5+ Most Abstract Concepts in Finance, Trading & Investing
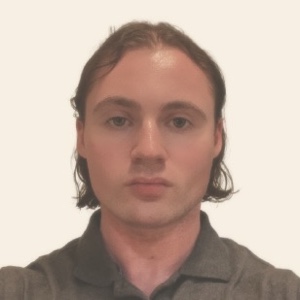
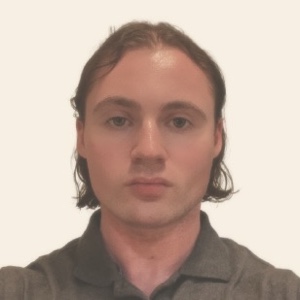
Let’s look at some of the most abstract concepts in finance, trading, and investing.
Key Takeaways – Most Abstract Concepts in Finance, Trading & Investing
- Strategic vs. Tactical Asset Allocation:
- Strategic asset allocation is a passively-held, well-diversified portfolio, while tactical asset allocation involves active tilts and trading.
- Tactical trading is hard and often leads to inefficient portfolio construction.
- It’s essential to maintain a sound portfolio structure and balance.
- Financial Economy vs. Real Economy:
- It’s important to understand the difference between the financial and real economy, and the forward-thinking nature of the financial economy.
- Bayesian Statistics in Finance:
- Bayesian statistics is a mathematical approach used in finance to update the probability of a hypothesis as more evidence or information becomes available.
- Understanding Correlations:
- Correlations analyze the relationships between different assets and asset classes, helping in diversifying portfolios and managing risk.
- Correlations can change over time and misinterpreting them can lead to flawed trading or investment strategies.
- It’s essential to understand the intrinsic drivers behind asset movements and not rely solely on historical data.
- Decision Making Over Time:
- In finance, decisions unfold over a temporal spectrum, similar to a game of poker.
- Future implications, potential for gains or losses over time, and prospective shifts in market dynamics are considered.
Strategic Asset Allocation vs. Tactical Asset Allocation
In professional investment management, security selection is generally not as important as it’s portrayed.
It has importance, but it’s secondary to the structure and overall construction of the portfolio.
You’re taking various assets and customized return streams and combining and amalgamating them within a certain structure to achieve whatever the particular risk parameters, return objectives, and other goals of the portfolio might be.
The covariances and correlations among assets/returns streams (based on what they’re intrinsically like) are important, so single assets and asset classes aren’t viewed in isolation.
And some things in a portfolio are not part of the core asset allocation but structured as overlays such that they don’t take resources away from the core assets of the portfolio. Common examples would be gold and currency overlays.
In terms of the difference between strategic asset allocation and tactical asset allocation…
Strategic Asset Allocation
- Strategic asset allocation refers to a type of passively-held base portfolio.
- It should be relatively well-diversified among assets, asset classes, countries, and currencies and creates balance between various outcomes.
- This means balancing out the assets to offset their intrinsic environmental biases, which all asset classes have (an environment where they do well and an environment where they do poorly).
For example:
- Stocks do well when growth is above expectation and inflation is steady or falling relative to expectations.
- Nominal-rate government bonds do well when growth and inflation are below expectation.
- Commodities do well when growth and inflation are above expectation.
- Cash does best when money and credit are tight. And so on.
Holding the various things in a balanced way (based on how money and credit flows are interconnected) helps to offset their individual biases and extract risk premiums from the market in an efficient way.
And comes with a host of other benefits – more return per unit of risk, lower volatility, lower left-tail risk, lower drawdowns, and so on.
Wealth circulates more than it’s destroyed.
Because an asset class goes down doesn’t necessarily mean money/credit disappeared; it usually means it flowed somewhere else (e.g., different asset, different asset class, different country, different currency, converted to cash to spend in the real economy).
A basic stock index might be 6% forward annual returns @ 15% volatility.
A balanced mix might be 5-6% forward annual returns @ 5-8% volatility, depending on how it’s constructed.
If someone wanted a balanced mix but needed more return, that’s a dial that can be turned to get them there. (You don’t have to accept anything a certain way just because it comes prepackaged that way.)
If they wanted even less risk, they could hold more cash against it.
For those really gunning for high average annual returns in public markets (20-30%+), that’s where holding options/derivatives to chop off the left-tail risk beyond a certain point becomes more important.
There are also capacity constraints beyond a certain level.
