Neural Network
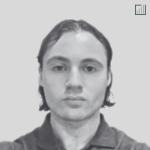
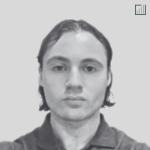
A neural network is a type of machine learning algorithm modeled after the structure and function of the human brain.
It is composed of interconnected nodes, called artificial neurons, that process information and make predictions or decisions.
Neural networks are trained using a dataset, where the network adjusts the strengths of the connections between neurons, called weights, to produce the desired output.
Neural networks can be used for a variety of tasks, such as image recognition, natural language processing, and prediction.
They are particularly useful for identifying patterns and relationships in complex data, and can improve their performance over time as they are exposed to more data.
They have garnered significant popularity in trading and investing contexts.
Key Takeaways – Neural Network
- A neural network is a computer algorithm inspired by the structure and function of the human brain, designed to recognize patterns and make decisions based on data.
- Types of Neural Networks Used in Quant Trading
- Feedforward Neural Networks (FNNs)
- Convolutional Neural Networks (CNNs)
- Recurrent Neural Networks (RNNs)
- Long Short-Term Memory Networks (LSTMs)
- Gated Recurrent Unit Networks (GRUs)
- Probabilistic Neural Networks (PNNs)
- Generative Adversarial Networks (GANs)
- Autoencoders
- Variational Autoencoders (VAEs)
- Radial Basis Function Networks (RBFNs)
- Siamese Networks
- Transformer Networks
- Capsule Networks
- Reinforcement Learning Networks
- Self-Organizing Maps (SOMs)
- Algorithmic Trading
- Neural networks, like recurrent structures like LSTM, can capture time-related dependencies in market data.
- Offers traders algorithms that can predict future price movements more accurately.
- Risk Assessment
- By analyzing vast datasets, neural networks can uncover subtle patterns, correlations, and non-linear elements that traditional models might miss.
- Portfolio Optimization
- The application of neural networks in portfolio management allows for dynamic asset allocation based on predictive analytics.
Neural Networks in Trading
Neural networks can play a variety of roles in trading, including:
Predictive modeling
Neural networks can be used to analyze historical market data and make predictions about future market movements.
This can be used for tasks such as predicting stock prices or identifying trends in currency exchange rates.
Algorithmic trading
Neural networks can be used to develop automated trading systems, which can make decisions and execute trades based on market data and the output of the neural network.
Risk management
Neural networks can be used to analyze market data and assess the risk of different trades or trading/investment strategies.
This can help traders and investors to make more informed decisions about how to manage their portfolios.
Sentiment analysis
Neural networks can be used to process large amounts of text data, such as news articles or social media posts, to identify the sentiment or tone of the text.
This can be used to gauge market sentiment and make trades accordingly.
Portfolio optimization
Neural networks can be used to optimize a portfolio of assets according to specific criteria such as minimizing volatility, maximizing returns, or minimizing risk.
Basics of Building a Neural Network
Building a neural network involves several steps, including designing the architecture, selecting the appropriate training data, and adjusting the parameters of the network to optimize its performance.
Designing the architecture
This involves deciding on the number of layers and the number of neurons in each layer that the neural network will have.
The number of layers and the number of neurons in each layer will depend on the complexity of the task that the neural network is being used for.
A deeper network with more layers and neurons will be able to model more complex relationships in the data, but it will also require more computational resources and will be more difficult to train.
Selecting the training data
The neural network needs to be trained on a dataset of examples that are representative of the task it will be used for.
For example, if the neural network is being used for image recognition, the training data should be a set of images labeled with their corresponding class.
The training data should be large enough to provide the network with enough examples to learn from, but not so large that it becomes computationally infeasible to train the network.
Training the network
The neural network is trained using a process called backpropagation, which involves adjusting the weights of the connections between the neurons to minimize the difference between the network’s predictions and the actual labels of the training data.
This is done using an optimization algorithm, such as stochastic gradient descent (SGD).
Adjusting the parameters
Once the network is trained, it can be fine-tuned by adjusting the parameters such as the learning rate, momentum, batch size, and the number of epochs to improve its performance.
Testing the network
The final step is to test the neural network on a new, unseen dataset to evaluate its performance.
The accuracy and loss of the network can be used to evaluate the performance of the network.
Building a neural network requires a good understanding of the data and the task. Moreover, it requires a good understanding of the mathematical concepts behind neural networks.
It’s an iterative process that can require several attempts to get a good model, and the final model may not be the best one possible but one that is good enough.
