Why the Zillow Zestimate (And Other AVMs) Are Flawed
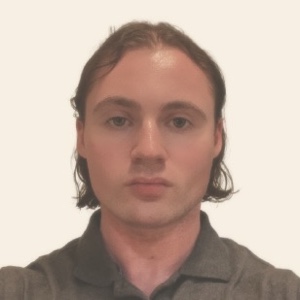
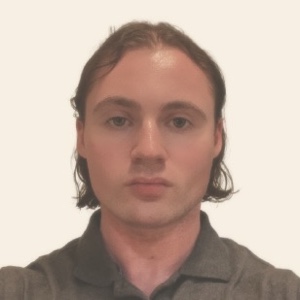
The Zillow Zestimate and other Automated Valuation Models (AVMs) like it are designed to provide an estimate of a property’s market value using algorithmic modeling.
These models typically rely on a combination of publicly available data, historical transaction data, and various computational techniques.
They involve statistical analysis (like regressions) and often basic machine learning algorithms.
However, there are several reasons why these estimates might not always be accurate.
Key Takeaways – Why the Zillow Zestimate (And Other AVMs) Are Flawed
- Data Quality and Timeliness: AVMs like Zillow’s Zestimate suffer from reliance on potentially outdated or poor-quality data.
- Overlooking Local and Property Specifics: These models often miss local market nuances and unique property features (like renovations), important for precise valuation.
- Limitations of Algorithmic Modeling: AVMs struggle with the non-linear dynamics of real estate valuation, such as the variable impact of a certain feature, and can’t replicate the qualitative judgments of human appraisers, leading to discrepancies in estimated and actual values.
Data Limitations
AVMs depend heavily on the quality and recency of the data they use.
In real estate, data can quickly become outdated due to rapid changes in market conditions.
If an AVM is not regularly updated with new data, its accuracy can suffer.
Local Market Nuances
Real estate markets are highly localized and can vary significantly even within the same city.
AVMs, which often use broad datasets, may not capture these local nuances effectively.
This can lead to inaccuracies in specific neighborhoods or for particular types of properties.
Property Specifics
AVMs may not have detailed information about the unique features of a property that can significantly affect its value, such as recent renovations, interior designs, or unpermitted modifications.
These models typically rely on general characteristics like:
- square footage
- number of bedrooms and bathrooms, and
- location
This might not fully capture a property’s unique value.
In a separate article, we looked at the wide nuance in how a swimming pool might affect a property’s valuation – because it’s highly dependent on the specific type of buyer.
Human Judgment Factor
Real estate valuation is not just quantitative, but often involves qualitative judgment by experienced professionals.
Factors such as curb appeal, neighborhood feel, and interior aesthetics are difficult to quantify and incorporate into an algorithmic model.
Market Dynamics
Real estate markets are influenced by a variety of economic, social, and political factors that can change rapidly.
AVMs might not be agile enough to incorporate these changes in real-time.
This can lead to discrepancies between estimated values and actual market prices.
Algorithmic Complexity
The algorithms used in AVMs have inherent limitations.
They may not account for non-linear relationships or interactions between variables that a human appraiser might intuitively understand.
Example
As an example, the algorithm might linearly correlate square footage and property value.
However, it could miss complex interactions like:
- the diminishing return of additional square footage in certain areas or
- the impact of neighborhood gentrification on property values
These are insights a human appraiser might intuitively grasp.
An AVM is a fairly generic, out-of-the-box system that misses out on a wide range of factors.
Standardization
Standardization issues in AVMs arise due to the inconsistent formatting and representation of data across different sources.
Properties can vary significantly in features and descriptions.
This makes it challenging to align and compare them uniformly in a model.
For instance, a “renovated kitchen” can mean different things in different listings. This affects how such features are quantified and compared.
If a homeowner remodels their kitchen or bathroom or any part of the house, the AVM doesn’t know that and they don’t get credit for whatever that entails.
It’s just a bot that’s fed whatever it’s fed.
The lack of a standardized framework for data representation in real estate contributes to difficulties in achieving precise valuations through automated models.
Conclusion
AVMs like Zillow’s Zestimate provide a useful starting point for understanding property values, but their estimates should be taken with caution.
They’re essentially marketing tools.
They’re best used as one of several tools in assessing a property’s value, complemented by professional appraisals and an understanding of the local market.
There is a path to using AVMs for property assessment in a robust way – i.e., in a way where one could actually form a business model flipping/trading/investing in homes in the context of an adversarial market – but it’s more complicated than using basic regressions and statistical techniques.