Variance
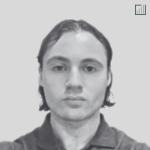
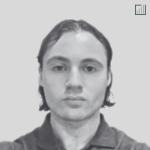
Variance plays an important role in trading and investing as it measures the degree of dispersion of returns around the mean return. I
n other words, variance indicates how much the returns of a security or portfolio deviate from its average return.
Investors and traders use variance (volatility) to estimate the risk of an investment.
A higher variance means there is more uncertainty and risk associated with the investment, while a lower variance indicates less risk.
Key Takeaways – Variance
- Variance measures the degree of dispersion of returns around the mean return of a security or portfolio.
- High variance indicates higher uncertainty and risk associated with the investment, while low variance indicates less risk.
- Traders and investors use variance to determine the optimal position size for a trade and to diversify their portfolios to manage risk.
Variance Formula
The variance formula is a mathematical expression that calculates the spread or variability of a set of data points.
It is defined as the average of the squared differences between each data point and the mean of the set.
The formula for variance is:
Variance = Σ (xi - μ)² / n
where:
- Σ is the summation symbol (meaning “sum of”)
- xi is the value of the ith data point in the set
- μ is the mean (average) value of the set
- n is the total number of data points in the set
To calculate the variance, you first need to calculate the mean of the data set.
Then, for each data point, you subtract the mean from the data point, square the difference, and add it to the sum.
Finally, you divide the sum by the total number of data points to get the variance.
The variance formula is used in many statistical calculations, such as hypothesis testing, regression analysis, and quality control.
It is also a key component in calculating other statistical measures, such as standard deviation, which is the square root of the variance.
How Is Variance Used in Trading?
In trading, variance is often used to determine the optimal position size for a trade.
By understanding the expected variance of a security or portfolio, traders can determine the amount of risk they are willing to take on and adjust their position size accordingly.
For example, if the variance is high, a trader may decide to reduce their position size to limit potential losses.
In portfolio management, variance plays a key role in diversification.
A well-diversified portfolio will have assets with different natural levels of variance, as this helps to reduce overall portfolio risk.
By combining these types of assets, investors can create a portfolio that achieves the desired level of risk while maximizing returns.
Overall, variance is a crucial measure for investors and traders, as it helps them to understand the level of risk associated with an investment and make informed decisions about portfolio management and trading strategies.
Variance vs. Standard Deviation
Both variance and standard deviation are measures of variability or spread in a set of data.
However, the standard deviation is more commonly used in trading and investing, as it’s used to measure volatility.
Variance
Variance is a statistical measure of how much the data points in a set deviate from the mean of that set.
It is calculated by finding the average of the squared differences between each data point and the mean of the set.
A higher variance indicates that the data points are more spread out and further from the mean.
Standard Deviation
Standard deviation, on the other hand, is the square root of the variance.
It is a measure of how much the data points vary from the mean of the set. Standard deviation is often used in trading and investing as a way to measure the volatility of a stock or a portfolio.
A higher standard deviation indicates that the returns of the stock or portfolio are more volatile or risky.
In trading and investing, it is important to understand the variability of returns because it can indicate the level of risk associated with a particular investment.
For example, an investor may prefer a stock with a lower standard deviation because it is less volatile and offers a more stable return over time.
However, a higher standard deviation may be preferred by a more risk-tolerant investor who is willing to accept higher volatility in exchange for potentially higher returns.
This is where traders/investors will start talking about things like “risk-adjusted returns” or ratios like the Sharpe, Sortino, Treynor, and other forms of measuring return as adjusted for risk.
Overall, standard deviation is a more useful measure in trading and investing because it provides a more intuitive understanding of the variability of returns, and it is widely used in financial models and analysis.
Types of Variance in Statistics
In statistics, variance is a measure of how spread out a set of data is. There are several types of variance that are commonly used in statistical analysis.
Here are some of the most common types of variance:
Population variance
Population variance is the variance of an entire population.
It measures the spread of the data in the population.
Sample variance
Sample variance is the variance of a sample of data.
It is used to estimate the population variance.
Since the sample is typically smaller than the population, the sample variance will be different from the population variance.
Within-group variance
Within-group variance is the variance within a group of data.
It is used in analysis of variance (ANOVA) to determine if there are significant differences between groups.
Between-group variance
Between-group variance is the variance between groups of data.
It is also used in ANOVA to determine if there are significant differences between groups.
Residual variance
Residual variance is the variance that remains after a model has been fit to the data. It measures the extent to which the model is able to explain the variability in the data.
Each of these types of variance has its own specific use in statistical analysis. It is important to choose the appropriate type of variance for the analysis you are performing to ensure accurate and meaningful results.
Variance vs. Covariance
Variance and covariance are both important concepts in trading and finance, as they help quantify the volatility and correlation of assets in a portfolio.
