Risk Measure
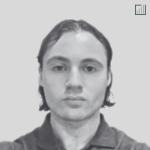
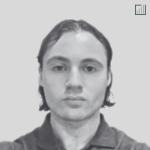
Risk management plays a critical role in ensuring the stability of financial institutions and mitigating potential losses.
One essential tool for managing these risks is the risk measure.
Risk measures are mathematical models used to quantify and manage the risks taken by banks, insurance companies, and other financial institutions.
The aim is to determine the amount of reserve to be maintained to meet regulatory requirements and protect against unexpected losses.
We look into various types of risk measures, including coherent, deviation, distortion, and spectral risk measures, as well as the popular Value-at-Risk (VaR) method and its subvariants, Expected Shortfall and Entropic Value-at-Risk.
Key Takeaways – Risk Measure
- By implementing appropriate risk measures and strategies, institutions can better protect themselves against unexpected events and meet regulatory requirements.
- Coherent risk measures provide accurate risk representation: Coherent risk measures, which adhere to specific axioms, offer a more accurate representation of potential risks. They consider factors such as diversification, risk-free assets, and risk scaling, enabling better risk management decisions and more precise quantification of risks.
- Different risk measures address specific aspects of risk: There is a wide range of risk measures available, each designed to capture different dimensions of risk.
- Deviation measures focus on variability and downside risk, distortion measures incorporate risk aversion, and spectral measures combine coherent and distortion principles.
- Understanding the characteristics and applications of these measures helps institutions tailor their risk assessment and management approaches.
Coherent Risk Measure
A coherent risk measure is a type of risk measure that adheres to four key axioms:
- monotonicity
- subadditivity
- positive homogeneity, and
- translational invariance
Monotonicity means that if one portfolio has a lower risk than another, the risk measure should reflect that.
Subadditivity states that the risk of combined portfolios should be less than or equal to the sum of their individual risks, promoting diversification.
Positive homogeneity implies that the risk measure scales linearly with the size of the position.
And translational invariance ensures that the risk measure accounts for the impact of risk-free assets.
Coherent risk measures are desirable because they provide a more accurate representation of the potential risks and enable better risk management decisions.
Deviation Risk Measure
Deviation risk measures focus on the deviation of an asset’s returns from its expected value.
In essence, they quantify the uncertainty or variability in returns.
Some common deviation risk measures include standard deviation, semi-deviation, and lower partial moments.
Standard deviation measures the average deviation from the mean, while semi-deviation considers only the downside deviation, which is particularly useful for traders or investors seeking to minimize losses.
Lower partial moments capture downside risk by considering deviations below a specified threshold.
Deviation risk measures are widely used to assess portfolio risk and optimize asset allocation.
Distortion Risk Measure
Distortion risk measures, also known as distortion risk-averse measures, are a class of risk measures that incorporate an investor’s risk aversion by applying a distortion function to the cumulative distribution function (CDF) of the asset returns.
The distortion function modifies the CDF, emphasizing the impact of undesirable outcomes based on the investor’s risk aversion level.
Distortion risk measures offer a flexible approach to risk measurement, as they can be tailored to reflect the specific risk preferences of different traders/investors or institutions.
Spectral Risk Measure
Spectral risk measures combine the principles of coherent risk measures and distortion risk measures.
They are derived from a weighted average of Expected Shortfalls, where the weights are determined by a user-defined risk-aversion function called the spectral function.
This approach ensures that spectral risk measures satisfy the axioms of coherent risk measures while allowing for trader-/investor-specific customization through the spectral function.
Spectral risk measures provide a comprehensive and customizable risk assessment tool that can be tailored to various risk management objectives.
Value-at-Risk (VaR)
Value-at-Risk (VaR) is a widely used risk measure that estimates the maximum potential loss of a portfolio over a specific time horizon and at a given confidence level.
VaR provides a single number that encapsulates the risk of an investment, making it a popular choice for risk managers and regulators.
However, VaR has some limitations, such as its lack of subadditivity and inability to capture tail risk.
To address these limitations, additional measures have been developed, including Expected Shortfall and Entropic Value-at-Risk.
Expected Shortfall
Conditional Value-at-Risk (CVaR)
Expected Shortfall (ES), also known as Conditional Value-at-Risk (CVaR), is an extension of VaR that focuses on the tail risk of a portfolio.
It estimates the expected loss of a portfolio in the worst-case scenarios, specifically those beyond the VaR threshold.
ES is considered a coherent risk measure as it satisfies the four axioms mentioned earlier.
It provides a more comprehensive assessment of risk compared to VaR, as it takes into account the severity of potential losses in extreme scenarios, which is important for effective risk management.
Entropic Value-at-Risk
Entropic Value-at-Risk (EVaR) is another alternative to VaR that addresses some of its limitations.
EVaR is derived from information theory and is defined as the minimum amount of additional capital required to maintain the same risk level when the portfolio’s risk is increased by adding a small amount of a highly risky asset.
