Why Zillow iBuying Failed (Zillow Offers)
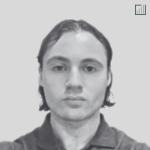
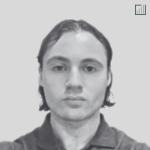
Zillow, the prominent online real estate marketplace, attempted to venture into the home “iBuying” industry with an Automated Valuation Model (AVM) designed to purchase homes they perceived as undervalued.
Using a robust dataset gathered over a span of time and an artificial intelligence-driven approach, they aimed to create a robust real estate buying model (officially termed Zillow Offers).
Unfortunately, their ambitious endeavor ultimately resulted in a massive failure.
This case serves as a stark example of how even a company rich in data can misapply artificial intelligence, leading to bad consequences.
We’ll explain the various angles of why Zillow iBuying failed.
Key Takeaways – Why Zillow iBuying Failed
- Zillow’s ambitious attempt to use an AI-driven approach for home “iBuying” (Zillow Offers) failed partly due to its AI’s inadequate understanding of macroeconomic cycles, a critical aspect of real estate investing.
- The AI model lacked the ability to account for local factors and hidden property issues, leading to overpaying for properties and subsequent financial losses for Zillow.
- Zillow’s failure serves as a cautionary example of the dangers of misapplying AI in complex fields like real estate, emphasizing the importance of a more profound understanding of cause-effect relationships and industry nuances when leveraging AI technologies.
The AI Technique: Ambitious, But Inadequate
Zillow deployed an AI technique to power its iBuying program, primarily focusing on identifying and purchasing undervalued homes.
The process essentially worked like this:
- Online Evaluation: Homeowners entered their home details on the Zillow website. This included the address and any relevant information about the home’s condition, upgrades, and features. Zillow then used this information along with its extensive database on comparable sales and real estate data to make an initial offer.
- Algorithm-Based Valuation: Zillow’s offer was primarily based on an Automated Valuation Model. This model used an algorithm that takes into account hundreds of data points, including recent sales of similar homes in the same area, market trends, property features, and more. Naturally, it was largely regression-based, taking various data points and fitting a valuation to them.
- Human Review and Inspection: If the homeowner decided to proceed with the initial offer, Zillow then sent an independent contractor or a Zillow employee to perform a home inspection. This inspector evaluated the home’s condition, checked for any necessary repairs, and confirmed details about the home. The initial offer would be adjusted based on the results of this inspection.
- Final Offer: After the home inspection, Zillow will finalize its offer. This included taking into account any necessary repairs and adjusting the offer to reflect these costs. If the homeowner agreed to this final offer, they can then proceed with the sale to Zillow Offers.
The algorithm they used was excellent at spotting trends and adjusting to seasonality changes.
However, one of the fatal flaws in their system was the AI’s inability to understand and react to macroeconomic cycles, a significant aspect of real estate investing.
Though there was a human element working for Zillow via the inspection, it was still Zillow’s Automated Valuation Model competing against human participants in an adversarial market.
Overlooking the Impact of Macroeconomic Cycles
Real estate markets are heavily influenced by macroeconomic cycles.
These cycles, which reflect the overall economic climate, can significantly impact property values.
Yet, Zillow’s AI model didn’t consider these broad market factors.
The omission of this critical factor was a significant oversight in Zillow’s model, reflecting the issues of overly simplistic statistical methods in valuing any market.
Practical Limitations of The AI Approach
Zillow’s approach to the market was, in essence, almost a purely statistical one, lacking a reasoning engine to interpret and respond to market nuances.
When put into practice, the algorithm consistently underperformed.
Within the context of an adversarial market, their AI system often overpaid for properties because it lacked insights that other market participants had.
This knowledge gap became an Achilles’ heel for Zillow, as it found itself on the losing side of transactions and overpaying for properties.
Adversarial Market: A Core Challenge
The adversarial aspect of real estate markets poses a significant challenge to the AI model.
Whenever Zillow made a successful purchase, it often meant that other investors had passed on the property, possibly due to issues with the property that the statistical bot simply could not identify.
Without a more nuanced understanding of each property’s unique features and potential problems, Zillow’s AI was at a significant disadvantage.
Example
If a home has a swimming pool, it’s unknown what kind of value Zillow’s AI would ascribe to it, if any.
However, for a human buyer, there can be a lot of variance.
Some might value it a lot.
Or they might not value it at all for a variety of reasons, such as:
- cost of maintenance and increased operating costs of the property
- insurance and liability
- limited appeal for many buyers (e.g., a safety risk, an unnecessary luxury, or an unwanted responsibility)
- personal preferences (style, size, shape, depth, or location of the pool might not suit every potential buyer’s preferences – e.g., a buyer might want a deeper pool for diving, a longer pool for swimming laps, or a different style or location for aesthetic reasons)
- climactic conditions affect its usability
- space utilization (some potential buyers may prefer to utilize the space taken up by a pool in other ways, such as landscaping, gardening, or adding other outdoor amenities)
While a swimming pool can be an attractive feature for some buyers, it may not always add to the overall value of a home.