Variance measures the variability of returns for a single asset, while covariance measures the joint variability of returns for two assets.
In trading, variance is often used to measure volatility, orthe degree of variation of an asset’s returns over time.
Volatility is an important factor for traders because it affects the risk and potential reward of an investment.
A higher variance indicates a greater degree of volatility and risk, while a lower variance indicates less volatility and risk.
Covariance, on the other hand, measures how two assets move together. A positive covariance indicates that the two assets tend to move in the same direction, while a negative covariance indicates that they tend to move in opposite directions.
Covariance is important for traders because it helps them to diversify their portfolio by identifying assets that are not strongly correlated with each other. This can help reduce overall risk and increase potential returns.
In short, variance measures the volatility of a single asset, while covariance measures the correlation between two assets. Both are important concepts in trading and finance and can be used to manage risk and increase potential returns in a portfolio.
Mean-Variance Tradeoff
The mean-variance tradeoff is a fundamental concept in finance that refers to the tradeoff between the expected return and the risk (or variance) of an investment.
In other words, investors face a choice between taking on more risk to potentially earn higher returns or accepting lower returns in exchange for a lower level of risk.
In the context of trading and investing, the mean-variance tradeoff is often used to construct portfolios that optimize the balance between risk and return.
Modern Portfolio Theory, developed by Harry Markowitz, provides a framework for determining the optimal portfolio that achieves the highest expected return for a given level of risk.
This theory assumes that investors are risk-averse and prefer portfolios that minimize risk for a given level of return or maximize return for a given level of risk.
In practice, investors use tools such as the Capital Asset Pricing Model (CAPM) and the Efficient Frontier to identify the optimal portfolio allocation.
The CAPM uses the expected return of the market, risk-free rate, and the beta of the security to calculate the expected return of an asset, whereas the Efficient Frontier identifies the set of portfolios that offer the highest expected return for a given level of risk.
Investors must consider the mean-variance tradeoff in order to make informed investment decisions.
High-risk, high-return investments may be appropriate for some investors, while others may prefer lower-risk, lower-return investments.
A well-diversified portfolio that includes a mix of assets with different levels of risk can help to achieve a more optimal balance between risk and return.
Variance vs. Expected Value
In trading and investing, variance and expected value are both important concepts that help investors make informed decisions about their investments.
Expected value (EV) is a measure of the average outcome of a particular investment or trading strategy.
It represents the sum of the probability-weighted outcomes of all possible scenarios.
For example, if an investor has a 60% chance of earning a 10% return on an investment and a 40% chance of losing 5%, the expected value of the investment would be:
(0.6 * 0.10) + (0.4 * -0.05) = 0.06 – 0.02 = 0.04, or a 4% expected return.
Variance measures the dispersion or spread of possible outcomes around the expected value.
A high variance indicates that the actual outcome is likely to deviate significantly from the expected value, while a low variance indicates that the actual outcome is likely to be close to the expected value.
In other words, variance is a measure of the risk associated with an investment or trading strategy.
For example, if two investments have the same expected return of 10%, but one has a high variance and the other has a low variance, the high variance investment is riskier because its actual returns are likely to be more spread out and unpredictable.
Investors and traders use both expected value and variance to evaluate and compare investment opportunities.
They may prefer investments with a high expected value and low variance, indicating that the investment has the potential to generate significant returns while also being relatively predictable.
However, some investors may be willing to take on higher risk and accept a higher variance for the potential of higher returns.
Gamified Example of Variance vs. Expected Value
Let’s say you’re playing a game with three options:
- Option 1: 80% chance of winning $10,000
- Option 2: 30% chance of winning $100,000
- Option 3: 10% chance of winning $1,000,000
Your expected values in each situation are:
- $8,000
- $30,000
- $100,000
Would you sacrifice EV for lower variance?
It can be perfectly reasonable.
The type of person who would select each option based on their risk tolerance and financial situation would vary depending on their individual preferences, financial goals, and attitudes toward risk.
Option 1
An individual who is risk-averse and has a lower financial risk capacity may select option 1 because it offers a higher probability of winning, albeit at a lower reward.
This person may prioritize the security of a more guaranteed payout and would be willing to sacrifice the possibility of winning a larger sum of money to avoid the risk of losing it all.
Option 2
An individual who has a moderate level of risk tolerance and has a higher financial risk capacity may select option 2 because it offers a higher potential reward for taking on more risk.
This person may be willing to accept a lower probability of winning in exchange for the potential of winning a higher sum of money.
However, this person may still want to limit their exposure to risk and may not be willing to risk everything for a chance at a large payout.
Option 3
An individual who is highly risk-tolerant and has a high financial risk capacity may select option 3 because it offers the highest potential reward, despite having the lowest probability of winning.