EVaR is also a coherent risk measure and has some desirable properties, such as subadditivity and robustness to model misspecification.
It provides a more accurate representation of tail risk and is less sensitive to estimation errors, making it a valuable tool for risk managers and regulators.
Superposed Risk Measures
Superposed risk measures combine two or more risk measures to create a new risk measure that captures the desired characteristics of the original measures.
The superposition is usually achieved by taking a linear or convex combination of the underlying risk measures, allowing for flexibility and customization based on the specific needs of the financial institution.
It can be thought of as a “risk index” that better captures multiple statistics rather than just one.
Superposed risk measures can provide a more comprehensive assessment of risk by incorporating the strengths of multiple measures, resulting in a more accurate and robust representation of the potential risks faced by a portfolio.
Drawdown
Drawdown is a risk measure that focuses on the maximum decline in the value of an investment or portfolio from its peak to its lowest point over a specified period.
Drawdown is particularly relevant for traders, investors, and portfolio managers who are concerned with the preservation of capital and managing the potential for significant losses.
By quantifying the worst-case scenario of an investment, drawdown helps investors assess the risk-reward trade-off and make more informed investment decisions.
Tail Conditional Expectation
Tail Conditional Expectation (TCE), also known as Tail Conditional Mean or Tail Value-at-Risk, is a risk measure that focuses on the expected loss given that a specified extreme event occurs.
TCE is calculated by taking the average of the potential losses that exceed a certain threshold, such as the Value-at-Risk.
By emphasizing the potential losses in the tail of the return distribution, TCE provides a more comprehensive assessment of tail risk and helps financial institutions better understand the severity of potential losses during extreme events.
Superhedging Price
The superhedging price is a risk measure related to the concept of hedging in finance.
It represents the minimum initial investment required to construct a self-financing hedging strategy that ensures the investor’s liability is covered, regardless of future market scenarios.
The superhedging price takes into account transaction costs, market frictions, and the potential for model misspecification, providing a worst-case estimate of the cost of hedging a given financial position.
This risk measure is particularly useful for risk managers, portfolio managers, and regulators, as it helps assess the adequacy of hedging strategies and the potential capital requirements for covering liabilities.
What would be an example?
One example of a self-financing hedging strategy is known as a collar strategy.
In a collar strategy, a trader/investor aims to protect the value of an underlying asset in their portfolio while generating income to offset the hedging costs.
Here’s an example of how a collar strategy can be implemented:
- Assume an investor holds 1,000 shares of a company’s stock, which is currently trading at $100 per share. The investor wants to protect against potential downside risk but is also willing to limit potential upside gains.
- To establish the collar, the investor simultaneously performs the following actions:
a. Sell Call Options: The investor sells 10 call options contracts with a strike price of $110, expiring in three months. By selling the call options, the investor receives a premium, which helps offset the cost of the protective put options.
b. Buy Put Options: The investor purchases 10 put options contracts with a strike price of $90, expiring in three months. The put options provide downside protection by allowing the investor to sell the shares at the strike price, even if the stock price drops below that level.
- By implementing this collar strategy, the investor has created a protective range for their portfolio. The call options sold will limit potential gains if the stock price exceeds the strike price of $110. However, the put options purchased provide downside protection if the stock price falls below the strike price of $90.
- As the collar strategy is self-financing or close to it (puts generally cost more than calls in the equity markets due to the demand for protection), the premium received from selling the call options helps offset the cost of buying the put options. Ideally, the premium received is enough to cover the cost or even provide a small net credit.
The self-financing collar strategy allows the trader/investor to limit potential losses if the stock price declines, while still participating in potential gains within a specified range.
The trader/investor can benefit from income generated by selling call options while minimizing the overall cost of hedging.
Expectile
Expectile is a risk measure that generalizes the concept of quantiles to capture the entire range of potential outcomes in a portfolio’s return distribution.
The expectile is defined as the point that minimizes the asymmetric weighted sum of the squared deviations between the observed returns and the expectile itself.
This measure provides a more comprehensive view of the risk profile, as it captures both the central tendency and the dispersion of the return distribution.
Expectiles are particularly useful for risk-sensitive investors, as they can be tailored to emphasize specific regions of the return distribution based on the investor’s risk preferences.
By exploring a variety of risk measures, financial institutions can better understand the complex nature of risk in their portfolios and make more informed decisions regarding risk management.
Each risk measure offers unique insights and addresses different aspects of risk, making it crucial for practitioners to choose the most appropriate measure based on their specific needs and objectives.
Conclusion
Risk measures play an important role in finance, enabling traders and institutions to quantify and manage their exposure to risk.
The various risk measures discussed, including coherent, deviation, distortion, spectral, and Value-at-Risk, each have their unique advantages and limitations.
By understanding the nuances of these measures and selecting the most appropriate one(s) based on the specific context, financial institutions can make more informed risk management decisions, ensuring their stability and resilience over time.