Volatility Clustering
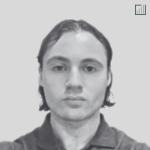
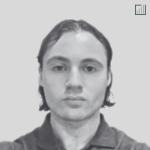
In finance, volatility clustering refers to the observation that large price changes in financial markets are often followed by more large changes, and similarly, small changes are typically succeeded by more small changes.
This observation was first noted by Mandelbrot in 1963, who stated that “large changes tend to be followed by large changes, of either sign, and small changes tend to be followed by small changes.”
Key Takeaways – Volatility Clustering
- Persistence of Volatility:
- Volatility clustering refers to the observation that high volatility days in financial markets tend to be followed by high volatility days, and low volatility days tend to be followed by low volatility days.
- Risk Management Implication:
- For traders, this means that after a period of significant price swings, they should be prepared for continued volatility.
- They can adjust their risk management strategies or portfolio construction strategies accordingly.
- Predictive Power:
- Recognizing volatility clustering can offer traders an edge.
- It suggests that volatility is not random but has a degree of predictability, aiding in more informed trading decisions.
Quantitative Roots of Volatility Clustering
A more quantitative understanding of this observation reveals that while returns in financial markets might appear uncorrelated, their absolute returns or their squares exhibit a different behavior.
Specifically, the autocorrelation function of these absolute returns displays a positive, significant, and slowly decaying nature.
This means that the correlation between the absolute returns at time “t” and those at a later time “t+τ” remains positive for durations ranging from mere minutes to several weeks.
This empirical property was extensively documented in the 1990s.
Notable contributions in this area were made by Granger and Ding in 1993 and Ding and Granger in 1996.
Some studies, such as those by Ding, Granger, and Engle in 1993 and Barndorff-Nielsen and Shephard, have pointed to the existence of long-range dependence in volatility time series.
Implications for Financial Models
The observations of volatility clustering challenge the foundational assumptions of simple random walk models in finance.
These observations have been instrumental in steering the financial community towards more sophisticated models that can better capture the nuances of market volatility.
One of the primary responses to this challenge has been the development and adoption of GARCH models and mean-reverting stochastic volatility models.
These models have become cornerstones in financial forecasting and the pricing of options and derivatives.
The ARCH and GARCH Models
The ARCH (Autoregressive Conditional Heteroskedasticity) model, introduced by Engle in 1982, and its generalized version, the GARCH (Generalized Autoregressive Conditional Heteroskedasticity) model, proposed by Bollerslev in 1986, were developed to provide a more accurate description of volatility clustering and related phenomena, such as kurtosis.
The central premise behind these models is the idea that volatility is not an independent or random factor.
Instead, it is influenced by past realizations of both the asset process and the associated volatility process.
This concept encapsulates the intuition that asset volatility tends to revert to a mean value over time, rather than remaining constant or moving in a singular direction.
ARCH vs. GARCH
ARCH models volatility as a function of past error terms, capturing the idea that large price changes tend to be followed by more large changes.
GARCH extends ARCH by including lagged values of the forecast variance itself, allowing for a more flexible modeling of the volatility process.
Both models are essential for understanding and forecasting the volatility of financial returns, with GARCH being more widely used due to its enhanced adaptability.
Volatility Clustering & Options Prices
Volatility clustering impacts options pricing.
When markets exhibit volatility clustering, options prices tend to rise due to the increased perceived risk.
Traders anticipate more volatile periods following volatile days, leading to higher premiums for options.
This can open up new trading opportunities for those looking to, e.g., harvest the volatility risk premium.
FAQs – Volatility Clustering
What is volatility clustering?
Volatility clustering in finance refers to the phenomenon where periods of high volatility in asset prices are often followed by similar periods of high volatility, and periods of low volatility are followed by similar low volatility periods.
How is volatility clustering quantified?
A quantitative representation of volatility clustering is that while returns themselves might not be correlated, their absolute returns or their squares display a significant and positive autocorrelation function.
This means that the correlation between absolute returns at different time intervals, represented as corr(|rt|, |rt+τ |), remains positive for time intervals ranging from a few minutes to several weeks.
What causes volatility clustering in financial markets?
Volatility clustering in financial markets arises when large price changes are followed by large price changes, and small price changes are followed by small price changes, regardless of the direction.
This phenomenon is often attributed to the flow of information in the market, where news or events can lead to periods of high or low volatility.
Generally when “there’s a lot going on” it doesn’t resolve in one or a few days, leading to higher volatility over a period of time.
