Factor Risk Models
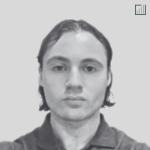
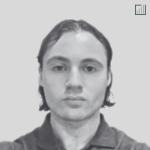
Factor risk models are used by traders and investors who need to estimate the relationship and riskiness of securities.
These tools are especially helpful in building the covariance matrix of assets in a portfolio.
Essentially, the covariance matrix measures the movements of returns from different assets and how they correspond with one another.
This information is used to gauge the risk level of a portfolio based on how the assets within it relate.
Let’s look more into factor risk models and their use in portfolio management.
Key Takeaways – Factor Risk Models
- Factor risk models help traders/investors estimate the relationship and riskiness of securities, particularly in portfolio management.
- They often involve using the covariance matrix to measure the movements of returns among different assets and determine the portfolio’s risk level.
- There are two main types of factor risk models: statistical and fundamental.
- Statistical models use historical data to identify key factors influencing asset returns, while fundamental models rely on economic understanding to explain asset return behavior.
- Popular factor risk models include the BARRA model, which combines statistical and fundamental factors, and the Fama-French three-factor model, which focuses on market risk, company size, and the book-to-market ratio.
- Factor risk models provide insights into portfolio risk management and optimization but may have limitations in predicting future returns during unpredictable market events.
Two Main Types of Factor Risk Models
Factor risk models are largely categorized into two types: statistical and fundamental factor risk models.
Statistical models use historical data to identify the key factors that influence asset returns.
On the other hand, fundamental factor risk models use economic theories to discern the elements that affect asset returns.
These models vary in their approaches but offer useful insights for traders/investors looking to understand the risks associated with their portfolios.
Statistical Factor Risk Models
Statistical factor risk models are built around historical data.
The assumption is that past performance and trends of assets can predict future outcomes.
These models help in understanding the factors that have previously influenced asset returns, providing a predictive angle for future returns.
But, of course, the future can be different from the past. So while they can be useful to learn from, they shouldn’t be used in isolation.
Fundamental Factor Risk Models
In contrast to statistical models, fundamental factor risk models lean on economic theory to point out the factors that drive asset returns.
Factors such as inflation, GDP growth, and corporate earnings often play a key role in these models.
While these models might not always offer a definitive predictive perspective, they are robust in explaining why certain asset returns behave the way they do.
Popular Factor Risk Models: BARRA and Fama-French
Among the various factor risk models, the BARRA risk model and the Fama-French three-factor model stand out.
BARRA
The BARRA model is a multivariate regression-based model that uses both statistical and fundamental factors.
It provides a framework for assessing portfolio risk by analyzing the impact of various factors, including market risk, interest rates, and industry-specific variables.
Fama-French
The Fama-French three-factor model, on the other hand, is a fundamental factor model.
It identifies three key factors affecting portfolio returns: market risk, company size, and the book-to-market ratio.
Fama French Three Factor Model
Advantages and Disadvantages of Factor Risk Models
Like any other tool, factor risk models come with their pros and cons.
On the one hand, they provide traders/investors with an understanding of the potential risks and relationships between assets.
This understanding is important in risk management and in optimizing portfolio construction.
On the other hand, factor risk models may fall short when it comes to predicting future returns, particularly in the case of black swan/tail events or unpredictable market shocks.
For instance, statistical models may not adequately capture changes in market dynamics, while fundamental models may oversimplify the complexities of real-world economics.
FAQs – Factor Risk Models
How to interpret SMB and HML in factor risk models?
In factor risk models, SMB (Small Minus Big) and HML (High Minus Low) are two common factors used to explain the cross-section of stock returns.
These factors were first introduced in the Fama-French three-factor model and have since been widely used in finance research.
Here’s how SMB and HML can be interpreted in factor risk models:
- SMB (Small Minus Big): SMB captures the effect of the size of companies on their returns. It measures the excess returns of small-cap stocks over large-cap stocks. A positive SMB indicates that small-cap stocks outperformed large-cap stocks, while a negative SMB suggests the opposite. This factor is often interpreted as a proxy for the risk associated with investing in smaller companies, as they tend to have higher market beta and higher idiosyncratic risk.