Features of a Neural Network
A neural network is a type of machine learning model that is designed to mimic the structure and function of the human brain. It is composed of layers of interconnected “neurons,” which process and transmit information.
There are several key features of a neural network:
Layers
Neural networks are composed of layers of interconnected neurons.
These layers are stacked on top of each other, with the input layer receiving the initial data, and the output layer producing the final output.
Weights and biases
Each neuron in a neural network has a set of weights and biases associated with it.
These values are learned during training and are used to adjust the output of the neuron.
Activation functions
An activation function is applied to the output of each neuron in order to introduce non-linearity into the network.
This allows the neural network to model more complex patterns and relationships in the data.
Backpropagation
Backpropagation is the process by which the neural network learns from the data.
It involves adjusting the weights and biases of the neurons based on the errors made during the previous iteration.
Gradient descent
Gradient descent is an optimization algorithm used to adjust the weights and biases of the neurons in order to minimize the error between the network’s output and the desired output.
Overfitting
Overfitting is a phenomenon that occurs when a neural network is trained for too long, or when it has too many layers and neurons.
This can cause the network to become too specialized for the training data and perform poorly on new, unseen data.
Overfitting is a problem in financial market modeling because there are events that can happen (e.g., droughts, wars, revolutions, civil wars, and other uncommon events) that may occur that are unlike the events that make up the data the model is trained on.
Regularization
Regularization is a technique used to prevent overfitting in neural networks.
Some popular regularization methods include dropout, L1, and L2 regularization.
Types of Neural Networks
Feedforward Neural Networks (FNNs)
FNNs are the simplest type of neural network, where information moves in only one direction, from input to output.
In finance, they’re used for credit scoring by analyzing borrower data to predict creditworthiness.
Convolutional Neural Networks (CNNs)
CNNs are designed to process data that come in the form of multiple arrays (it’s most common for images).
In financial markets, they analyze stock charts to identify patterns that precede significant price movements.
Recurrent Neural Networks (RNNs)
RNNs can use their internal state (memory) to process sequences of inputs, which makes them ideal for analyzing time-series data (like stock prices) to predict future market trends.
Long Short-Term Memory Networks (LSTMs)
A type of RNN capable of learning long-term dependencies.
LSTMs are used in quantitative trading to model and predict sequences of market data over long periods.
Most common for time series data.
Gated Recurrent Unit Networks (GRUs)
GRUs simplify the LSTM architecture and are used similarly for time-series analysis in finance, such as predicting economic or market data, or asset prices.
Probabilistic Neural Networks (PNNs)
PNNs are used for classification tasks in finance, like identifying which assets will rise or fall based on the inputs from agent-based models, by estimating the probability of different outcomes.
Generative Adversarial Networks (GANs)
GANs generate new data similar to existing data.
In finance, they’re used to create synthetic financial datasets for testing trading algorithms without risking actual capital.
Autoencoders
Autoencoders are unsupervised neural networks used for dimensionality reduction and feature learning.
An application would be in fraud detection by encoding and decoding financial transactions to identify anomalies.
Variational Autoencoders (VAEs)
VAEs are used for generating new data samples.
They can model and simulate the distribution of market returns, which can help in risk management and trading/investment strategy development.
Radial Basis Function Networks (RBFNs)
RBFNs are used for interpolation in multidimensional space.
Applied in finance to predict asset prices by mapping nonlinear relationships between inputs and market prices.
Siamese Networks
Siamese Networks are unique for their ability to compare inputs.
In finance, they’re used for verifying the authenticity of transactions by comparing them against known fraud patterns.
Transformer Networks
Transformers process sequences of data, making them effective for high-frequency trading by analyzing sequences of market data to predict short-term market movements.
Capsule Networks
Capsule Networks are designed to recognize patterns in data hierarchically.
In finance, they could be used to identify market conditions that lead to specific outcomes, such as sudden stock price movements.
Reinforcement Learning Networks
Not a neural network itself but often combined with neural networks, reinforcement learning is used in algorithmic trading to develop strategies that improve over time based on rewards from successful trades.
But like most things in finance, sources of alpha will eventually erode as more people enter a trade.
Self-Organizing Maps (SOMs)
SOMs are used for clustering and visualizing high-dimensional data.
In finance, they help in market segmentation and portfolio analysis by organizing stocks into clusters based on similar performance patterns.
Let’s look at a few of these types of neural networks commonly used in finance in more detail (along with their example applications):
Feedforward Neural Networks (FNNs)
Comprises input, hidden, and output layers in a forward-only architecture.
Data moves from the input to the output layer, possibly through multiple hidden layers.