Instead, it can complicate the selling process by limiting potential buyers, adding to maintenance and insurance costs, and requiring specific conditions and preferences to be met; thus, potentially devaluing the property.
The value humans ascribe to such features can be very wide in a positive or negative way.
For an AI program, they generally aren’t sophisticated enough to accurately assess all the various nuances of a feature like this, let alone all that goes into a home.
Consequences of Misapplying AI
Zillow’s iBuying failure underscores the dangers of misapplying AI, particularly in complex fields like real estate.
Even with a vast amount of data, the absence of a reasoning engine to understand cause-effect relationships led to significant missteps.
Zillow’s approach was too simplistic for the task at hand, leading to substantial losses (more than $1 billion in a few years) and ultimately, the failure of their Zillow Offers venture.
The Path Forward: Understanding Cause-Effect Linkages
The failure of Zillow’s iBuying program demonstrates the importance of a deeper and more nuanced understanding of cause-effect linkages in real estate investing.
Any successful approach must account for complex factors such as the macroeconomic cycle and property-specific issues that can significantly influence property values.
Only then can AI be appropriately utilized in the home flipping.
This lesson from Zillow’s experience serves as a reminder for other firms looking to leverage AI in complex industries, including in traditional equity, fixed-income, commodity, currency, and interest rate markets.
FAQs – Why Zillow iBuying Failed
What was Zillow’s iBuying program and how did it fail?
Zillow’s iBuying program, officially known as Zillow Offers, was an initiative by Zillow to buy and sell homes directly.
The idea was to use an AI-based algorithm to identify undervalued homes, purchase them, and then sell them for a profit.
However, this program ultimately failed due to several reasons.
Zillow’s AI system was trained on a massive amount of housing data, but this data was short-term and backward-looking.
It meant that the AI could accurately predict some trends and seasonality, but it missed the broader macroeconomic cycle that is vital in real estate markets and didn’t understand specific nuances of the properties in its database.
Furthermore, the property market is adversarial in nature.
When Zillow’s AI bought a property, it ended up overpaying on average because it didn’t account for hidden factors that other market participants knew about (i.e., “hidden” to an Automated Valuation Model).
These could be anything from neighborhood development plans to issues with the house that aren’t apparent from the data Zillow had access to.
This lack of knowledge resulted in the AI overvaluing properties and Zillow making losses.
Why couldn’t Zillow’s AI model account for the broader macroeconomic cycle?
Zillow’s AI model was fundamentally limited because it was largely statistical and lacked a deeper understanding of what it was doing.
It was capable of analyzing past trends and predicting future patterns based on those trends.
However, the model didn’t have a mechanism to understand some of the broader cause-effect linkages that underpin the real estate market.
The broader macroeconomic cycle, which includes factors like inflation, interest rates, and economic growth, is critical to property prices.
Zillow’s model, being backward-looking and short-term, was not equipped to factor these into its decision-making process, leading to costly errors in valuation.
Why was Zillow’s AI system unable to understand the local factors that influence property prices?
Zillow’s AI system was “naive” in the sense that it could only know and analyze the data it was given.
This data was largely generic and didn’t include the more nuanced factors that often play a significant role in property valuation.
Market participants with local knowledge often know about these factors and can use them to their advantage.
This information could be anything from knowledge about a neighborhood’s future development plans to a property’s hidden defects.
When Zillow won an auction, it often won for a reason.
The adversarial nature of the market meant that these other participants were often able to outmaneuver any automated bot they might be competing against, leading to overvalued property purchases and subsequent financial losses for Zillow.
Is it possible for an AI system to succeed in buying and selling homes?
While Zillow’s iBuying program failed, it doesn’t mean that an AI approach can’t succeed in buying and selling homes.
The important aspect is to use AI appropriately and understand its limitations.
A successful AI system would need to incorporate a deeper understanding of the cause-effect linkages in the real estate market.
This could mean using more advanced AI techniques, such as causal inference, to help understand the broader macroeconomic cycle and local market factors.
Such a system would also need to incorporate local knowledge into its decision-making process, which could involve partnerships with local experts or more advanced data collection and analysis.
It’s a more complex task but not an impossible one.
However, any organization attempting such a task needs to be aware of the complexities and potential pitfalls involved in using AI in this way.
The failure of Zillow’s iBuying program highlights the potential risks of misapplying AI, even when there’s an abundance of data.
And for those who simply use Zillow to check their home’s Zestimate, also be wary of blindly trusting it (a relatively naive, regression-based output). There’s a lot of information that it simply doesn’t know about.
Conclusion
While Zillow’s iBuying program aimed to revolutionize real estate investing using AI, the venture ultimately failed due to a fundamental misunderstanding of the complexities of the industry.
The absence of a macroeconomic perspective and a reasoning engine to understand specific property issues highlighted the limitations of a purely data-driven approach.
The experience provides a critical lesson for other firms in other financial fields and beyond – the successful application of AI requires a thorough understanding of the industry, the macroeconomic environment, and the specific nuances of the phenomenon being studied.