics suggests that financial actors, such as traders, investors, and institutions, are part of an interconnected system.
This means that the actions of one actor can influence others, similar to the concept of entanglement in quantum physics.
For instance, the way credit links debtors and creditors through loans is a form of financial entanglement.
How does Quantum Economics address behavioral economics findings?
Many findings in behavioral economics contradict classical logic.
However, they align with the quantum decision theory assumed in quantum social science.
This means that quantum economics might offer a more accurate representation of human decision-making processes in economic scenarios.
Is Quantum Economics widely accepted in the financial world?
Quantum economics is still an emerging field, and while it offers promising insights and alternative perspectives, it’s yet to gain widespread acceptance.
However, as research progresses and more applications are discovered, it’s likely that its influence in the financial world will grow.