Modern Trading & Portfolio Trends
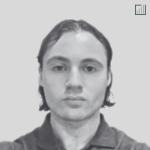
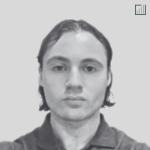
Modern finance and trading is seeing many ongoing shifts.
For example, we increasingly see a shift away from pure equity and credit strategies toward more balanced strategies – i.e., balancing risk across asset classes to enhance portfolio stability.
These methods address the limitations of traditional approaches that often concentrate risk in equities.
We’ll explore this and other key trends in trading and portfolio management, including algorithmic trading, AI and machine learning, ESG, and the influence of macroeconomic variables on trading decisions.
Key Takeaways – Modern Trading & Portfolio Trends
- Balanced Allocation Strategies
- There’s an increasingly greater focus on maximizing return for each unit of risk through diversification to enhance portfolio stability and resilience against market shocks.
- Leveraging Algorithmic Trading
- At one point, trading/investing was purely discretionary.
- As time has gone one, technology is doing more of the decision-making.
- Example: Machine learning algorithms for real-time data analysis and high-frequency trading to optimize trade execution and strategies.
- Other Factors
- We’ll also look at such things as the role of behavioral finance, macro variables, ESG, advances in derivatives and structured products, and more.
Risk Parity and Balanced Strategies
Risk parity is a portfolio construction strategy that challenges traditional thinking.
It shifts the focus from maximizing returns from concentration to balancing risk across different asset classes.
In a nutshell, it tries to make sure that each asset class, sub-portfolio, or source of return contributes equally to the overall risk of the portfolio.
Reason Behind the Popularity
Why is this important?
Traditional approaches often concentrate risk in one or a few asset classes, like equities.
Traders and portfolio managers often specialized in equities or credit, but now it’s going increasingly toward more of a “mash-up” approach where many different things are combined into optimally structured portfolios.
Concentration in one type of asset class leaves the portfolio vulnerable to market shocks.
Concepts like risk parity address this by diversifying risk across a broader range of assets, including bonds, commodities, and different returns streams (that might be individually engineered).
The goal is to create a more balanced and resilient portfolio that can weather different market environments.
For example, during periods of high inflation, commodities might outperform, while government bonds may offer stability during deflationary economic downturns.
By allocating risk across these assets, the portfolio is less likely to experience extreme swings in value.
Implementing risk parity requires a more quantitative approach, often involving leverage or leverage-like techniques so that equal risk contributions from each component.
It’s not a purely set-it-and-forget-it strategy, as regular rebalancing is necessary to maintain the desired risk allocation.
Old-school Soros-like approaches of concentrated betting are less and less common in favor of more diversified, well-structured portfolios.
For Both Trading and Investing Approaches
Risk parity and balanced allocation approaches benefit both trading and investing approaches such that risk is better distributed across returns streams and diverse asset classes, which enhances overall portfolio stability.
Even traders can benefit from these strategies as they provide a structured framework that they can deviate from for tactical trading decisions.
Example
This isn’t just theoretical.
If you take a very basic “balanced” portfolio:
- 35% Stocks
- 45% Bonds
- 20% Gold and Commodities
You can see how the drawdowns are less steep over time due to the different environmental biases and how this can help offset losses in the other:
Limitations
While risk parity has gained popularity, it’s important to understand its limitations.
It’s not a guaranteed path to higher returns, but rather a way to manage risk more effectively and getting more return relative to your risk over the long run.
Algorithmic Trading and High-Frequency Trading
Most of the market is run off algorithms and this is increasingly the case.
We’ve moved from simple rule-based systems to increasingly sophisticated machine learning algorithms that analyze massive datasets in real-time.
High-frequency trading (HFT), a subset of algorithmic trading, has taken this to the extreme, executing trades in microseconds.
Quantitative Investing and Factor Models
Quantitative investing has evolved from academic theories to real-world strategies driving significant portions of the market.
Early strategies focused on simple statistical arbitrage, but we’ve seen a shift toward more sophisticated models incorporating machine learning and alternative datasets.
Key factors like momentum, value, size, quality, and low volatility have been extensively studied and shown to deliver excess returns over the long term.
These factors capture different dimensions of risk and reward, allowing traders to systematically tilt their portfolios toward specific characteristics.
Integrating quantitative strategies with traditional portfolio management is a growing trend.
Quantitative models can help identify undervalued assets, optimize portfolio construction, and manage risk more effectively.
For example, a traditional value investor might use a quantitative model to screen for stocks with attractive valuations, while incorporating qualitative assessments of management quality or competitive positioning.
ESG and Sustainable Finance
ESG has moved from a niche concept to a mainstream force.
It’s not just about “doing good.”
Traders are recognizing that environmental, social, and governance factors can genuinely impact a company’s long-term performance and risk profile.
We see increasing demand for sustainable investment products, tighter regulations on corporate disclosures, and a growing awareness among traders/investors about the importance of sustainability.
But What About Portfolio Performance?
Contrary to some misconceptions, ESG doesn’t necessarily mean sacrificing returns or throwing out good returns streams because they don’t meet environmental criteria (or accepting bad returns streams in a portfolio because they’re ESG-friendly).
Companies with strong ESG practices can outperform their peers in the long run.
They tend to be better managed, more resilient to shocks, and aligned with evolving consumer preferences.
Integrating ESG factors into trading decisions is complex.
It requires analyzing a wide range of data, from carbon emissions to labor practices to governance practices.
Some investors use ESG ratings to screen out companies with poor practices, while others engage with companies to improve their ESG performance.
In essence, ESG is another dimension of risk and opportunity that can be considered alongside traditional financial metrics.