- HML (High Minus Low): HML captures the effect of the book-to-market ratio (value) of companies on their returns. It measures the excess returns of high book-to-market (value) stocks over low book-to-market (growth) stocks. A positive HML indicates that value stocks outperformed growth stocks, while a negative HML suggests the opposite. This factor is often interpreted as a proxy for the risk associated with investing in value stocks, as they tend to have higher financial distress risk (e.g., higher debt, mismanagement, obsolescence) and higher systematic risk.
Both SMB and HML are considered risk factors because they capture the systematic sources of risk that are not diversified away in a well-diversified portfolio.
The interpretation of these factors is based on the historical relationship between the factor returns and the returns of different portfolios sorted by company size (for SMB) and book-to-market ratio (for HML).
How do you calculate SMB and HML factors?
To calculate the SMB (Small Minus Big) and HML (High Minus Low) factors, you would typically follow a three-step process:
Sort the universe of stocks
Start by sorting the universe of stocks based on two characteristics: market capitalization for SMB and book-to-market ratio for HML.
- For SMB, you would divide the stocks into two groups: small-cap and large-cap. The specific cutoff for defining small-cap and large-cap stocks can vary, but a common approach is to use the median market capitalization as the threshold.
- For HML, you would divide the stocks into two groups: value and growth. Value stocks typically have a high book-to-market ratio (indicating they are relatively undervalued), while growth stocks have a low book-to-market ratio (indicating they are relatively overvalued). The specific cutoff for defining value and growth stocks can vary, but a common approach is to use the median book-to-market ratio as the threshold.
Calculate the portfolio returns
Once you have sorted the stocks into their respective groups, you calculate the returns of the portfolios representing each factor.
- For SMB, you would calculate the excess returns of the small-cap portfolio over the large-cap portfolio. Excess returns can be computed by subtracting a risk-free rate (such as the Treasury bill rate) from the actual returns of each portfolio.
- For HML, you would calculate the excess returns of the value portfolio over the growth portfolio. Again, excess returns are calculated by subtracting the risk-free rate from the actual returns of each portfolio.
Determine the factor returns
Finally, you calculate the factor returns by taking the average excess returns of the portfolios for each factor over a specific time period.
- For SMB, the factor return is the average excess return of the small-cap portfolio minus the average excess return of the large-cap portfolio.
- For HML, the factor return is the average excess return of the value portfolio minus the average excess return of the growth portfolio.
These factor returns can then be used in factor risk models, such as the Fama-French three-factor model, to explain the cross-section of stock returns and analyze the risk and performance of different portfolios.
How are factor risk models related to factors like value, size, momentum, etc.?
Factor risk models are closely related to factors like value, size, momentum, and others.
These factors represent specific characteristics or attributes of assets that have been found to influence their expected returns.
As we’ve covered above, factor risk models aim to explain the cross-section of asset returns by identifying and quantifying the impact of these factors.
By including these factors in the model, analysts can better understand the risk and return dynamics of a portfolio or security.
For example:
- Value Factor: Value refers to the price relative to a fundamental measure, such as the book-to-market ratio. The value factor captures the excess returns of value stocks over growth stocks. In a factor risk model, the value factor is used to explain the performance differences between companies with different valuation characteristics.
- Size Factor: The size factor captures the excess returns of small-cap stocks over large-cap stocks. It represents the effect of company size on stock returns. In a factor risk model, the size factor helps explain the risk and return patterns associated with investing in smaller or larger companies.
- Momentum Factor: Momentum captures the tendency of assets that have performed well (or poorly) in the recent past to continue performing well (or poorly) in the future. The momentum factor captures the excess returns of assets with positive momentum over those with negative momentum. In a factor risk model, the momentum factor helps explain the persistence of price trends and trader behavior.
Factor risk models combine multiple factors to capture the different sources of risk and return in a portfolio.
By considering these factors, analysts can gain insights into the specific drivers of portfolio performance, evaluate risk exposure, and make better decisions.
Conclusion
Despite their limitations, factor risk models remain a tool for traders/investors in understanding the riskiness and relationships between securities.
By leveraging both statistical and fundamental factor risk models, market practitioners can gain a holistic perspective on portfolio risk, which can subsequently inform more effective and strategic decision-making.