Likewise, when there’s “not much going on” then that tends to persist as well, causing lower volatility.
How does volatility clustering affect investment and trading strategies?
Volatility clustering can influence trading and investment strategies by prompting traders/investors to adjust their portfolios in response to perceived risks.
For instance, during periods of high volatility, risk-averse investors might reduce their exposure to more volatile assets, while risk-seeking investors might see it as an opportunity to capitalize on price swings.
This activity can cause new activity, which causes new activity, and so on, leading to more volatility.
Likewise, when there’s low activity, this can also lead to less activity from others, and so on.
Are there any models or theories that explain volatility clustering?
Yes, the most prominent model that explains volatility clustering is the Autoregressive Conditional Heteroskedasticity (ARCH) model and its generalized version, the GARCH model.
These models capture the changing variance in financial time series data and are widely used in financial econometrics.
How do GARCH and ARCH models interpret volatility?
Both the GARCH and ARCH models operate on the principle that volatility is not constant or monotonically changing over time.
Instead, they suggest that asset volatility tends to revert to a mean value.
For example, it’s commonly noted that the S&P 500 tends to have an annualized volatility over 15% on average (when represented as a standard deviation).
This reversion is based on the past behaviors of the asset and its volatility, capturing the essence of volatility clustering in financial time series.
How does volatility clustering relate to financial crises?
Volatility clustering often becomes more pronounced during financial crises and downturns.
As uncertainty and panic increase in the market, large price swings become more common, leading to periods of heightened volatility.
This clustering can exacerbate market downturns as traders react to the increased risk.
What are the implications of volatility clustering for risk management?
For risk management, volatility clustering implies that risk is not constant over time.
Risk managers need to account for changing volatility levels when assessing portfolio risk and determining capital reserves.
Ignoring volatility clustering can lead to underestimation of potential losses.
Can volatility clustering be predicted?
While individual price movements are challenging to predict, the persistence of volatility can be forecasted to some extent using models like GARCH.
These models can provide insights into future volatility based on past data, but they are not always perfect in their predictions.
How do different asset classes exhibit volatility clustering?
Different asset classes, such as stocks, bonds, commodities, and currencies, can all exhibit volatility clustering, but the intensity and duration might vary.
For instance, commodities might experience sharp volatility due to supply-demand shocks, while stocks might react strongly to corporate news or macroeconomic events.
What is the difference between volatility clustering and autocorrelation in returns?
Volatility clustering refers to the persistence of volatility over time, while autocorrelation in returns refers to the correlation of a time series with its lagged values.
In simpler terms, volatility clustering focuses on the magnitude of price changes, while autocorrelation focuses on the direction and pattern of returns.
How does volatility clustering impact portfolio diversification?
Volatility clustering can challenge the benefits of portfolio diversification.
During periods of high volatility, correlations between asset classes might increase, reducing the diversification benefits.
Traders need to be aware of this and might need to consider this in their portfolio construction process.
Are there any tools or software specifically designed to analyze volatility clustering?
Yes, various financial software and econometric packages, such as R, Python’s statsmodels, and MATLAB, offer tools to analyze volatility clustering.
These tools often include implementations of the ARCH and GARCH models, among others.
How do central banks and policymakers respond to volatility clustering?
Central banks and policymakers monitor volatility clustering closely as it can signal financial instability.
In response, they might adjust monetary policy, implement macroprudential measures, or intervene in markets to stabilize them.
What role do high-frequency trading and algorithmic trading play in volatility clustering?
High-frequency trading (HFT) and algorithmic trading can amplify volatility clustering.
As these strategies react quickly to market information, they can lead to rapid price changes, especially in illiquid markets or during times of stress.
Are there any notable historical events that serve as examples of volatility clustering?
The 2008 financial crisis is a notable example where volatility clustering was evident.
Following the collapse of Lehman Brothers, global financial markets experienced prolonged periods of high volatility.
Other examples include the dot-com bubble burst in 2000 and the Black Monday crash in 1987.
Why is volatility clustering significant in financial modeling?
Observations of volatility clustering in financial time series challenge the assumptions of simple random walk models.
Recognizing this phenomenon has led to the development and use of more sophisticated models like GARCH and mean-reverting stochastic volatility models.
These models are used in financial forecasting and derivatives pricing to account for the observed volatility patterns.
Conclusion
Volatility clustering is an important concept in finance, offering insights into the behavior of financial markets and challenging traditional models.
The development of models like ARCH and GARCH were developed to understand and forecast market volatility more accurately.