‘Probabilities of Probabilities’ in Finance
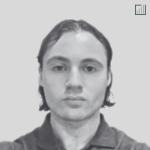
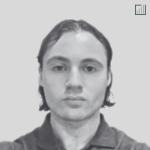
“Probabilities of probabilities,” often referred to as uncertainty of uncertain events or stochastic uncertainty, is a fascinating concept in finance and markets.
Trading and investing is fundamentally an exercise of applied probability, and we have probability distributions to represent this process.
However, the probabilities themselves are often unknown.
It recognizes the multi-dimensional and nested layers of uncertainty.
There’s what you know, what you know you don’t know, and what you don’t even know what you don’t know.
In markets, the range of knowns is low relative to the range of unknowns relative to what’s discounted in the price.
In such a scenario, you’re dealing with a probability distribution where the probabilities themselves are subject to uncertainty, and things are dependent on other things.
This is a step beyond the usual probabilistic models where probabilities are assumed to be known or can be estimated directly.
And not only that, there’s the element of how these probabilities, k-th order probabilities, distributions, and multi-order probability structures evolve over time.
Ultimately, when it comes to alpha bets in the markets, it involves betting on a certain distribution relative to the one that’s priced in.
In this higher-order structure, the probabilities are essentially random or semi-random variables themselves (depending on the range of knowns and unknowns).
This creates a layered or hierarchical model of uncertainty.
Key Takeaways – Probabilities of Probabilities in Finance
- Probabilities themselves are often unknown in financial markets.
- Applying these concepts to financial models allows for a more nuanced understanding of risk and uncertainty.
- Example: In portfolio optimization, instead of working with a single expected return (e.g., stocks yield 6% per year), you could consider a distribution of expected returns, each with its own probability.
- This approach can lead to better trading/investment strategies that account for the uncertainty in the model itself.
- Aligns with the broader theme in quantitative finance of seeking deeper insights into the behavior of markets and investments – beyond the traditional models that assume certainty or static probability distributions.
- Example: Agent-based modeling provides a framework for simulating and understanding the diverse and adaptive behaviors of market participants in response to economic changes, and this can be modeled as a stochastic environment that fits higher-order probability models.
Overview
Here’s a breakdown:
Probabilities Themselves Are Often Unknown
In finance, we often estimate probabilities of events like stock prices rising or falling.
However, these probabilities themselves are not fixed constants, but rather can be influenced by other uncertain/unknown factors.
Example
Imagine predicting the probability of a company exceeding or falling short of revenue expectations.
This probability might depend on the fluctuations in various countries’ business cycles, political climate, economic indicators, and countless other variables.
Each of these variables depends on has its own probability of unfolding in particular ways, which adds another layer of unknowns.
Short-Run vs. Long-Run
We often hear that stocks yield a certain amount per year or bonds yield X%.
Nonetheless, over the short-term there’s much more variance to those figures.
Over the long run, you might expect stocks to yield in line with the nominal growth rate of the economy.
But even then, over longer periods, you see disparities between the cash flows being generated in the real economy versus those in financial markets (e.g., due to the influence of interest rates and other factors).
Mathematical Areas
Modeling distributions where you are dealing with “probabilities of probabilities” or higher-order probability structures is a complex task.
It typically involves several mathematical concepts and techniques – particularly when dealing with high dimensionality.
The following areas of mathematics can be used in addressing these challenges:
Bayesian Statistics
This is a natural framework for dealing with probabilities of probabilities.
In Bayesian inference, prior probability distributions are updated with new data to form posterior distributions.
This process inherently deals with distributions of distributions, and k-th order distributions of whatever you’re attempting to model.
Related: Bayesian Efficiency
Measure Theory
This branch of mathematics provides a foundation for probability theory, most useful when dealing with complex, high-dimensional probability spaces.
Measure theory is key to defining and understanding probability distributions in such spaces.
Stochastic Processes
When dealing with high-dimensional data over time, stochastic processes, especially Markov processes and Martingales, are essential.
These processes help in modeling the evolution of probabilities over time.
Multivariate Statistics
This field deals with the analysis of data that involves multiple variables, which is essential when dealing with high-dimensional probability distributions.
Techniques like principal component analysis (PCA) can be used to reduce dimensionality.
Information Theory
This field provides tools for quantifying information content and uncertainty in probability distributions, which is useful in the context of probabilities of probabilities.
Differential Geometry
Differential geometry helps understand the curvature and topology of data.
This is important in analyzing complex, high-dimensional probability structures where each point represents a different probability distribution.
Information Geometry
Information geometry applies the principles of differential geometry to the space of probability distributions.
It provides a structured way to measure the distance and divergence between different distributions.
Computational Techniques
Given the complexity and high dimensionality, numerical methods and simulation-based approaches like Monte Carlo methods are often necessary for practical computation and estimation.