Applications in Finance
- Credit Scoring – Assessing the creditworthiness of borrowers by analyzing their financial history, demographic information, and other relevant data.
- Fraud Detection – Identifying potentially fraudulent transactions by recognizing patterns indicative of fraud.
Convolutional Neural Networks (CNNs)
Specialized for processing data with a known grid-like topology, such as time series or spatial data.
Uses convolutional layers to automatically detect and learn spatial hierarchies in data.
Applications in Finance
- Market Sentiment Analysis – Analyzing financial news or social media content to gauge “sentiment.”
- Anomaly Detection in Time Series Data – Identifying unusual patterns in financial time series, which could indicate market manipulation or errors in data recording.
- Image Processing – CNNs are most commonly used for image data.
Recurrent Neural Networks (RNNs) and Long Short-Term Memory Networks (LSTMs)
Designed to process sequences of data by having loops within them, which allows information to persist.
LSTMs are a special kind of RNN capable of learning long-term dependencies.
Applications in Finance
- Stock Price Forecasting – Predicting future stock prices based on historical price data and other related economic indicators.
- Algorithmic Trading – Developing trading strategies that adapt to new information and predict market movements.
- Time Series – Anything related to time series data.
Autoencoders
Neural networks designed for unsupervised learning of efficient codings by learning to compress the input data into a lower-dimensional code and then reconstructing the output from this code.
Applications in Finance
- Feature Extraction and Reduction – Reducing the dimensionality of financial data to identify the most significant features for further analysis or modeling.
- Portfolio Optimization – Analyzing correlations and dependencies among various assets to optimize portfolio allocations.
Generative Adversarial Networks (GANs)
Consists of two networks, a generator and a discriminator, that are trained simultaneously.
The generator tries to produce data that is indistinguishable from real data, while the discriminator tries to distinguish between real and generated data.
Applications in Finance
- Synthetic Financial Data Generation – Generating synthetic data for stress testing and scenario analysis purposes without exposing sensitive information.
- Market Simulation – Simulating market dynamics to test trading strategies or understand the potential impact of large trades.
Reinforcement Learning (RL) Models
Not a neural network per se but often combined with neural networks (deep reinforcement learning) to make decisions by learning policies that maximize some notion of cumulative reward.
For example, in a multi-strategy algorithmic trading system, an RL model might up-weight the strategy/strategies that are performing well and down-weight the ones that are performing less well.
Applications in Finance
- Portfolio Management – Dynamically adjusting portfolio allocations to maximize returns or minimize risk.
- Order Execution – Learning optimal trading execution strategies to minimize market impact and trading costs.
Probabilistic Neural Networks (PNNs)
A type of feedforward neural network that is based on the concept of statistical probability.
PNNs use a supervised learning algorithm and are particularly designed for classification problems.
They operate by estimating the probability density function of each class based on training data and then classifying new data based on which class’s probability density function is highest for that new data point.
Applications in Finance
- Credit Approval – Determining the likelihood that a loan applicant falls into different credit risk categories based on their financial indicators. PNNs can help in classifying loan applications swiftly with a probabilistic understanding of risk.
- Market Segmentation – Classifying customers or market segments based on purchasing behavior, risk profile, or other financial characteristics. This classification can help in tailored marketing, personalized product offerings, or risk assessment.
- Bankruptcy Prediction – Identifying companies at risk of bankruptcy by analyzing financial ratios and other indicators. PNNs can provide a probabilistic assessment of bankruptcy risk. Helps in investment decisions or credit extension.
Key Characteristics of PNNs in Finance
- Rapid Training – PNNs can be trained quickly. This makes them suitable for applications where the model needs to be updated frequently with new data.
- High Accuracy for Classification – Given sufficient training data, PNNs can achieve high accuracy in classification tasks. Useful for various financial services applications where decision speed and accuracy are important.
- Handling of Uncertainty – The probabilistic nature of PNNs allows them to handle unknowns in financial data effectively. Provides not just classifications but also confidence levels associated with those classifications.
Challenges & Considerations of Neural Networks
Overfitting
A primary concern with ANNs is overfitting, where a model performs well on historical data but fails to generalize to unseen data.
Regularization techniques and proper validation are important to handle this risk.
Data Quality and Availability
The performance of ANNs heavily relies on the quality and quantity of input data.
Inaccurate or incomplete data can lead to misleading predictions.
Interpretability
ANNs are often considered “black boxes” because understanding the internal workings and reasoning behind their predictions is challenging.
Efforts are being made to improve the interpretability of these models.
