Weather Derivatives
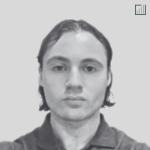
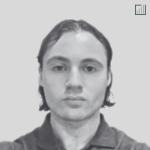
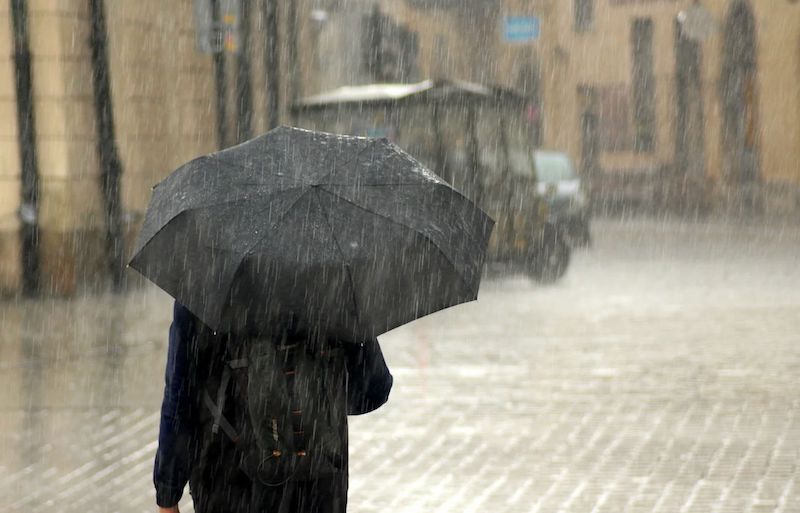
Weather derivatives are one type of derivative. They allow companies and governments to hedge their risks associated with adverse or unexpected weather conditions as part of an overall risk management strategy.
For instance, a company that produces food agricultural commodities may want to enter into a weather derivative contract to protect itself from the risk that it will not be able to sell as many crops if there are drought conditions or frost conditions impacting their harvests.
Weather derivatives are index-based instruments that typically use observed weather data to formulate an index on which a contract and associated payout can be based on.
For the crop producer, it could be based on rainfall measured over a certain period. Or the number of times it falls below a certain temperature to protect against frost damage.
In the case of weather derivatives (weather insurance), the holder of the derivative (policy) does not need to show proof of loss or demonstrate that an adverse event occurred.
The settlement is based on objective criteria – i.e., the ending value of whatever weather index was chosen for the given period. The payout(s) and settlement date is stipulated in the contract.
Uses of weather derivatives
Farmers may want to use weather derivatives in cases where:
- insufficient rain during growing periods causes poor harvests
- too much rain during harvest periods
- temperatures fall too low and cause crop damage
- strong winds destroy certain property
Utility companies may choose to use cooling degree days (CDD) or heating degree days (HDD) to help make their earnings more predictable.
Amusement parks may want to insure against rainy Saturdays or Sundays during peak months (usually during the summer) to counteract lost revenue that would be associated with them.
A management company that sets up concerts or sporting events may want to enter into a weather derivative contract to account for the possibility that it may rain on the day of the music or sporting event. If such weather transpires, not as many tickets will be sold, hurting revenue.
In each case, these entities would want to buy a weather derivative for a fixed price in exchange for a payout.
The counterparty (a bank, investor, hedge fund, etc.) would price it based on the buyer’s needs and the associated probabilities involved.
Examples of a weather derivative transaction
Example #1
For example, if a theme park has:
- 30 weekend days during its peak summer season where the bulk of its revenue is made and
- there is a 10 percent chance that it rains on each day during the summer and
- the expected loss in revenue (i.e., lower ticket sales and other revenue) from a downpour is $1 million per day
That means that the average expected loss per summer season due to adverse weather conditions would be:
30 days * 0.10 (chance of rain) * $1 million expected loss = $3 million
So they would probably want to pay around this amount (ideally under) for the weather derivative.
The counterparty underwriting the weather derivative would want also want something in this vicinity and would look to make some amount of profit on it.
After all, if there’s a 10 percent chance of rain over 30 of their highest-grossing days, it could easily rain on 5 of those days and cause an expected loss of $5 million.
Or it could rain for a longer duration of the day and cause even worse revenue losses.
It could also rain on weekdays and impact revenue on those lighter revenue days.
So the theme park could be willing to pay somewhat above the $3 million mark for insuring those 30 weekend days.
The trigger, for example, could be at least 0.25″ of rain recorded at the local weather station of the city that it’s located in.
They could also go based on levels of rainfall – 0.05″ to 0.15″ for the first payout, 0.15″ to 0.25″ for the second payout, and so on – with more rainfall equaling a higher payout to better correlate with expected revenue losses.
Even rainfall of 0.01″ could cause revenue losses, given it could dissuade some people from coming.
Example #2
Heating degree days are one of the most popular index types for weather derivative evaluation.
Common terms for an HDD contract could be:
For the October to April period, for each day that the daily mean temperature falls below a certain point (60 degrees Fahrenheit in the US, or, 16 degrees Celsius in most other countries outside the US), a total ongoing count is kept of the daily departures from this reference temperature.
The cumulative total can be used as the basis for a derivative contract that might be structured as a standard option (call or put) or as a swap that is very conceptually similar and essentially an agreement to pay or to receive payment.