Behavioral Finance and Market Psychology
Behavioral finance challenges the traditional notion of perfectly rational investors and reveals how our emotions and cognitive biases can significantly impact trading decisions.
Consider loss aversion, for example.
Most traders feel the pain of a loss more acutely than the pleasure of an equal gain.
This often leads to holding onto losing positions for too long, hoping they’ll bounce back, or selling winners too early to lock in profits.
Another common bias is overconfidence.
We tend to overestimate our abilities and the accuracy of our predictions. This can lead to excessive trading, chasing hot stocks, and underestimating risks.
Confirmation bias is another culprit. We tend to seek out information that confirms our existing beliefs and ignore evidence that contradicts them.
This can reinforce our biases and lead to poor trading decisions.
So, how can we get rid of these biases?
Awareness is the first step.
By recognizing our own tendencies, we can take steps to counteract them.
This is also where diversification comes in. What we know is small in relation to what we don’t know – especially in relation to what’s discounted into markets.
Developing a disciplined trading plan, sticking to a pre-determined strategy (assuming it’s been adequately stress-tested and shown to work over time), and seeking out diverse viewpoints can help us make more rational decisions.
It’s also helpful to keep a trading journal to track our successes and failures, analyze our decision-making process, and identify any patterns of biased behavior.
Machine Learning and Artificial Intelligence in Finance
Artificial intelligence and machine learning are no longer futuristic concepts in finance. They’re actively reshaping how we trade and manage portfolios.
We’re seeing AI algorithms execute trades, optimize portfolios, assess risk, and even generate trading ideas.
Predictive Analytics
One of the most exciting applications lies in predictive analytics.
ML models can sift through mountains of data, uncovering patterns and relationships that humans might miss.
The human brain might be more creative in a lot of ways, but it can’t compare with the computer’s memory or raw calculation power.
This can lead to more accurate forecasts of market trends, asset prices, and risk exposures.
Big Data
Big data is more important.
With the explosion of information from social media, news feeds, satellite imagery, and more, AI algorithms can extract insights that inform trading decisions.
For example, sentiment analysis of social media posts can gauge market sentiment, while satellite images of retail parking lots can help predict customer volume and hence company earnings.
Challenges
Challenges remain.
AI models can be complex and difficult to interpret, raising concerns about transparency and accountability.
There’s also the risk of overfitting, where models become too specialized to past data and fail to generalize to new situations.
Looking Ahead
We can expect even more sophisticated algorithms, greater use of alternative data sources, and increased automation across the trading/investment process.
This could lead to more efficient markets, better risk management, and ultimately, improved outcomes.
But AI still remains a tool to augment human expertise, not replace it.
It’s likely that humans will increasingly take on more creative and strategic roles as machines perform better at more and more tasks.
Example
If one were to use LLM technologies in a portfolio, you wouldn’t blindly use it to pick stocks because that’s hopeless.
However, you could feed it information about current market conditions across various criteria, and ask it where it’s seen these circumstances before.
Then you can go and look at the market conditions during those times and in those societies and see how things played out going forward – e.g., policymaker decisions, economic and market outcomes, and so on.
Related: Day Trading with AI
Impact of Macroeconomic Variables on Portfolio Management
Interest rates, inflation, and economic cycles are key players that dictate economies and markets at a high level, influencing asset valuations and creating opportunities or threats.
Interest Rates
Take interest rates, for example.
When they rise, bond prices typically fall, and growth stocks might take a hit as their future earnings are discounted at a higher rate.
Conversely, lower interest rates can boost bond prices and make equities more attractive.
Inflation
Inflation, that silent thief of purchasing power, erodes the real value of investments and your portfolio.
It can favor certain things over time (e.g., diversified commodities), as their prices tend to rise with inflation, while nominal fixed-income assets may suffer.
Economic Cycles
Economic cycles, with their expansions and contractions, also leave their mark on portfolios.
During boom times, cyclical stocks and riskier assets often thrive, while defensive sectors like consumer staples might outperform during downturns.
Taking Them Into Account
Sophisticated traders use hedging strategies to reduce these macroeconomic risks.
This might involve using derivatives like interest rate swaps to offset interest rate risk, or buying inflation-protected securities to better hedge against inflation.
Case Study
The 2008 financial crisis, triggered by a housing bubble and credit crunch, led to a massive flight to safety, with traders flocking to government bonds and gold.
Throughout this traders have learned and gotten new data for backtesting purposes.
Innovations in Derivatives and Structured Products
Derivatives and structured products are constantly evolving, driven by demand for sophisticated risk management and return enhancement methods.
One trend you see is the rise of increasingly exotic options with complex payoffs linked to multiple underlying assets or specific market conditions.
These instruments cater to specific risk profiles and objectives, but require careful understanding due to their inherent complexities.
Structured Products
Structured products, which combine derivatives with traditional securities like bonds, are also gaining traction.
They offer tailored risk-return profiles, allowing traders/investors to express specific market views or participate in particular asset classes while reducing downside risks.
Autocallable notes, for instance, provide potential upside linked to equity markets with a built-in protection mechanism if markets decline.
Regulatory Implications
As derivatives and structured products become more complex, regulators grapple with transparency concerns, managing systemic risks, and protecting traders/investors from mis-selling.
Recent regulatory reforms like the Dodd-Frank Act in the US have introduced stricter capital requirements and reporting obligations for dealers and users of derivatives.
For portfolio managers and traders, understanding these developments is important.
Derivatives and structured products can be used for hedging, income generation, or expressing standard strategic or tactical views.
But they require expertise due to the complexities and risks involved.
They require thorough due diligence, an assessment of the suitability of these products for specific portfolios, and stay abreast of new developments.