Energy Derivative Models
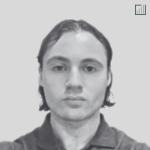
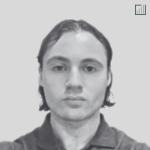
Energy derivatives are financial instruments whose value is derived from the underlying energy products like oil, natural gas, electricity, or renewables.
These derivatives are commonly used for hedging and speculative purposes.
This allows traders to manage or take on the risk associated with the price volatility of energy commodities.
Key Takeaways – Energy Derivative Models
- Market-Specific Factors
- Energy derivative models incorporate unique market drivers like weather conditions, regulatory changes, and supply-demand imbalances for accurate pricing and hedging.
- Volatility and Correlation
- They account for high volatility and the correlation between energy prices and other economic indicators to manage risks in energy portfolios.
- Complexity in Structuring
- Designing energy derivatives requires understanding complex physical markets and contract structures.
Let’s explore the typical models used for pricing and risk management of energy derivatives.
Black-Scholes Model
Originally developed for stock options, the Black-Scholes model is also applied to energy derivatives, especially those where the underlying asset can be approximated as following a geometric Brownian motion.
Nevertheless, the model’s assumptions, such as constant volatility, limit its accuracy for energy commodities which often exhibit mean-reversion and seasonal volatility.
Geometric Brownian Motion (GBM)
GBM is a stochastic process used to model the price paths of commodities, including energy products.
It incorporates a drift and volatility component to reflect the noisy/random nature of price movements.
It’s a fundamental model in quantitative finance and often used as a starting point for more complex models.
Mean-Reversion Models
Energy prices often exhibit mean-reverting behavior, where prices tend to move toward a long-term average over time (while still adjusting for inflation to reflect the appropriate nominal terms).
Models like the Ornstein-Uhlenbeck process or the more general mean-reverting jump diffusion model are used to capture this characteristic.
These models are more suitable for commodities like electricity, which show strong mean-reversion due to physical constraints and market conditions.
Multi-Factor Models
These models consider several factors affecting the price of energy derivatives, such as spot price, convenience yield, and interest rates.
Multi-factor models can provide a more comprehensive view of the dynamics in energy markets, and are popular for complex derivatives.
Monte Carlo Simulations
Due to the complexity and non-linearity in energy markets, Monte Carlo simulations are often used.
They involve simulating a large number of possible price paths for the underlying energy commodity and calculating the derivative’s value across these scenarios.
Weather Derivative Models
For energy derivatives linked to weather conditions, like temperature or rainfall, models that incorporate weather forecasts or historical weather patterns are used.
These derivatives are important for energy sectors heavily influenced by weather, such as natural gas and electricity.
Hybrid Models
Hybrid models combine elements from different models to better capture the complexities of energy markets.
For example, a model might combine GBM for the short-term price behavior (to reflect random unimportant wiggles in day-to-day markets) with a mean-reverting process for the long-term trend.
Real Options Analysis
This approach is used for valuing investments or projects in the energy sector, treating them as options.
It’s useful in scenarios like evaluating the feasibility of an energy project under uncertain market conditions.
How Energy Derivatives Are Created
Financial engineers design energy derivatives and structured products to provide risk management solutions and speculative opportunities for participants in energy markets, structured around the price movements of underlying energy commodities
The design process involves identifying the specific needs of market participants, such as:
- hedging against price volatility
- speculating on price movements, or
- securing a future supply of energy at a predictable cost
Financial engineers and quants design energy derivatives by developing mathematical models that accurately reflect the dynamics of energy prices.
These models account for unique characteristics of energy markets, including seasonality, mean reversion, supply and demand imbalances, and geopolitical risks.
The steps involved in creating these models typically include:
Market Analysis
Understanding the specific attributes of the energy commodity, including its volatility, price drivers, and historical price behavior.
Model Selection
Choosing appropriate mathematical frameworks, such as geometric Brownian motion for simpler derivatives or mean-reverting models for commodities like electricity that exhibit mean reversion and seasonality.
They can also combine multiple elements.
Parameter Estimation
Using historical price data to estimate the parameters of the chosen model, such as volatility, mean reversion rate, and long-term price trends.
Simulation and Testing
Employing Monte Carlo simulations or other numerical methods to simulate the behavior of the derivative under various market conditions and stress-testing against extreme scenarios.
Product Structuring
Tailoring the derivative’s features, such as strike prices, maturity, and payoff structures, to meet the hedging or speculative needs of clients.
Doing this well can facilitate more efficient and stable energy markets.
Conclusion
Each model has its strengths and limitations, and the choice depends on the:
- specific characteristics of the energy derivative being analyzed
- nature of the underlying commodity, and
- market conditions.
Advanced statistical and machine learning techniques are also increasingly being incorporated to enhance the predictive power and accuracy of these models.
Article Sources
- https://www.taylorfrancis.com/chapters/edit/10.1201/9781003265399-30/modelling-spikes-pricing-swing-options-electricity-markets-ben-hambly-sam-howison-tino-kluge
The writing and editorial team at DayTrading.com use credible sources to support their work. These include government agencies, white papers, research institutes, and engagement with industry professionals. Content is written free from bias and is fact-checked where appropriate. Learn more about why you can trust DayTrading.com