History of weather derivatives
Weather derivatives are a fairly new phenomenon.
The first official weather derivative contract was made in July 1996. Consolidated Edison (ConEd) bought a commodity hedge from Aquila Energy.
It related to ConEd’s purchase of electric power from Aquila in the following month.
The deal had a weather clause in the contract that involved Aquila paying ConEd a rebate if August’s weather turned out to be colder than expected.
The measurement came in the form of cooling degree days (CDDs) based on the Central Park weather station in New York.
The weather portion of the contract basically said that if CDDs were higher than normal ConEd would receive a discount on the power price. Various levels were discounted for greater than normal departures from normal.
Soon after, weather derivatives began trading OTC.
Once they became a fixture and demand was common, the Chicago Mercantile Exchange (CME) started exchange-traded weather futures contracts along with options in 1999.
These began for various cities in the US, Canada, Europe, Japan, and Australia.
They primarily related to tracking cooling degree days or heating degree days. Rainfall and snowfall measures also were tracked.
There is also a CME Hurricane Index based on wind speed and radius of named tropical storms.
Enron Corporation was one of the first innovators in the weather derivatives space, enabling them to be traded through its EnronOnline platform.
Weather derivatives and reinsurance + portfolio diversification
Weather derivatives are closely tied to reinsurance.
Reinsurance is often considered its own asset class because of its diversification properties.
Most financial assets have to do with the credit cycle and are impacted by interest rates.
Weather, on the other hand, is different.
Things like CAT bonds (catastrophe bonds) and weather derivatives have no correlation to credit cycles and don’t involve valuation changes based on movements in interest rates.
If investors and other entities can deal in weather and reinsurance markets with positive expected value bets, it can be an uncorrelated returns stream relative to other things in their portfolio.
Things like stocks, bonds, real estate, commodities, and other types of financial assets all have the credit cycle and interest rates baked into them in some form or another.
Counterparties for these types of exotic derivatives include utility companies, individual companies, insurance firms, farming operations, individual companies, and others are looking to hedge their exposures to things that can be impacted by the weather and climate through weather derivatives.
Hedge funds, banks, and other types of traders and investors have become a common counterparty in providing this kind of protection.
Valuation of weather derivatives
Weather derivatives have no common way to value them like the Black-Scholes model for equity options or other options pricing models for other asset classes.
The underlying asset or product in weather derivatives – the weather – isn’t a good fit for these types of models.
Normally weather derivatives will be priced on some form of expected value calculation.
Some common modeling procedures:
Physical modeling of the weather
Some weather derivative pricing is based on mathematical models describing the weather system and the predictive analytics that comes out of them.
Modeling day-to-day weather is difficult past the standard 10-day timeframe. Some will involve ensemble forecasts that use Monte Carlo forecasts to derive a range of expected outcomes and probabilities within the contract period.
Statistical and physical models
Instead of only physical modeling of the weather, some weather derivatives will be priced off both statistical and physical models to help cross-check results and get more accurate readings on expected outcomes.
Historical pricing
Like many things in finance, historical pricing can provide a model for future pricing.
The historical payout of the derivative is used to find a rough approximation of what can be expected in the future.
This is simple and straightforward to do, but the estimates of what should happen may not be reliable and should only be used as an approximation.
Weather systems are complex, open systems. So lots of things are possible, which makes using historical estimates of dubious reliability.
This is much like trying to use historical financial data to inform the future. If the models are based on the past and the future is different from the past, then what you’re relying on isn’t very sound.
Composite modeling
Composite modeling is one way to price weather derivatives. This method looks at multiple different aspects of the atmosphere and climate and how they’re likely to behave.
One example would be an approach that looks at both global atmospheric wind speeds and cloud cover and uses them in a model that forecasts things like precipitation and temperature dispersion in the context of certain meteorological events.
Composite modeling takes many different factors into account when it comes to physical models that use weather data for predictive analysis.
Pricing specific to the business model
In one of the examples we did earlier in this article, we looked at business specifics related to the amusement park and how much they’d be likely to lose based on whether it rained an expected number of times.
Business pricing entails the company using weather derivative instruments to understand how its financial performance would be affected by adverse weather events and conditions across a variety of possible outcomes.
The business could plot out curves to see how its business would be impacted based on certain occurrences with the weather or in terms of the climate.
Then management can determine how much they would be willing to pay to protect against certain risks that could impact their business results considering all that’s known, the cost-benefit analysis, and how much risk they’re willing to take on.
By paying for certain derivatives they can gain greater clarity into their revenue and expenses and avoid any uncertainties that can lead to lumpier earnings.
On the other side of the trade, a bank, hedge fund, or another investor can determine how much risk they’re willing to take on and effectively extend insurance that has a positive expected payoff profile.
Index modeling
Index modeling involves building a representation of the underlying index that the derivative is based on.
An easy way to model the index is based on historical index outcomes. These can be parametrized based on known distributions or non-parametrized to allow more flexibility.
For example, for heating degree days and cooling degree days, a normal distribution is typically a good model to follow.
But the predictive ability of such a model will be constrained by that assumption and may require analyzing on a more granular scale. Daily temperature contracts and other elements (rain, snow, wind) can be built out through time series analysis.
Of course, they can get much more complicated when taking into account physical and statistical models of the global ocean-atmosphere system.