Market Dynamics are “Open Systems”
Financial markets are influenced by various factors, and are “open systems” in that the future won’t be like the past.
ANNs must be used cautiously, as one of many analytical tools.
They can’t capture the full spectrum of market-influencing factors.
A machine learning approach to stock trading | Richard Craib and Lex Fridman
FAQs – Neural Network
What’s the architecture and functioning of a neural network?
ANNs are structured in layers: input, hidden, and output.
The input layer receives raw data, which is then processed in hidden layers through weighted connections.
The output layer produces the final decision or prediction.
Training involves adjusting these weights based on the error of predictions, using algorithms like backpropagation.
What are some applications of neural networks in trading?
Some applications of neural networks in trading:
Pattern Recognition
ANNs excel in identifying patterns in market data.
They can discern trends and anomalies that might be imperceptible to human analysts, which can provide a significant advantage in predicting market movements.
Price Forecasting
ANNs are widely used for predicting asset prices and market indices.
By analyzing past price data and other relevant variables, they can help make better forecasts of future prices.
Algorithmic Trading
ANNs form the backbone of many algorithmic trading systems.
They can process vast amounts of market data in real-time, make predictions, and execute trades at speeds unattainable by human traders.
Risk Management
ANNs assist in evaluating and managing risk by predicting the volatility of assets and identifying potential risk factors in investment portfolios, which can help in the construction of risk-optimized portfolios.
Can you use deep learning for trading?
Yes, deep learning can be used for trading.
It is a type of machine learning that uses neural networks with multiple layers to extract features from data and make predictions.
In trading, deep learning models can be used to analyze and make predictions about financial market data, such as stock prices, to inform trading decisions.
However, it’s important to note that the stock market is highly complex and dynamic, and there is no guarantee that a deep learning model will be successful in predicting stock prices or generating profits.
How can a conditional neural network be used for the stock market?
A conditional neural network can be used to predict stock market trends by analyzing historical data and using it to inform predictions about future market behavior.
The network can be trained on a dataset of past stock prices, along with other relevant information such as news articles and leading economic indicators, and can use this information to make predictions about future stock prices.
The “conditional” aspect of the network refers to the fact that it can make these predictions based on specific input conditions, such as a particular stock or market sector.
This can be useful for making more accurate predictions and identifying potential trading opportunities.
Should I learn Python for trading?
Python is a popular programming language that is widely used in many industries, including finance and trading.
It has a large number of libraries and frameworks that can be used for data analysis, visualization, and machine learning, making it a useful tool for traders.
Python has several libraries like pandas, numpy, matplotlib, scikit-learn, etc., that are commonly used in trading, such as for data analysis and visualization, and for implementing machine learning models.
Additionally, many popular trading platforms, such as Interactive Brokers and Alpaca, have Python libraries that allow traders to connect to their platforms via an API and execute trades programmatically.
However, it’s worth noting that learning Python is not the only way to get into trading.
There are other programming languages such as R, Java, and C++ that can also be used, depending on the specific needs and requirements of the trader.
It’s important to evaluate the different options and select the one that best suits your needs.
How good are the predictive capacities of a conditional neural network?
The predictive capacities of a conditional neural network can vary depending on the specific task and dataset it is applied to.
However, in general, neural networks have been shown to have strong predictive abilities in a variety of fields, including finance.
In the context of the stock market, neural networks have been used to predict stock prices and returns with some success. Like with everything, it depends on the application.
However, it’s worth noting that predicting stock prices is a challenging task, and no model can predict with perfect accuracy.
Additionally, the stock market is complex and influenced by a wide range of factors, making it difficult to develop a model that consistently outperforms the market.
It’s also important to be aware of potential overfitting and bias issues when using neural networks for financial market predictions.
It’s essential to use proper techniques such as cross-validation and regularization to ensure the robustness and generalization of the model.
Conclusion – Neural Network
Neural networks are a type of machine learning algorithm that are modeled after the structure and function of the human brain.
They are able to learn from data and make predictions or decisions without being explicitly programmed to do so.
In the field of trading, neural networks can be used to analyze market data and make predictions about future price movements.
They can also be used to identify patterns and relationships in the data that humans may not be able to discern (or do so slowly and inefficiently).
This can be used for trading strategies such as predicting stock prices, foreign exchange rates, and commodity prices.
They can also be used in the execution of trades, such as identifying the optimal time to buy or sell a security.
However, it is worth noting that the application of neural networks in trading is a complex task and it’s not always guaranteed to work properly.
It’s important to have a good understanding of the data and the market, as well as to evaluate and test the performance of the neural network thoroughly before using it in a live trading environment.