Weather Derivatives Models
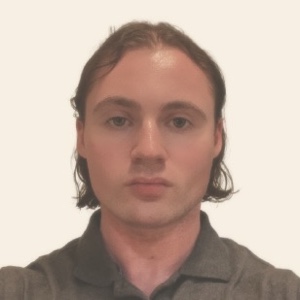
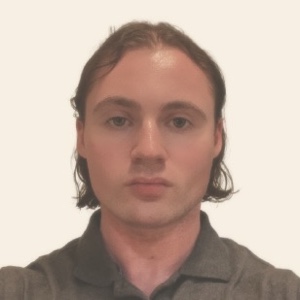
Weather derivatives are financial instruments that can be used to hedge or speculate on the impact of weather conditions, such as temperature, rainfall, or snowfall.
These instruments are used by businesses whose financial performance is significantly affected by weather, such as agriculture, energy, and tourism sectors.
There are various models used to price and manage weather derivatives, and their choice depends on the specific characteristics of the derivative contract, including the:
- underlying weather variable
- geographical location, and
- contract period
Key Takeaways – Weather Derivatives Models
- Hedging Against Weather Risks
- Weather derivatives allow businesses to manage financial risks associated with weather variability.
- Used in sectors like agriculture, energy, and insurance.
- Modeling Challenges
- Accurate pricing relies on complex models that predict weather patterns and their economic impacts.
- Requires advanced statistical and meteorological analysis.
- Market Adaptability
- These derivatives demonstrate financial innovation by converting weather uncertainty into tradable assets, and offers new hedging and investment opportunities.
Temperature-Based Models
These models are often used for temperature-related derivatives like heating degree days (HDD) and cooling degree days (CDD).
They may involve using historical temperature data to forecast future temperatures and calculate the expected payout.
Statistical methods like regression analysis, time series analysis, or even machine learning techniques can be applied.
Precipitation Models
For derivatives based on rainfall or snowfall, historical precipitation data is analyzed.
These models can be complex, as precipitation is more variable and less predictable than temperature.
They often involve stochastic processes or even hydrological models.
Simulation Models
These involve simulating weather patterns and outcomes using historical data.
Monte Carlo simulations are commonly used, which involve generating a large number of random but plausible weather scenarios to estimate the distribution of potential outcomes.
Index-based Models
These models are based on an index value derived from weather data, such as the amount of rain or the average temperature over a certain period.
The derivative pays out based on the deviation of the actual index value from a predetermined threshold.
Hybrid Models
In some cases, hybrid models combining elements of statistical and simulation approaches may be used.
These can be particularly useful for complex weather derivatives that involve multiple weather variables.
Catastrophic Models
For derivatives linked to catastrophic weather events like hurricanes or floods, models may incorporate climatological data, historical event frequencies, and physical models of weather phenomena.
Weather Derivatives Creation
Quants and financial engineers approach weather derivatives with the intent to design products to mitigate financial risks associated with adverse weather conditions.
Financial engineers create these instruments by first identifying the specific weather-related risk exposure of a business.
This might be temperature fluctuations for an energy company or rainfall levels for an agricultural firm.
The design process involves several steps:
Risk Assessment
Evaluate the financial impact of various weather conditions on the business to identify which weather variables (e.g., temperature, precipitation, wind speed) are most relevant.
Data Analysis
Collect and analyze historical weather data for the relevant variables and geographical location.
This analysis helps in understanding the variability and patterns in weather conditions.
Structuring the Derivative
Choose the appropriate structure for the derivative, such as options or swaps, based on the client’s risk management needs.
The payout of the derivative is typically linked to an index (e.g., HDD, CDD, rainfall index) that quantifies the specific weather condition.
Modeling and Pricing
Develop a mathematical model to price the derivative.
This involves using statistical and probabilistic methods to forecast future weather conditions and their potential impact on the index.
Monte Carlo simulations, time series analysis, and regression models are common techniques used for modeling and pricing weather derivatives.
Parameter Selection
Set the parameters of the derivative, including the strike value (the point at which payouts begin), the cap (maximum payout), and the contract period.
Backtesting and Calibration
Test the model against historical data to ensure its accuracy and adjust parameters as necessary.
Summary
Financial engineers leverage their expertise in mathematics, statistics, and financial theory, along with programming and/or modeling software, to design weather derivatives that provide tailored risk management solutions to businesses exposed to weather variability.
Conclusion
When structuring and pricing weather derivatives, it’s good to have a deep understanding of the underlying weather dynamics, access to quality weather data, and expertise in statistical and financial modeling.
Additionally, considerations around the liquidity of the instrument, counterparty risk, and regulatory environment are also important.
Related