Tactical Asset Allocation
Then there’s the tactical asset allocation, which involves active tilts and trading (“this is good, this is bad”).
Tactical is a zero-sum game (negative-sum when factoring in transaction costs) and highly discouraged for individuals and most professionals who generally lack an informational, analytical, or technological edge to add value to that and would just end up losing relative to what a good strategic mix would offer.
Doing it can lead to inefficient portfolio construction (too much uncompensated risk).
And if they do want to do the tactical stuff, try to keep it within the context of a sound portfolio structure and balance.
Why Tactical Asset Allocation Is Hard
Tactical trading is hard because everything that’s known is already discounted into the price.
The bets are a lot more equal than most realize after adjusting for risk because of the human and technological resources that are dedicated to combing over everything (especially in public markets).
Betting on a good company isn’t necessarily better than betting on a weaker one.
It’s the same thing with betting at the race track as an example.
There are faster horses, there are slower horses.
But that information is already discounted based on the way their odds are handicapped.
So, betting on any horse is roughly equally good or bad from an expected value standpoint.
Financial Economy vs. Real Economy
The financial economy (supply and demand for money and credit) is also different than the real economy (supply and demand for goods and services), though most assume financial market outcomes should simply mirror real economy outcomes in a co-temporaneous way.
The financial economy (markets) leads the real economy.
Most think a big, booming economy should be the best environment for the stock market.
But the issue is that the financial economy is forward-thinking and relative to the discounted expectations that are already embedded in current market pricing.
When prevailing conditions become extrapolated forward in an inappropriate way (e.g., because what caused things to go up in price is not sustainable – such as debt-fueled booms), underperformance relative to what’s discounted becomes more likely than the opposite.
Just like a slow-moving economy is typically one of the best periods for stocks for the same reason.
So, following from the section above, when people are trading tactically based on their subjective instincts, that’s generally a recipe to performing poorly.
When untrained people give their reasoning (or have random opinions) why they think something should go a certain way, because they view the financial and real economy as the same and aren’t generally cognizant of how markets work based on discounted expectations, the opposite of their views is actually more likely to be correct.
Even when they think their logic is sound and obvious it’s probably wrong and not the best idea for most individuals to actively compete in a highly competitive game where a small percentage make a lot and most lose (relative to a benchmark).
This is borne out in the data looking at retail trading account returns vs. simply holding low-cost broad market indices.
And the risk premium element is another important dimension. There’s a lot to be said for going heavier on a fat return stream when the risk/reward is assessed correctly.
But a lot of these are usually smaller, lower-capacity opportunities that tend to be process-driven and tied to a particular skill set. It’s also much more relevant in private markets.
Probabilities
People generally struggle with probabilities because they want certainty.
However, trading is always a game of probabilities.
We’ll look at Bayesian statistics more closely.
Bayesian Statistics
Bayesian statistics is a mathematical approach to calculating probability where the calculation of the probability of an event occurring is influenced by prior knowledge of conditions that might be related to the event.
This is in contrast to frequentist statistics, where probabilities are determined solely based on the frequency of occurrences over a large number of trials.
Components
- Prior Probability (P(A)): This is the probability of an event A occurring, based on existing knowledge before considering new evidence.
- Likelihood (P(B|A)): This is the probability of observing the new evidence B given that event A is true.
- Marginal Likelihood (P(B)): This is the total probability of observing the evidence B, considering all possible values of A.
- Posterior Probability (P(A|B)): This is the updated probability of event A occurring given the new evidence B. It is calculated using Bayes’ theorem.
Application
In practice, Bayesian statistics is used in a wide range of fields including science, engineering, and economics to update the probability of a hypothesis as more evidence or information becomes available.
Example
Earnings reports are an example of Bayesian statistics.
They allow traders to update their beliefs about a company’s financial health.
Initially, investors have prior beliefs (or prior probabilities) about a company’s performance.
When a new earnings report is released, it provides new data.
Using Bayesian statistics, traders combine their prior beliefs with this new data to form updated beliefs (or posterior probabilities) about the company’s future prospects.
In essence, the new earnings information is used to update and refine old beliefs, embodying the Bayesian principle of integrating prior knowledge with new evidence.
Overall, Bayesian statistics offers a flexible approach to statistical analysis by allowing for the incorporation of prior knowledge and uncertainty in the analysis.
It’s a tool for making informed decisions based on incomplete or evolving data.
Correlations
Correlations help in diversifying portfolios, managing risk, and identifying investment opportunities by analyzing the relationships between different assets and asset classes.
