Types of Heavy-Tailed Distributions for Financial Data
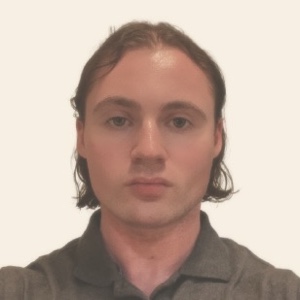
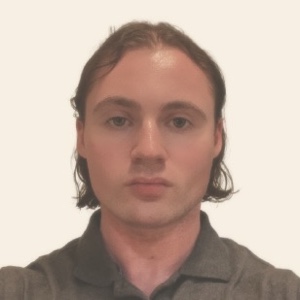
Heavy-tailed distributions (or fat-tailed distributions) are used in financial modeling as these probability distributions better capture the behavior of financial data.
The occurrence of extreme events such as market drops or booms are more common than would be predicted by normal (Gaussian) distributions.
These distributions have “heavy tails” – i.e., a higher likelihood of extreme values – which can impact trading strategies and risk management.
Understanding these distributions allows traders to better model risk and return.
Key Takeaways – Types of Heavy-Tailed Distributions in Finance
- Expect the unexpected
- Markets go beyond the bell curve and have “fat tails” – rare events are more likely than normal models predict.
- It’s important to prepare for price falls and rises beyond “normal” risk measures.
- Don’t underestimate risk
- The usual tools might underestimate risk of big losses. Use stress tests and heavy-tailed models to understand potential dangers.
- Hedge
- Diversify to thin out your tails, use options, and set realistic stop-loss orders. Surviving the storm matters more than maximizing every sunny day.
Types of Heavy-Tailed Distributions for Financial Data
Pareto Distribution
This distribution is used to model the distribution of wealth, returns, or other financial variables that exhibit a “rich-get-richer” phenomenon.
It has a heavy tail on the right side, indicating a small probability of very large values.
Cauchy Distribution
Unlike the normal distribution, the Cauchy distribution has fatter tails.
This makes it suitable for modeling financial instruments that can have sudden large deviations.
Its mean and variance are undefined, which reflects markets better.
Levy Distribution
The Levy distribution is another heavy-tailed distribution used in financial modeling, most commonly used for describing the distribution of stock price movements (because of their volatility).
It captures the asymmetry and leptokurtosis (excess kurtosis) often observed in financial returns.
Stable Distributions (including Alpha-Stable)
Stable distributions encompass a range of distributions that are capable of modeling heavy tails and skewness.
The alpha-stable distribution, in particular, is versatile for modeling financial data due to its ability to adjust for varying degrees of tail heaviness and asymmetry.
Student’s t-Distribution
With heavier tails than the normal distribution, the Student’s t-distribution can better model financial returns.
It’s useful for assets that may experience large jumps or drops.
It is parameterized by degrees of freedom, with lower values indicating heavier tails.
Generalized Hyperbolic Distribution
This distribution is flexible, and capable of modeling asymmetric heavy tails and skewness in financial return distributions.
It’s useful for assets that exhibit volatility clustering, which is a common feature in financial markets.
Weibull Distribution
While often associated with life data analysis, the Weibull distribution can model the tail behavior of financial returns – especially when considering the time until the first occurrence of a significant market movement.
Lognormal Distribution
The lognormal distribution is used to model stock prices and other financial variables that can’t assume negative values.
It has a heavy right tail.
Generalized Extreme Value (GEV) Distribution
GEV distribution is used for modeling the maxima or minima of long sequences of transactions or financial returns.
It’s useful in risk management for assessing the probability of extreme tail events.
Generalized Pareto Distribution (GPD)
The GPD is used in extreme value theory to model tails of distributions and is useful for modeling tail risks and the likelihood of extreme losses.
Non-Parametric Distributions
Non-parametric distributions adapt to data and don’t assume a specific functional form.
With more of quantitative finance going toward machine learning approaches, non-parametric approaches are becoming more common in representing financial, economic, and market data.
Applications of Heavy-Tailed Distributions in Trading
Risk Management
Heavy-tailed distributions are instrumental in calculating Value at Risk (VaR) and Expected Shortfall (ES) more accurately.
These metrics are important for traders to assess the risk of their portfolios and to set aside capital for potential losses.
Portfolio Optimization
Understanding the tail behavior of asset returns allows traders to construct portfolios that are more resilient to extreme market movements.
This involves diversifying not just across assets but also considering the tail dependencies among them.
Option Pricing
Traditional option pricing models, like the Black-Scholes model, assume normality in the underlying asset returns.
However, models that incorporate heavy-tailed distributions can provide more accurate pricing for options, especially those with long maturity or out-of-the-money options, where the impact of extreme movements is more pronounced.
Market Stress Testing
Traders use heavy-tailed distributions to simulate extreme market conditions and test how their portfolios would perform.
This helps in developing strategies that can withstand market shocks.
Algorithmic Trading
For traders using quantitative models, incorporating heavy-tailed distributions can improve the prediction of extreme price movements, which can lead to strategies that can capitalize on high volatility periods.
Machine Learning Techniques for Estimating Distributions in Financial Data
Flexible machine learning models can capture complex empirical finance and risk distributions without oversimplifying assumptions about the stochastic dynamics.
Here are some common machine learning techniques for estimating distributions in financial data and their high-level descriptions:
Kernel Density Estimation (KDE)
Estimates probability density function directly from the data using kernel functions centered on each observation.
It essentially smoothes out histograms to reveal the underlying, continuous probability distribution of the data.
Mixture Density Networks
Neural networks that model overall density as a mixture of simpler parametric distributions.
Normalizing Flows
Invertible neural networks that transform a simple base distribution into a complex one matching the data.
Gaussian Processes
Nonparametric Bayesian approach that models a distribution over functions and performs probabilistic regression.
Hidden Markov Models
Model financial time series as a Markov process between hidden states with emissions probabilities.
Score Matching
Parameter estimation by minimizing discrepancies between empirical and model score functions derived from densities.
Quantile Regression
Instead of modeling the full distribution, quantile regression estimates conditional quantiles to characterize broader behaviors.
Conclusion
For traders, the acknowledgment and application of heavy-tailed distributions are essential for accurately modeling financial data and managing risks.
These distributions provide a more realistic framework for understanding their data – especially the likelihood of extreme events.
By leveraging these distributions, traders can enhance their strategies for risk management, option pricing, and portfolio optimization, and help with better decision-making.