Graphical Models
These include Bayesian networks and Markov Random Fields.
These provide a way to visually and mathematically represent complex relationships between a large number of random variables.
Functional Analysis
In some advanced applications, concepts from functional analysis might be used, especially when dealing with infinite-dimensional spaces, as in the case of random functions or fields.
Algebraic Topology
In some research and highly specialized forms of financial engineering or quantitative trading, methods from algebraic topology are used to understand the shape of high-dimensional data.
Summary
In a financial context, these mathematical tools are applied to model complex systems, such as the behavior of financial markets, risk assessment, and decision-making processes under uncertainty.
For instance, Bayesian methods can be used for updating beliefs about market trends or the performance of financial instruments in light of new data.
Computational techniques are particularly important in finance due to the often intractable nature of analytical solutions in high-dimensional settings.
Example of ‘Probabilities of Probabilities’ in Agent-Based Models
Agent-based modeling (ABM) in finance involves simulating the interactions of multiple agents (such as traders/investors, consumers, firms, governments) to understand the dynamics of financial markets.
Each agent in such models is programmed with specific rules governing their behavior and decisions.
But these actions aren’t known for sure in most cases. You have to estimate and most things are dependent on other things.
In the context of modeling higher-order probability structures, ABM can be used to simulate how each participant reacts to changes in key macroeconomic factors like, for example, discounted growth, inflation, risk premiums, interest rates + risk premiums (discount rates).
Here’s a breakdown of how this can be structured:
Agents and Their Beliefs
Agents
Participants in the model, each with their own set of beliefs and decision-making rules.
These agents could represent different types of traders/investors (retail, institutional), companies, or governments.
Beliefs
Each agent has beliefs about future economic conditions (growth, inflation), which are represented as probability distributions.
These beliefs are the first-order probabilities.
Higher-Order Probability Structures
Reaction to Economic Indicators
Agents update their beliefs (probabilities) based on new information about economic indicators.
This update mechanism can be modeled using Bayesian methods, where prior beliefs are updated with new data to form posterior beliefs.
Modeling Interactions
Agents interact with each other and the market.
They’re influenced and are being influenced by the actions and beliefs of other agents.
These interactions create complex, higher-order probability structures as the beliefs and actions of one agent affect the probabilities associated with another agent’s beliefs and actions (and correlation structures within markets and so on).
Moreover, agents/entities learn more over time and this changes the course of future data.
Key Economic Factors in a Macro-Based ABM Model
Discounted Growth
Agents form expectations about future economic growth. This affects their trading/investment decisions.
For instance, an agent might expect high growth (relative to what’s discounted) and invest more in stocks.
Another might expect the opposite and lean more toward lower-risk fixed-income securities.
Discounted Inflation
Expectations about future inflation can influence agents’ decisions on interest rate-sensitive instruments (which is basically everything).
Discount Rates
Agents have beliefs about future interest rates, which impact their discount rates for valuing assets.
All assets compete with each other.
So if cash becomes more competitive with bonds and stocks, that impacts the relative appetite for them – a residual known as the risk premium.
Risk Premiums
Agents assess the risk associated with different assets and require premiums accordingly.
For example, an entity might require 10-year Treasury bonds to yield 2% more than cash to allocate equally between cash and 10Y Treasuries.
That same entity might require stocks to yield an extra 2.5% over 10-year Treasuries to allocate equally to stocks as those types of bonds.
So, you would say their risk premium between stocks and cash is 4.5% by extension, adding the two together.
Dynamics of the Model
Feedback Loops
The market’s reaction to agents’ collective actions can further influence individual agents’ beliefs and actions, creating feedback loops.
Market Sentiment and Behavioral Factors
Agents’ decisions are influenced not just by rational expectations but also by behavioral factors like herd behavior, overreaction, or underreaction to news.
Modeling Approach
Initialization
Define the initial state of the market, including the initial beliefs and characteristics of each agent.
For probabilities of probabilities type simulations, stochastic modeling will be involved in some way, which also involves the time evolution element of the analysis.
Rules and Algorithms
Implement algorithms for how agents update their beliefs and make decisions based on changes in economic factors.
Simulation
Run on real-world data (backtesting).
Applications in Finance
Risk Management
Understanding how markets might react under different economic scenarios.
Strategic Planning
Firms and traders/investors can use insights from these models for strategic asset allocation.
Policy Analysis
Regulators can use ABMs to assess the potential impact of policy changes on market dynamics.
Challenges
Complexity
ABMs can become extremely complex and computationally demanding.
There are lots of different market participants with all sorts of different motivations.
Calibration and Validation
Ensuring that the model accurately reflects real-world behavior is challenging.
Sensitivity to Assumptions
The outcomes of ABMs can be highly sensitive to the rules and parameters set for agent behavior.
This becomes even more complex as stochastic processes and multi-order probability concepts are involved.