Correlation refers to the statistical measure that expresses the extent to which two securities move in relation to each other.
Correlations are usually measured mathematically on a scale from -1 to 1.
A correlation of 1 indicates that two assets move perfectly in tandem, a correlation of -1 means they move in opposite directions, and a correlation of 0 indicates no relationship in their movements.
They’re sometimes shown as percentages as well.
However, what’s most important when it comes to correlation isn’t just “A and B are 60% correlated” but rather understanding the intrinsic drivers behind their movement.
Let’s look at some examples.
Copper-Stocks Correlation
Historical Context:
- Historically, copper prices and stock market indices have shown a positive correlation.
- Copper is often viewed as a barometer for the global economy because of its wide-ranging industrial applications.
- When the economy is good, demand for copper rises, pushing its price up.
- This uptrend is often mirrored in stock prices as corporate profits and economic activity increase.
However, copper and stocks each have their own unique supply and demand characteristics.
Traders who rely on the belief that copper and stocks are positively correlated may make suboptimal decisions if they’re relying on that holding.
Stocks-Bonds Correlation
Historical Context:
- Generally, stocks and bonds have been traditionally thought of as having an inverse correlation by the current generation of traders/investors because of their experiences. When stock prices rise, bond prices tend to fall, and vice versa.
- However, when inflation volatility is more of a factor than growth volatility, then this correlation can flip.
Overall, stocks and bonds and not inherently diversifying.
In a world where growth is the main driver of returns at the macro level, stocks and bonds generally represent offsetting flows in markets.
However, when inflation becomes the bigger driver to returns, stocks and bonds can have a positive correlation and dip together.
This is why the traditional 60/40 is considered flawed and why changing its composition to include more inflation-linked bonds and commodities is a good idea.
Challenges and Considerations to Correlations
Changing Correlations:
- Correlations are not static. They can change over time due to various economic, geopolitical, and market-specific factors.
- For instance, during times of economic distress and market liquidity issues, traditionally uncorrelated assets may move in sync.
Misinterpretation:
- Misinterpreting correlations can lead to flawed investment strategies. Just because two assets have moved together in one way or another in the past does not guarantee they’ll do so in the future.
- Correlation does not imply causation, and investors should conduct comprehensive analysis beyond just correlation metrics.
How Decisions Transpire Over Time
In finance/investing, decisions unfold over a temporal spectrum, much like in a game of poker.
The concept of how decisions transpire over time is a nuanced one, involving a forward-looking perspective, a consideration of potential outcomes, and an assessment of risks and rewards.
In poker, a player doesn’t just consider the immediate odds related to the pot (pot odds), which represent the current value of the pot versus the cost of a contemplated call.
They also think about implied odds and reverse implied odds, which take into account the expected future bets that they can win or lose.
Implied odds assess the likelihood of winning additional money if a drawing hand improves, while reverse implied odds estimate the losses if the hand does not improve or if an opponent’s hand gets better.
Poker Example
For example, a poker player might have two spades pre-flop and see two spades on the flop, giving them two more cards to make a flush.
Even though they might currently have nothing, they know they have close to a 50% chance of hitting their flush, which can allow to win a lot of chips on future streets.
In this case, they’ll generally choose to call bets that make sense. Even if they miss their flush, they could potentially turn the hand into a bluff, which is a necessary for optimal results in a mixed-strategy game.
Finance Example
This concept parallels the decision-making process in finance and investing.
Traders, investors, and financial professionals don’t just look at the current market conditions or the immediate potential return on an investment.
They consider the future implications of their investment decisions, the potential for gains or losses over time, and the prospective shifts in market dynamics.
For instance, an investor considering investing in a start-up doesn’t only assess the current valuation of the company and the potential return on investment.
They also contemplate the future growth trajectory of the company, the scalability of its business model, the competitive landscape, and potential exit strategies.
These future-oriented considerations significantly impact the investment decision, much like how implied and reverse implied odds influence a poker player’s decision to call or raise a bet.
Moreover, just as a poker player adjusts their strategy based on the playing styles and tendencies of their opponents, investors and traders adapt their investment decisions based on evolving market conditions, economic indicators and new data, and other events.
They continuously reassess their investment thesis and strategy as new information emerges, ensuring that their decisions are aligned with their financial goals and risk tolerance.
And what we think is possible is just a function of what we know right now. Virtually everything is possible, it’s the probabilities that matter.