This person may be willing to take on a higher level of risk in exchange for the possibility of winning a much larger sum of money.
However, this person must be prepared to accept the risk of losing everything for a chance at a much larger payout.
It is worth noting that these are just generalizations, and people’s risk tolerance and financial situation can vary greatly based on their personal circumstances, financial goals, and psychological makeup.
How would you design this game to get one-third of a statistically significant sample size on each option?
This would take trial and error.
You would need enough participants to ensure statistical significance as well as a truly randomized sample.
For example, if you choose new college graduates, you can bet that there would be a plurality of the votes on the first option because an 80% chance of having $10,000 is more valuable to them than swinging for the fences on a bigger amount they’re likely to not have.
On the other hand, if your sample is successful business people, the first option might be underrepresented because $10,000 might not be that big of a deal to them.
If you had a randomized sample with a statistically significant number of votes and found that Option 1 was dominating, you could redesign the game to something like:
- Option 1: 80% chance of winning $10,000
- Option 2: 50% chance of winning $100,000
- Option 3: 20% chance of winning $1,000,000
Your expected values in each situation would then be:
- $8,000
- $50,000
- $200,000
This process of trial and error is analogous to how markets work in the real world.
The “clearing price” would be one that gets a third of the votes on each.
In financial markets, this might be analogous to owning safe Treasury bonds vs. owning equities or riskier forms of credit vs. owning venture capital.
As you go up the risk spectrum, you generally have higher expected value, but at the trade-off of higher variance or higher risk of loss or failure.
Variance – Clearly Explained (How To Calculate Variance)
FAQs – Variance
Is variance the same thing as volatility?
Variance and volatility are related concepts, but they are not exactly the same thing.
Variance is a statistical measure that quantifies the spread of a set of data points around the mean or average.
It is calculated as the average of the squared differences from the mean.
In finance, variance is often used to measure the risk of a portfolio or an individual security.
Volatility, on the other hand, is a measure of the degree of variation of a financial instrument’s price over time.
It can be calculated using different methods, but one common approach is to use the standard deviation of the instrument’s returns over a certain period.
Volatility is often used as a proxy for risk (or a component of risk), as higher volatility implies a greater degree of uncertainty or potential for large price movements.
In finance, volatility and variance are often used interchangeably, but they are not exactly the same thing.
Variance is a mathematical concept that is often used as a building block for calculating volatility, but volatility is a more specific measure that takes into account the time series of prices or returns.
What is the bias-variance tradeoff?
The bias-variance tradeoff is a fundamental concept in machine learning that refers to the tradeoff between the ability of a model to fit the training data well (low bias) and its ability to generalize to new, unseen data (low variance).
Bias is the difference between the predicted values of a model and the true values of the data.
A model with high bias is typically oversimplified and cannot capture the complexity of the data, leading to underfitting.
In other words, the model is not able to learn the underlying patterns in the data and its predictions are consistently wrong.
Variance is the variability of the model’s predictions for different training datasets.
A model with high variance is typically too complex and has overfit the training data, resulting in a model that is not able to generalize well to new data.
In other words, the model has memorized the training data and is unable to apply what it has learned to new data.
The goal of machine learning is to find the optimal tradeoff between bias and variance, by selecting a model that is sufficiently complex to capture the underlying patterns in the data but not so complex that it overfits the training data.
This can be achieved by selecting an appropriate model complexity, using regularization techniques to penalize complex models, or using ensemble methods to combine multiple models with different biases and variances.
What is a variance swap?
A variance swap is a financial contract that allows investors to bet on the level of volatility in the price of an underlying asset, such as a stock, an index, or a commodity.
The contract is typically structured as an over-the-counter (OTC) derivative and involves a payment based on the difference between the realized variance of the asset’s price over a specified period of time and a pre-agreed level of variance, known as the strike variance.
In a variance swap, the buyer agrees to pay a fixed amount of money to the seller in exchange for a payment based on the difference between the realized variance and the strike variance.
If the realized variance is higher than the strike variance, the seller will make a payment to the buyer, and vice versa.
Variance swaps are commonly used by investors who are interested in taking a view on the volatility of an asset, rather than its direction.
They can be used for hedging or speculative purposes, and are often used by market makers and institutional investors.
Variance swaps can be customized to meet the specific needs of the buyer and seller, such as the underlying asset, the strike variance, and the length of the contract.
Conclusion – Variance
Variance is a statistical measure that quantifies the spread or dispersion of a set of data points around their mean or expected value.
In trading and investing, variance is an important concept that helps market participants understand the risk associated with their investments.
A high variance indicates that the actual returns are likely to deviate significantly from the expected value, making the investment riskier, while a low variance indicates that the actual returns are likely to be closer to the expected value, making the investment less risky.
Investors use variance to evaluate and compare investment opportunities and to make informed decisions about their portfolios.